Paper:
Enhanced Naive Agent in Angry Birds AI Competition via Exploitation-Oriented Learning
Kazuteru Miyazaki

National Institution for Academic Degrees and Quality Enhancement of Higher Education
1-29-1 Gakuennishimachi, Kodaira, Tokyo 185-8587, Japan
The Angry Birds AI Competition engages artificial intelligence agents in a contest based on the game Angry Birds. This tournament has been conducted annually since 2012, with participants competing for high scores. The organizers of this competition provide a basic agent, termed “Naive Agent,” as a baseline indicator. This study enhanced the Naive Agent by integrating a profit-sharing approach known as exploitation-oriented learning, which is a type of experience-enhanced learning. The effectiveness of this method was substantiated through numerical experiments. Additionally, this study explored the use of level selection learning within a multi-agent environment and validated the utility of the rationality theorem concerning the indirect rewards in this environment.
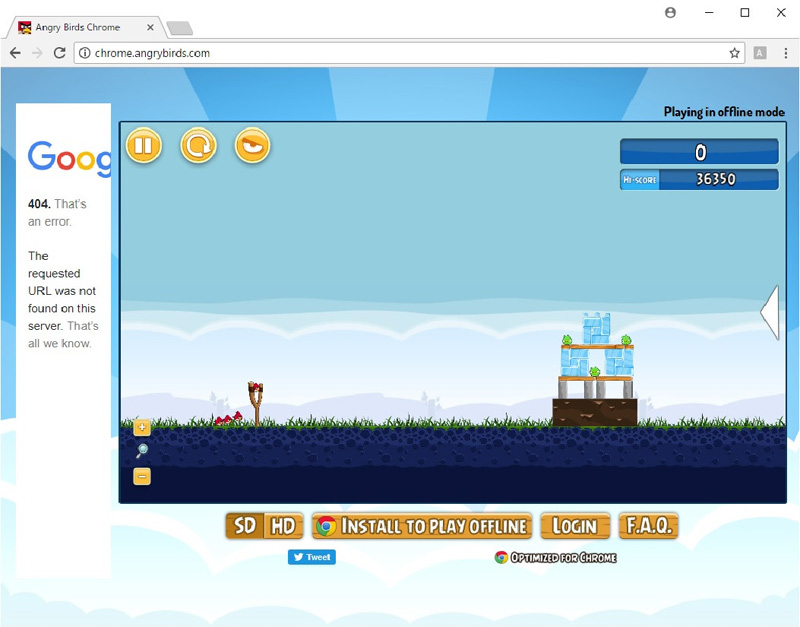
Screenshot of the Angry Birds game
- [1] T. Liu, J. Renz, P. Zhang, and M. Stephenson, “Using Restart Heuristics to Improve Agent Performance in Angry Birds,” arXiv:1905.12877, 2019. https://doi.org/10.48550/arXiv.1905.12877
- [2] J. Renz, “AIBIRDS: The Angry Birds Artificial Intelligence Competition,” Proc. of the AAAI Conf. on Artificial Intelligence, Vol.29, No.1, 2015. https://doi.org/10.1609/aaai.v29i1.9347
- [3] M. Stephenson and J. Renz, “Deceptive Angry Birds: Towards Smarter Game-Playing Agents,” Proc. of the 13th Int. Conf. on the Foundations of Digital Games, Article No.13, 2018. https://doi.org/10.1145/3235765.3235775
- [4] M. Stephenson, J. Renz, X. Ge, and P. Zhang, “The 2017 AIBIRDS Competition,” arXiv:1803.05156, 2018. https://doi.org/10.48550/arXiv.1803.05156
- [5] M. Stephenson et al., “The 2017 AIBIRDS Level Generation Competition,” IEEE Trans. on Games, Vol.11, Issue 3, pp. 275-284, 2018. https://doi.org/10.1109/TG.2018.2854896
- [6] K. Miyazaki, M. Yamamura, and S. Kobayashi, “A Theory of Profit Sharing in Reinforcement Learning,” J. of the Japanese Society for Artificial Intelligence, Vol.9, Issue 4, pp. 580-587, 1994 (in Japanese). https://doi.org/10.11517/jjsai.9.4_580
- [7] K. Miyazaki, “Exploitation-Oriented Learning XoL: A New Approach to Machine Learning Based on Trial-and-Error Searches,” S.-H. Chen, Y. Kambayashi, and H. Sato (Eds.), “Multi-Agent Applications with Evolutionary Computational and Biologically Inspired Technologies: Intelligent Techniques for Ubiquity and Optimization,” IGI Globel, pp. 267-293, 2010. https://doi.org/10.4018/978-1-60566-898-7.ch015
- [8] K. Miyazaki and S. Kobayashi, “Exploitation-Oriented Learning PS-r#,” J. Adv. Comput. Intell. Intell. Inform., Vol.13, No.6, pp. 624-630, 2009. https://doi.org/10.20965/jaciii.2009.p0624
- [9] K. Miyazaki, “Challenge to the Angry Birds AI Competition Through the Exploitation-Oriented Learning Method,” SSI 2019, Poster No.SS09-01, 2019 (in Japanese).
- [10] D. Ha and J. Schmidhuber, “Recurrent World Models Facilitate Policy Evolution,” Proc. of the 32nd Conf. on Neural Information Processing Systems, pp. 2450-2462, 2018.
- [11] M. Polceanu and C. Buche, “Towards A Theory-of-Mind-Inspired Generic Decision-Making Framework,” Int. Joint Conf. on Artificial Intelligence (IJCAI) 2013 Symp. on AI in Angry Birds, 2013. https://doi.org/10.48550/arXiv.1405.5048
- [12] T. Eiter et al., “A Model Building Framework for Answer Set Programming with External Computations,” Theory and Practice of Logic Programming, Vol.16, Issue 4, pp. 418-464, 2016. https://doi.org/10.1017/S1471068415000113
- [13] V. Mnih et al., “Playing Atari with Deep Reinforcement Learning,” arXiv:1312.5602, 2013. https://doi.org/10.48550/arXiv.1312.5602
- [14] V. Mnih et al., “Human-level control through deep reinforcement learning,” Nature, Vol.518, pp. 529-533, 2015. https://doi.org/10.1038/nature14236
- [15] H. van Hasselt, A. Guez, and D. Silver, “Deep Reinforcement Learning with Double Q-Learning,” arXiv:1509.06461v3, 2015. https://doi.org/10.48550/arXiv.1509.06461
- [16] Z. Wang et al., “Dueling Network Architectures for Deep Reinforcement Learning,” arXiv:1511.06581v3, 2015. https://doi.org/10.48550/arXiv.1511.06581
- [17] D. Silver et al., “Mastering the game of Go with deep neural networks and tree search,” Nature, Vol.529, pp. 484-489, 2016. https://doi.org/10.1038/nature16961
- [18] R. S. Sutton and A. G. Barto, “Reinforcement Learning: An Introduction,” IEEE Trans. on Neural Networks, Vol.9, Issue 5, 1998. https://doi.org/10.1109/TNN.1998.712192
- [19] N. Kodama, T. Harada, and K. Miyazaki, “A Proposal of a Deep Reinforcement Learning Algorithm Using Non-Bootstrap Method,” The 46th Symp. on Intelligent System, 2019 (in Japanese).
- [20] K. Miyazaki, “Exploitation-Oriented Learning with Deep Learning – Introducing Profit Sharing to a Deep Q-Network –,” J. Adv. Comput. Intell. Intell. Inform., Vol.21, No.5, pp. 849-855, 2017. https://doi.org/10.20965/jaciii.2017.p0849
- [21] K. Miyazaki, S. Arai, and S. Kobayashi, “A Theory of Profit Sharing in Multi-Agent Reinforcement Learning,” J. of Japanese Society for Artificial Intelligence, Vol.14, No.6, pp. 1156-1164, 1999 (in Japanese). https://doi.org/10.11517/jjsai.14.6_1156
- [22] K. Miyazaki and S. Kobayashi, “Rationality of Reward Sharing in Multi-Agent Reinforcement Learning,” New Generation Computing, Vol.19, No.2, pp. 157-172, 2001. https://doi.org/10.1007/BF03037252
- [23] M.-J. Kim and K.-J. Kim, “Opponent Modeling Based on Action Table for MCTS-Based Fighting Game AI,” Proc. of 2017 IEEE Conf. on Computational Intelligence and Games (CIG), pp. 178-180, 2017. https://doi.org/10.1109/CIG.2017.8080432
- [24] A. Nakagawa, T. Shibazaki, S. Osaka, and R. Thawonmas, “Adjustment of Game Difficulty and Improvement of Action Variety in Fighting Action Games Using Neural Networks,” The J. of Game Amusement Society, Vol.3, No.1, pp. 35-40, 2009 (in Japanese).
- [25] R. Thawonmas and S. Osaka, “A Method for Online Adaptation of Computer-Game AI Rulebase,” Proc. of the 2006 ACM SIGCHI Int. Conf. on Advances in Computer Entertainment Technology, 2006. https://doi.org/10.1145/1178823.1178843
- [26] S. Yoon and K.-J. Kim, “Deep Q Networks for Visual Fighting Game AI,” Proc. of 2017 IEEE Conf. on Computational Intelligence and Games (CIG), pp. 306-308, 2017. https://doi.org/10.1109/CIG.2017.8080451
This article is published under a Creative Commons Attribution-NoDerivatives 4.0 Internationa License.