Paper:
A Heuristic-Based Model for MMMs-Induced Fuzzy Co-Clustering with Dual Exclusive Partition
Katsuhiro Honda, Yoshiki Hakui, Seiki Ubukata, and Akira Notsu
Osaka Prefecture University
1-1 Gakuen-cho, Nakaku, Sakai, Osaka 599-8531, Japan
MMMs-induced fuzzy co-clustering achieves dual partition of objects and items by estimating two different types of fuzzy memberships. Because memberships of objects and items are usually estimated under different constraints, the conventional models mainly targeted object clusters only, but item memberships were designed for representing intra-cluster typicalities of items, which are independently estimated in each cluster. In order to improve the interpretability of co-clusters, meaningful items should not belong to multiple clusters such that each co-cluster is characterized by different representative items. In previous studies, the item sharing penalty approach has been applied to the MMMs-induced model but the dual exclusive constraints approach has not yet. In this paper, a heuristic-based approach in FCM-type co-clustering is modified for adopting in MMMs-induced fuzzy co-clustering and its characteristics are demonstrated through several comparative experiments.
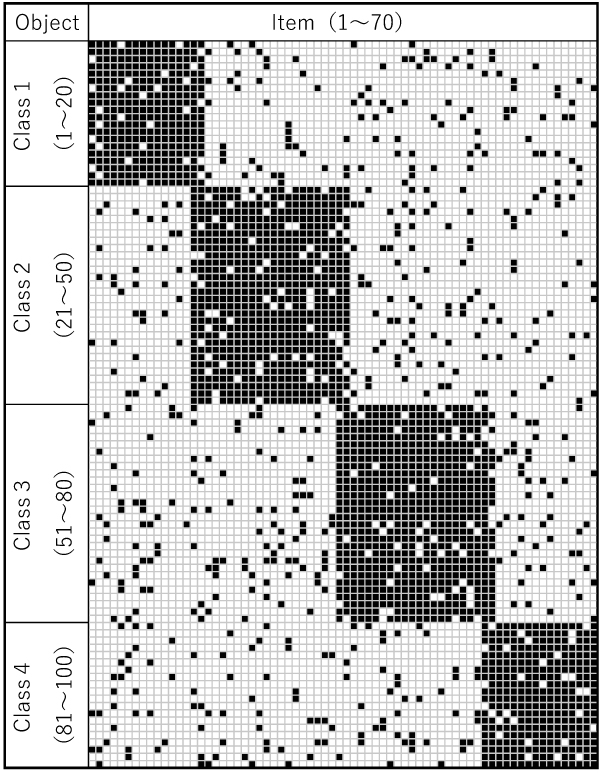
Co-clustering of cooccurrence information
- [1] J. C. Bezdek, “Pattern Recognition with Fuzzy Objective Function Algorithms,” Plenum Press, 1981.
- [2] S. Miyamoto, H. Ichihashi, and K. Honda, “Algorithms for Fuzzy Clustering,” Springer, 2008.
- [3] C.-H. Oh, K. Honda, and H. Ichihashi, “Fuzzy clustering for categorical multivariate data,” Proc. of Joint 9th IFSA World Congress and 20th NAFIPS Int. Conf., Vol.4, pp. 2154-2159, 2001.
- [4] K. Kummamuru, A. Dhawale, and R. Krishnapuram, “Fuzzy co-clustering of documents and keywords,” Proc. of 12th IEEE Int. Conf. on Fuzzy Systems, Vol.2, pp. 772-777, 2003.
- [5] T.-C. T. Chen and K. Honda, “Fuzzy Collaborative Forecasting and Clustering,” Springer, 2019.
- [6] K. Honda, S. Oshio, and A. Notsu, “Fuzzy co-clustering induced by multinomial mixture models,” J. Adv. Comput. Intell. Intell. Inform., Vol.19, No.6, pp. 717-726, 2015.
- [7] L. Rigouste, O. Cappé, and F. Yvon, “Inference and evaluation of the multinomial mixture model for text clustering,” Information Processing & Management, Vol.43, No.5, pp. 1260-1280, 2007.
- [8] K. Honda, C.-H. Oh, Y. Matsumoto, A. Notsu, and H. Ichihashi, “Exclusive partition in FCM-type co-clustering and its application to collaborative filtering,” Int. J. of Computer Science and Network Security, Vol.12, No.12, pp. 52-58, 2012.
- [9] W.-C. Tjhi and L. Chen, “A partitioning based algorithm to fuzzy co-cluster documents and words,” Pattern Recognition Letters, Vol.27, No.3, pp. 151-159, 2006.
- [10] W.-C. Tjhi and L. Chen, “A heuristic-based fuzzy co-clustering algorithm for categorization of high-dimensional data,” Fuzzy Sets and Systems, Vol.159, No.4, pp. 371-389, 2008.
- [11] S. Miyamoto and M. Mukaidono, “Fuzzy c-means as a regularization and maximum entropy approach,” Proc. of the 7th Int. Fuzzy Systems Association World Congress, Vol.2, pp 86-92, 1997.
- [12] H. Ichihashi, K. Miyagishi, and K. Honda, “Fuzzy c-means clustering with regularization by K-L information,” Proc. of 10th IEEE Int. Conf. on Fuzzy Systems, Vol.2, pp. 924-927, 2001.
- [13] K. Honda and H. Ichihashi, “Regularized linear fuzzy clustering and probabilistic PCA mixture models,” IEEE Trans. on Fuzzy Systems, Vol.13, No.4, pp. 508-516, 2005.
- [14] K. Tsuda, M. Minoh, and K. Ikeda, “Extracting straight lines by sequential fuzzy clustering,” Pattern Recognition Letters, Vol.17, No.6, pp. 643-649, 1996.
- [15] K. Honda, T. Nakano, C.-H. Oh, S. Ubukata, and A. Notsu, “Partially exclusive item partition in MMMs-induced fuzzy co-clustering and its effects in collaborative filtering,” J. Adv. Comput. Intell. Intell. Inform., Vol.19, No.6, pp. 810-817, 2015.
- [16] K. Honda, N. Yamamoto, S. Ubukata, and A. Notsu, “Noise rejection in MMMs-induced fuzzy co-clustering,” J. Adv. Comput. Intell. Intell. Inform., Vol.21, No.7, pp. 1144-1151, 2017.
- [17] T. Goshima, K. Honda, S. Ubukata, and A. Notsu, “Deterministic annealing process for pLSA-induced fuzzy co-clustering and cluster splitting characteristics,” Int. J. of Approximate Reasoning, Vol.95, pp. 185-193, 2018.
- [18] T. Hofmann, “Unsupervised learning by probabilistic latent semantic analysis,” Machine Learning, Vol.42, No.1-2, pp. 177-196, 2001.
This article is published under a Creative Commons Attribution-NoDerivatives 4.0 Internationa License.