Paper:
VIDVIP: Dataset for Object Detection During Sidewalk Travel
Tetsuaki Baba
Tokyo Metropolitan University
6-6 Asahigaoka, Hino, Tokyo 191-0065, Japan
In this paper, we report on the “VIsual Dataset for Visually Impaired Persons” (VIDVIP), a dataset for obstacle detection during sidewalk travel. In recent years, there have been many reports on assistive technologies using deep learning and computer vision technologies; nevertheless, developers cannot implement the corresponding applications without datasets. Although a number of open-source datasets have been released by research institutes and companies, large-scale datasets are not as abundant in the field of disability support, owing to their high development costs. Therefore, we began developing a dataset for outdoor mobility support for the visually impaired in April 2018. As of May 1, 2021, we have annotated 538,747 instances for 32,036 images in 39 classes of labels. We have implemented and tested navigation systems and other applications that utilize our dataset. In this study, we first compare our dataset with other general-purpose datasets, and show that our dataset has properties similar to those of datasets for automated driving. As a result of the discussion on the characteristics of the dataset, it is shown that the nature of the image shooting location, rather than the regional characteristics, tends to affect the annotation ratio. Accordingly, it is possible to examine the type of location based on the nature of the shooting location, and to infer the maintenance statuses of traffic facilities (such as Braille blocks) from the annotation ratio.
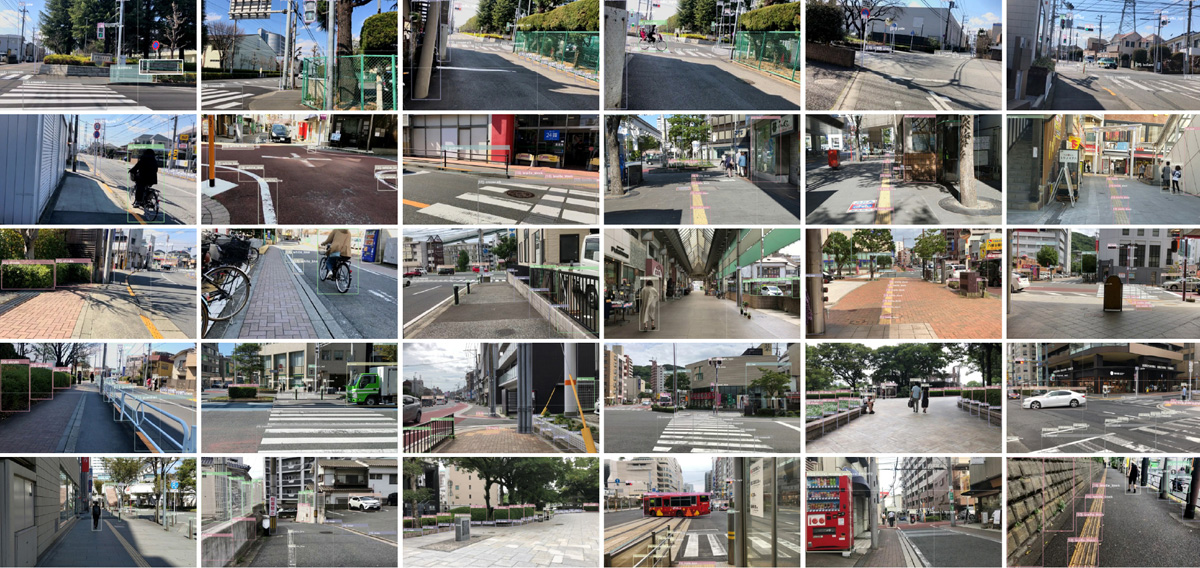
Examples of images actually annotated
- [1] A. Kuznetsova, H. Rom, N. Alldrin, J. Uijlings, I. Krasin, J. Pont-Tuset, S. Kamali, S. Popov, M. Malloci, T. Duerig, and V. Ferrari, “The open images dataset v4: Unified image classification, object detection, and visual relationship detection at scale,” Int. J. of Computer Vision, Vol.128, pp. 1956-1981, 2020.
- [2] T.-Y. Lin, M. Maire, S. J. Belongie, L. D. Bourdev, R. B. Girshick, J. Hays, P. Perona, D. Ramanan, P. Dollár, and C. L. Zitnick, “Microsoft COCO: common objects in context,” CoRR, abs/1405.0312, 2014.
- [3] N. Thakurdesai, A. Tripathi, D. Butani, and S. Sankhe, “Vision: A deep learning approach to provide walking assistance to the visually impaired,” CoRR, abs/1911.08739, 2019.
- [4] A. Geiger, P. Lenz, C. Stiller, and R. Urtasun, “Vision meets robotics: The kitti dataset,” Int. J. of Robotics Research (IJRR), 2013.
- [5] T. Baba, H. Watanave, and T. Kamae, “Design and prototyping for an outdoor activity support system for the visually impaired using deep learning for object detection,” SIG Technical Reports, IPSJ, Vol.32018-AAC-7, No.8, Aug. 2018 (in Japanese).
- [6] K. Ishisone, T. Baba, H. Watanave, and T. Kamae, “Basic study and prototyping of an object detection dataset for outdoor mobility support for the visually impaired,” SIG Technical Reports, IPSJ, Vol.2018-AAC-7, No.9, Aug. 2018 (in Japanese).
- [7] K. Kuzume, H. Masuda, and Y. Murakami, “Automatic identification of braille blocks by neural network using multi-channel pressure sensor array,” 2020 The 3rd Int. Conf. on Computational Intelligence and Intelligent Systems (CIIS 2020), pp. 93-99, New York, NY, USA, 2020.
- [8] S. Asad, B. Mooney, I. Ahmad, M. Huber, and A. Clark, “Object detection and sensory feedback techniques in building smart cane for the visually impaired: An overview,” Proc. of the 13th ACM Int. Conf. on PErvasive Technologies Related to Assistive Environments (PETRA ’20), New York, NY, USA, 2020.
- [9] T. Baba, K. Ishisone, K. Watanabe, H. Watanave, T. Kamae, K. Suematsu, S. Takata, and Y. Kuga, “Developing a localized object detection dataset supporting sidewalk use for visually impaired persons in japan,” Trans. of the Virtual Reality Society of Japan, Vol.25, No.3, pp. 185-195, 2020.
- [10] T. Baba, “Design for the visually impaired when traveling outdoors using omnidirectional imagery and image recognition,” Impact, Vol.2020, No.7, pp. 34-36, 2020.
- [11] P. Sun, H. Kretzschmar, X. Dotiwalla, A. Chouard, V. Patnaik, P. Tsui, J. Guo, Y. Zhou, Y. Chai, B. Caine, V. Vasudevan, W. Han, J. Ngiam, H. Zhao, A. Timofeev, S. Ettinger, M. Krivokon, A. Gao, A. Joshi, Y. Zhang, J. Shlens, Z. Chen, and D. Anguelov, “Scalability in perception for autonomous driving: Waymo open dataset,” Proc. of the IEEE/CVF Conf. on Computer Vision and Pattern Recognition (CVPR), Jun. 2020.
- [12] L. Ding, J. Terwilliger, R. Sherony, B. Reimer, and L. Fridman, “Mit driveseg (manual) dataset,” IEEE Dataport, doi: 10.21227/mmke-dv03, 2020.
- [13] J. Geyer, Y. Kassahun, M. Mahmudi, X. Ricou, R. Durgesh, A. S. Chung, L. Hauswald, V. H. Pham, M. Mühlegg, S. Dorn, T. Fernandez, M. Jänicke, S. Mirashi, C. Savani, M. Sturm, O. Vorobiov, M. Oelker, S. Garreis, and P. Schuberth, “A2D2: Audi Autonomous Driving Dataset,” arXiv:1911.08739, 2020.
- [14] B. Kaur and J. Bhattacharya, “A scene perception system for visually impaired based on object detection and classification using multi-modal DCNN,” CoRR, abs/1805.08798, 2018.
- [15] A. Palla, D. Mulfari, and L. Fanucci, “Using tensorflow to design assistive technologies for people with visual impairments,” IADIS Int. Conf. Big Data Analytics, Data Mining and Computational Intelligence 2017 (part of MCCSIS 2017), pp. 110-116, 2017.
- [16] S. Chaudhry and R. Chandra, “Design of a Mobile Face Recognition System for Visually Impaired Persons,” arXiv:1502.00756, Feb. 2015.
- [17] Information Technology Promotion Agency, “AI Hakusho2020,” 2020 (in Japanese).
- [18] D. Azuma et al., “Development of the new welfare service “partner mobility” using ai interactive automated drive system,” Bulletin of Kurume Institute of Technology, Vol.43, pp. 2-12, Mar. 2021 (in Japanese).
- [19] T. Okita, H. Kojima, S. Ooi, and M. Sano, “A study on navigation system for visually impaired person based on egocentric vision using deep learning,” Technical Report 10, Graduate School of Information Science and Technology, Osaka Institute of Technology; College of Information Science and Engineering, Ritsumeikan University; Faculty of Information Science and Technology, Osaka Institute of Technology, Mar. 2019 (in Japanese).
- [20] K. Li, G. Wan, G. Cheng, L. Meng, and J. Han, “Object detection in optical remote sensing images: A survey and a new benchmark,” ISPRS J. of Photogrammetry and Remote Sensing, Vol.159, pp. 296-307, Jan. 2020.
- [21] M. Everingham, L. Van Gool, C. K. I. Williams, J. Winn, and A. Zisserman, “The pascal visual object classes (voc) challenge,” Int. J. of Computer Vision, Vol.88, No.2, pp. 303-338, Jun. 2010.
- [22] J. Redmon and A. Farhadi, “Yolov3: An incremental improvement,” arXiv:1804.02767, 2018.
- [23] K. Watanabe, T. Baba, K. Tamura, H. Watanave, and T. Kamae, “Basic study of support for visually impaired people touring monuments in hiroshima peace memorial park,” SIG Technical Reports, IPSJ, Vol.2018-AAC-8, No.2, Nov. 2018 (in Japanese).
This article is published under a Creative Commons Attribution-NoDerivatives 4.0 Internationa License.