Paper:
Tsukuba Challenge 2017 Dynamic Object Tracks Dataset for Pedestrian Behavior Analysis
Jacob Lambert*, Leslie Liang**, Luis Yoichi Morales**, Naoki Akai**, Alexander Carballo**, Eijiro Takeuchi*, Patiphon Narksri**, Shunya Seiya*, and Kazuya Takeda*
*Graduate School of Informatics, Nagoya University
Furo-cho, Chikusa-ku, Nagoya 464-8603, Japan
**Institute of Innovation for Future Society, Nagoya University
Furo-cho, Chikusa-ku, Nagoya 464-8601, Japan
Navigation in social environments, in the absence of traffic rules, is the difficult task at the core of the annual Tsukuba Challenge. In this context, a better understanding of the soft rules that influence social dynamics is key to improve robot navigation. Prior research attempts to model social behavior through microscopic interactions, but the resulting emergent behavior depends heavily on the initial conditions, in particular the macroscopic setting. As such, data-driven studies of pedestrian behavior in a fixed environment may provide key insight into this macroscopic aspect, but appropriate data is scarcely available. To support this stream of research, we release an open-source dataset of dynamic object trajectories localized in a map of 2017 Tsukuba Challenge environment. A data collection platform equipped with lidar, camera, IMU, and odometry repeatedly navigated the challenge’s course, recording observations of passersby. Using a background map, we localized ourselves in the environment, removed the static background from the point cloud data, clustered the remaining points into dynamic objects and tracked their movements over time. In this work, we present the Tsukuba Challenge Dynamic Object Tracks dataset, which features nearly 10,000 trajectories of pedestrians, cyclists, and other dynamic agents, in particular autonomous robots. We provide a 3D map of the environment used as global frame for all trajectories. For each trajectory, we provide at regular time intervals an estimated position, velocity, heading, and rotational velocity, as well as bounding boxes for the objects and segmented lidar point clouds. As additional contribution, we provide a discussion which focuses on some discernible macroscopic patterns in the data.
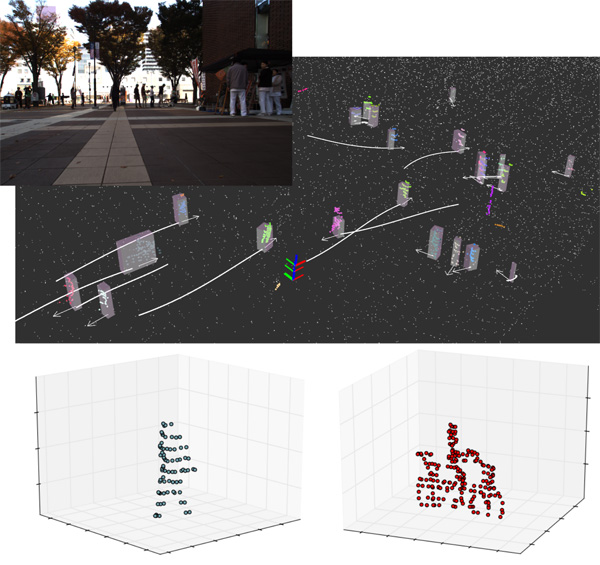
Dynamic object tracks open dataset
- [1] S. Yuta, H. Hashimoto, and H. Tashiro, “Tsukuba challenge: Real world robot challenge (RWRC): Toward actual autonomous robots in our daily life,” 25th Annual Conf. of the Robotics Society of Japan, p. 3D19, September 2007.
- [2] D. Helbing and P. Molnár, “Social force model for pedestrian dynamics,” Physical Review E, Vol.51, No.5, pp. 4283-4286, 1995.
- [3] X. Wang, K. T. Ma, G.-W. Ng, and W. E. L. Grimson, “Trajectory analysis and semantic region modeling using a nonparametric bayesian model,” 2008 IEEE Conf. on Computer Vision and Pattern Recognition, pp. 1-8, June 2008.
- [4] S. Yi, H. Li, and X. Wang, “Understanding pedestrian behaviors from stationary crowd groups,” 2015 IEEE Conf. on Computer Vision and Pattern Recognition (CVPR), pp. 3488-3496, June 2015.
- [5] A. Geiger, P. Lenz, C. Stiller, and R. Urtasun, “Vision meets robotics: The KITTI dataset,” Int. J. of Robotics Research (IJRR), Vol.32, No.11, pp. 1231-1237, 2013.
- [6] M. Cordts, M. Omran, S. Ramos, T. Rehfeld, M. Enzweiler, R. Benenson, U. Franke, S. Roth, and B. Schiele, “The cityscapes dataset for semantic urban scene understanding,” Proc. of the IEEE Conf. on Computer Vision and Pattern Recognition (CVPR), 2016.
- [7] N. Schneider and D. M. Gavrila, “Pedestrian path prediction with recursive bayesian filters: A comparative study,” 35th German Conf. on Pattern Recognition (GCPR 2013), pp. 174-183, Springer, September 2013.
- [8] G. J. Brostow, J. Fauqueur, and R. Cipolla, “Semantic object classes in video: A high-definition ground truth database,” Pattern Recognit. Lett., Vol.30, No.2, pp. 88-97, 2009.
- [9] L. Wang, J. Shi, G. Song, and I.-F. Shen, “Object detection combining recognition and segmentation,” 8th Asian Conf. on Computer Vision, pp. 189-199. Springer, November 2007.
- [10] P. Dollár, C. Wojek, B. Schiele, and P. Perona, “Pedestrian detection: an evaluation of the state of the art,” IEEE Trans. Pattern Anal. Mach. Intell., Vol.34, No.4, pp. 743-761, April 2012.
- [11] A. Teichman, J. Levinson, and S. Thrun, “Towards 3D object recognition via classification of arbitrary object tracks,” 2011 IEEE Int. Conf. on Robotics and Automation, p. 1, 2011.
- [12] M. De Deuge, A. Quadros, C. Hung, and B. Douillard, “Unsupervised feature learning for classification of outdoor 3D scans,” Proc. of Australasian Conf. on Robitics and Automation, Vol.2, p. 1, 2013.
- [13] S. Yi, H. Li, and X. Wang, “Pedestrian behavior modeling from stationary crowds with applications to intelligent surveillance,” IEEE Trans. on Image Processing, Vol.25, No.9, pp. 4354-4368, 2016.
- [14] E. Takeuchi and T. Tsubouchi, “A 3-D scan matching using improved 3-D normal distributions transform for mobile robotic mapping,” 2006 IEEE/RSJ Int. Conf. on Intelligent Robots and Systems, pp. 3068-3073, October 2006.
- [15] S. Kato, E. Takeuchi, Y. Ishiguro, Y. Ninomiya, K. Takeda, and T. Hamada, “An open approach to autonomous vehicles,” IEEE Micro, Vol.35, No.6, pp. 60-68, November 2015.
- [16] N. Akai, Y. Morales, T. Yamaguchi, E. Takeuchi, Y. Yoshihara, H. Okuda, T. Suzuki, and Y. Ninomiya, “Autonomous driving based on accurate localization using multilayer lidar and dead reckoning,” 2017 IEEE 20th Int. Conf. on Intelligent Transportation Systems (ITSC), pp. 1147-1152, October 2017.
- [17] A. Hornung, K. M. Wurm, A. Bennewitz, C. Stachniss, and W. Burgard, “OctoMap: An efficient probabilistic 3D mapping framework based on octrees,” Autonomous Robots, Vol.34, No.3, 2013.
- [18] P. Narksri, Y. Morales, N. Akai, and Y. Ninomiya, “3D LiDAR-based real-time ground segmentation for autonomous vehicles,” Poster session presented at The Second Seminar on JSPS Core-to-Core Program: Establishment of Research Hub for Compact Mobility Model in the ASEAN Region, August 2017.
- [19] R. B. Rusu, “Semantic 3D Object Maps for Everyday Manipulation in Human Living Environments,” KI – Künstliche Intelligenz, 2009.
- [20] H. W. Kuhn and B. Yaw, “The hungarian method for the assignment problem,” Naval Res. Logist. Quart, 1955.
- [21] H. Shum, M. Hebert, and K. Ikeuchi, “On 3D shape similarity,” Proc. of IEEE Conf. on Computer Vision and Pattern Recognition, pp. 526-531, 1996.
- [22] C. de Boor, “A Practical Guide to Spline,” Springer, 1978.
- [23] Y. Morales, T. Kanda, and N. Hagita, “Walking together: Side by side walking model for an interacting robot,” J. of Human-Robot Interaction, Vol.3, No.2, pp. 50-73, 2014.
This article is published under a Creative Commons Attribution-NoDerivatives 4.0 Internationa License.