Paper:
Sensorimotor Activities and Their Functional Connectivity Elicited by Robot-Assisted Passive Movements of Lower Limbs
Takeshi Sakurada*, Ayaka Horiuchi**, and Takashi Komeda**
*Faculty of Science and Technology, Seikei University
3-3-1 Kichijoji-kitamachi, Musashino-shi, Tokyo 180-8633, Japan
**Graduate school of Systems Engineering and Science, Shibaura Institute of Technology
307 Fukasaku, Minuma, Saitama, Saitama 330-8570, Japan
Robot-assisted body movements are a useful approach for the rehabilitation of motor dysfunction. Various robots based on end-effector or exoskeleton type have been proposed. However, the effect of these robots on brain activity during assistive lower limb movements remains unclear. In this study, we evaluated brain activity results among robot-assisted passive movements, voluntary active movements, and kinesthetic motor imagery. We measured and compared the brain activities of 21 young, healthy individuals during three experimental conditions associated with lower limb movements (active, passive, and imagery conditions) using functional near-infrared spectroscopy (fNIRS). Our results showed that although different brain areas with significant activity were observed among the conditions, the temporal patterns of the activity in each recording channel and the spatial patterns of functional connectivity showed high similarity between robot-assisted passive movements and voluntary active movements. Conversely, the robot-assisted passive movements did not show any similarity to motor imagery. Overall, these findings suggest that the robotic assistive approach is useful for activating not only afferent processes associated with sensory feedback processing but also motor control-related efferent processes.
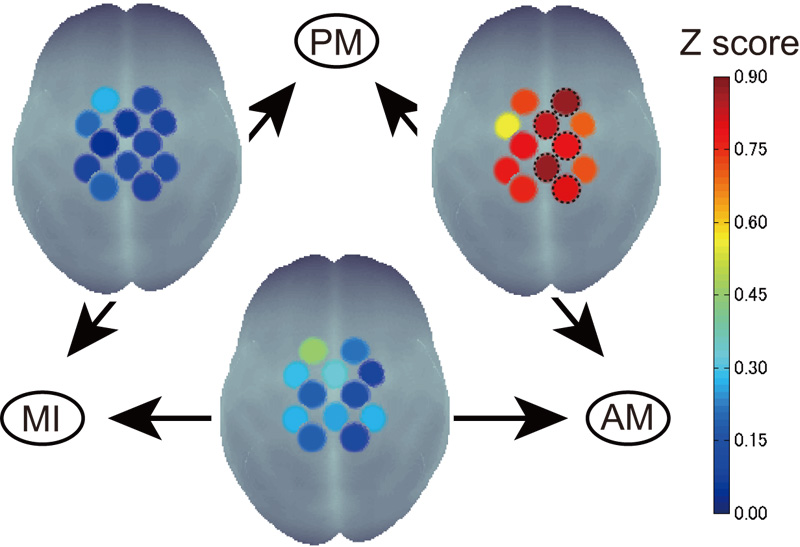
Sensorimotor activity pattern similarity
- [1] W. Meng, Q. Liu, Z. Zhou, Q. Ai, B. Sheng, and S. S. Xie, “Recent development of mechanisms and control strategies for robot-assisted lower limb rehabilitation,” Mechatronics, Vol.31, pp. 132-145, 2015.
- [2] H. S. Lo and S. Q. Xie, “Exoskeleton robots for upper-limb rehabilitation: State of the art and future prospects,” Med. Eng. Phys., Vol.34, No.3, pp. 261-268, 2012.
- [3] R. S. Calabrò, A. Naro, M. Russo, A. Leo, T. Balletta, I. Saccá, R. De Luca, and P. Bramanti, “Do post-stroke patients benefit from robotic verticalization? A pilot-study focusing on a novel neurophysiological approach,” Restor. Neurol. Neurosci., Vol.33, No.5, pp. 671-681, 2015.
- [4] F. Baronchelli, C. Zucchella, M. Serrao, D. Intiso, and M. Bartolo, “The effect of robotic assisted gait training with Lokomat on balance control after sstroke: systematic review and meta-analysis,” Front. Neurol., Vol.12, 661815, 2021.
- [5] T. Ueba, O. Hamada, T. Ogata, T. Inoue, E. Shiota, and Y. Sankai, “Feasibility and safety of acute phase rehabilitation after stroke using the hybrid assistive limb robot suit,” Neurol. Med. Chir. (Tokyo), Vol.53, No.5, pp. 287-290, 2013.
- [6] J. Li, N. Thakor, and A. Bezerianos, “Unilateral exoskeleton imposes significantly different hemispherical effect in parietooccipital region, but not in other regions,” Sci. Rep., Vol.8, No.1, 13470, 2018.
- [7] F. Molteni, E. Formaggio, A. Bosco, E. Guanziroli, F. Piccione, S. Masiero, and A. Del Felice, “Brain connectivity modulation after exoskeleton-assisted gait in chronic hemiplegic stroke survivors: a pilot study,” Am. J. Phys. Med. Rehabil., Vol.99, No.8, pp. 694-700, 2020.
- [8] R. Dickstein, A. Dunsky, and E. Marcovitz, “Motor imagery for gait rehabilitation in post-stroke hemiparesis,” Phys. Ther., Vol.84, No.12, pp. 1167-1177, 2004.
- [9] M. Jeannerod, “Mental imagery in the motor context,” Neuropsychologia, Vol.33, No.11, pp. 1419-1432, 1995.
- [10] A. Guillot, C. Collet, V. A. Nguyen, F. Malouin, C. Richards, and J. Doyon, “Brain activity during visual versus kinesthetic imagery: an fMRI study,” Hum. Brain. Mapp., Vol.30, No.7, pp. 2157-2172, 2009.
- [11] S. Pittaccio, F. Zappasodi, S. Viscuso, F. Mastrolilli, M. Ercolani, F. Passarelli, F. Molteni, S. Besseghini, P. M. Rossini, and F. Tecchio, “Primary sensory and motor cortex activities during voluntary and passive ankle mobilization by the SHADE orthosis,” Hum. Brain. Mapp., Vol.32, No.1, pp. 60-70, 2011.
- [12] S. Pittaccio, F. Zappasodi, G. Tamburro, S. Viscuso, L. Marzetti, L. Garavaglia, F. Tecchio, and V. Pizzella, “Passive ankle dorsiflexion by an automated device and the reactivity of the motor cortical network,” 35th Annual Int. Conf. of the IEEE Engineering in Medicine and Biology Society (EMBC), pp. 6353-6356, 2013.
- [13] S. Qiu, W. Yi, J. Xu, H. Qi, J. Du, C. Wang, F. He, and D. Ming, “Event-related beta EEG changes during active, passive movement and functional electrical stimulation of the lower limb,” IEEE Trans. Neural. Syst. Rehabil. Eng., Vol.24, No.2, pp. 283-290, 2016.
- [14] P. Caruso, M. Ridolfi, G. Furlanis, M. Ajčević, M. Semenic, R. Moretti, M. Naccarato, and P. Manganotti, “Cerebral hemodynamic changes during motor imagery and passive robot-assisted movement of the lower limbs,” J. Neurol. Sci., Vol.405, 116427, 2019.
- [15] E. Formaggio, S. Masiero, A. Bosco, F. Izzi, F. Piccione, and A. Del Felice, “Quantitative EEG evaluation during robot-assisted foot movement,” IEEE Trans. Neural. Syst. Rehabil. Eng., Vol.25, No.9, pp. 1633-1640, 2017.
- [16] C. Stippich, H. Ochmann, and K. Sartor, “Somatotopic mapping of the human primary sensorimotor cortex during motor imagery and motor execution by functional magnetic resonance imaging,” Neurosci. Lett., Vol.331, No.1, pp. 50-54, 2002.
- [17] G. R. Müller-Putz, D. Zimmermann, B. Graimann, K. Nestinger, G. Korisek, and G. Pfurtscheller, “Event-related beta EEG-changes during passive and attempted foot movements in paraplegic patients,” Brain. Res., Vol.1137, No.1, pp. 84-91, 2007.
- [18] D. Tsuzuki, V. Jurcak, A. K. Singh, M. Okamoto, E. Watanabe, and I. Dan, “Virtual spatial registration of stand-alone fNIRS data to MNI space,” Neuroimage, Vol.34, No.4, pp. 1506-1518, 2007.
- [19] D. Tsuzuki and I. Dan, “Spatial registration for functional near-infrared spectroscopy: from channel position on the scalp to cortical location in individual and group analyses,” Neuroimage, Vol.85, No.1, pp. 92-103, 2014.
- [20] V. Toronov, A. Webb, J. H. Choi, M. Wolf, A. Michalos, E. Gratton, and D. Hueber, “Investigation of human brain hemodynamics by simultaneous near-infrared spectroscopy and functional magnetic resonance imaging,” Med. Phys., Vol.28, No.4, pp. 521-527, 2001.
- [21] G. Strangman, J. P. Culver, J. H. Thompson, and D. A. Boas, “A quantitative comparison of simultaneous BOLD fMRI and NIRS recordings during functional brain activation,” Neuroimage, Vol.17, No.2, pp. 719-731, 2002.
- [22] G. Matsuda and K. Hiraki, “Sustained decrease in oxygenated hemoglobin during video games in the dorsal prefrontal cortex: A NIRS study of children,” Neuroimage, Vol.29, No.3, pp. 706-711, 2006.
- [23] M. L. Schroeter, M. M. Bücheler, K. Müller, K. Uludağ, H. Obrig, G. Lohmann, M. Tittgemeyer, A. Villringer, and D. Y. von Cramon, “Towards a standard analysis for functional near-infrared imaging,” Neuroimage, Vol.21, No.1, pp. 283-290, 2004.
- [24] F. Homae, H. Watanabe, T. Nakano, and G. Taga, “Large-scale brain networks underlying language acquisition in early infancy,” Front. Psychol., Vol.2, p. 93, 2011.
- [25] M. Imai, H. Watanabe, K. Yasui, Y. Kimura, Y. Shitara, S. Tsuchida, N. Takahashi, and G. Taga, “Functional connectivity of the cortex of term and preterm infants and infants with Down’s syndrome,” Neuroimage, Vol.85, No.1, pp. 272-278, 2014.
- [26] S. Hétu, M. Grégoire, A. Saimpont, M. P. Coll, F. Eugène, P. E. Michon, and P. L. Jackson, “The neural network of motor imagery: an ALE meta-analysis,” Neurosci. Biobehav. Rev., Vol.37, No.5, pp. 930-949, 2013.
- [27] S. H. Scott, “Optimal feedback control and the neural basis of volitional motor control,” Nat. Rev. Neurosci., Vol.5, No.7, pp. 532-546, 2004.
- [28] O. Ciccarelli, A. T. Toosy, J. F. Marsden, C. M. Wheeler-Kingshott, C. Sahyoun, P. M. Matthews, D. H. Miller, and A. J. Thompson, “Identifying brain regions for integrative sensorimotor processing with ankle movements,” Exp. Brain. Res., Vol.166, No.1, pp. 31-42, 2005.
- [29] A. Berger, F. Horst, F. Steinberg, F. Thomas, C. Müller-Eising, W. I. Schöllhorn, and M. Doppelmayr, “Increased gait variability during robot-assisted walking is accompanied by increased sensorimotor brain activity in healthy people,” J. Neuroeng. Rehabil., Vol.16, No.1, 161, 2019.
- [30] M. Mihara, I. Miyai, N. Hattori, M. Hatakenaka, H. Yagura, T. Kawano, M. Okibayashi, N. Danjo, A. Ishikawa, Y. Inoue, and K. Kubota, “Neurofeedback using real-time near-infrared spectroscopy enhances motor imagery related cortical activation,” PLoS One, Vol.7, No.3, e32234, 2012.
- [31] S. Silva, L. R. D. M. Borges, L. Santiago, L. Lucena, A. R. Lindquist, and T. Ribeiro, “Motor imagery for gait rehabilitation after stroke,” Cochrane Database Syst. Rev., Vol.9, No.9, CD013019, 2020.
- [32] W.-P. Teo and E. Chew, “Is motor-imagery brain-computer interface feasible in stroke rehabilitation?,” PM&R, Vol.6, No.8, pp. 723-728, 2014.
- [33] T. Sakurada, M. Hirai, and E. Watanabe, “Optimization of a motor learning attention-directing strategy based on an individual’s motor imagery ability,” Exp. Brain Res., Vol.234, No.1, pp. 301-311, 2016.
- [34] T. Sakurada, T. Nakajima, M. Morita, M. Hirai, and E. Watanabe, “Improved motor performance in patients with acute stroke using the optimal individual attentional strategy,” Sci. Rep., Vol.7, 40592, 2017.
- [35] T. Funane, H. Atsumori, T. Katura, A. Obata, H. Sato, Y. Tanikawa, E. Okada, and M. Kiguchi, “Quantitative evaluation of deep and shallow tissue layers’ contribution to fNIRS signal using multi-distance optodes and independent component analysis,” Neuroimage, Vol.85, pp. 150-165, 2014.
This article is published under a Creative Commons Attribution-NoDerivatives 4.0 Internationa License.