Paper:
Method Evaluation for Short-Term Wind Speed Prediction Considering Multi Regions in Japan
Ikki Tanaka and Hiromitsu Ohmori
School of Integrated Design Engineering, Graduate School of Science and Technology, Keio University
3-14-1 Hiyoshi, Kohoku-ku, Yokohama 223-8522, Japan
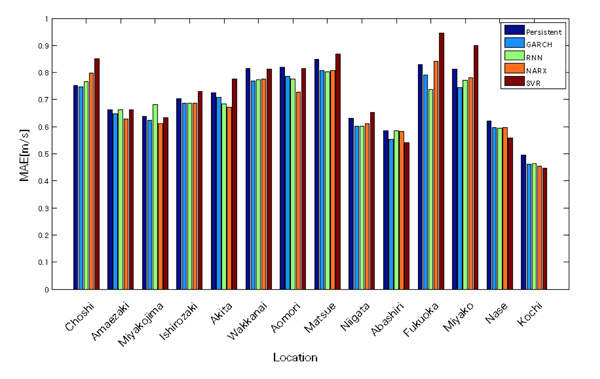
Prediction errors at observation points
- [1] N. Cheggaga, “Improvements in wind speed forecasting using an online learning,” 2014 5th Int. Renewable Energy Congress (IREC), pp. 1-6, 2014.
- [2] D. Sharma and T. T. Lie, “Wind speed forecasting using hybrid ANN-Kalman filter techniques,” 2012 Int. Power and Energy Conf. (IPEC), pp. 644-648, 2012.
- [3] H. Babazadeh et al., “An hour ahead wind speed prediction by Kalman filter,” 2012 IEEE Power Electronics and Machines in Wind Applications (PEMWA), pp. 1-6, 2012.
- [4] B. P. Sangita and M. S. R. Deshmukh, “Use of support vector machine for wind speed prediction,” 2011 Int. Conf. on Power and Energy Systems (ICPS), pp. 1-8, 2011.
- [5] R. G. Kavasseri and K. Seetharaman, “Day-ahead wind speed forecasting using f-ARIMA models,” Renewable Energy, Vol.34, No.5, pp. 1388-1393, 2009.
- [6] Y.-K. Wu and J.-S. Hong, “A literature review of wind forecasting technology in the world,” 2007 IEEE Lausanne Power Tech, pp. 504-509, 2007.
- [7] M. Lei et al., “A review on the forecasting of wind speed and generated power,” Renewable and Sustainable Energy Reviews, Vol.13, No.4, pp. 915-920, 2009.
- [8] H. Chen et al., “GARCH in mean type models for wind power forecasting,” 2013 IEEE Power & Energy Society General Meeting (PES), pp. 1-5, 2013.
- [9] H. B. Azad, S. Mekhilef, and V. G. Ganapathy, “Long-term wind speed forecasting and general pattern recognition using neural networks,” IEEE Trans. on Sustainable Energy, Vol.5, No.2, pp. 546-553, 2014.
- [10] R. L. Welch, S. M. Ruffing, and G. K. Venayagamoorthy, “Comparison of feedforward and feedback neural network architectures for short term wind speed prediction,” 2009 Int. Joint Conf. on Neural Networks, pp. 3335-3340, 2009.
- [11] H. Zhonghe, Z. Xiaoxun, and Y. Xiaojing, “Training Method of support vector regression based on multi-dimensional feature and research on forecast model of vibration time series,” 2010 Int. Conf. on Intelligent Computation Technology and Automation, Vol.3, pp. 1087-1090, 2010.
- [12] M. Sugimoto and K. Kurashige, “A Study of Effective Prediction Methods of the State-action Pair for Robot Control using Online SVR,” J. of Robotics and Mechatronics, Vol.27, No.5, pp. 469-479, 2015.
- [13] Y. Wang et al., “Short-term wind speed prediction using support vector regression,” 2010 IEEE Power & Energy Society General Meeting, pp. 1-6, 2010.
- [14] S. Oke et al., “Any-place forecasting method of nationwide time-series wind speed using classified forecast models based on wind conditions,” IEEJ Trans. on Power & Energy, Vol.129, pp. 598-604, 2009.
- [15] J. D. Hamilton, “Time series analysis,” Vol.2, Princeton, Princeton University Press, 1994.
- [16] A. Lojowska et al., “Advantages of ARMA-GARCH wind speed time series modeling,” 2010 IEEE 11th Int. Conf. on Probabilistic Methods Applied to Power Systems, pp. 83-88, 2010.
- [17] V. Vapnik, S. E. Golowich, and A. Smola, “Support vector method for function approximation, regression estimation, and signal processing,” Advances in Neural Information Processing Systems, Vol.9, 1996.
- [18] V. Vapnik, “The nature of statistical learning theory,” Springer Science & Business Media, 2013.
- [19] C. M. Bishop, “Pattern Recognition,” Machine Learning, 2006.
- [20] A. G. Abo-Khalil and D.-C. Lee, “MPPT control of wind generation systems based on estimated wind speed using SVR,” IEEE Trans. on Industrial Electronics, Vol.55, No.3, pp. 1489-1490, 2008.
- [21] S. C. Johnson, “Hierarchical clustering schemes,” Psychometrika, Vol.32, No.3, pp. 241-254, 1967.
This article is published under a Creative Commons Attribution-NoDerivatives 4.0 Internationa License.