Letter:
Respiration Differentiating Using Wavelet Transform and Autoencoder
Kazuki Sekimoto and Junji Satake
Fukuoka Institute of Technology
3-30-1 Wajiro-higashi, Higashi-ku, Fukuoka, Fukuoka 811-0295, Japan
This study proposes a novel respiration differentiation method using a wavelet transform and an autoencoder. Depth changes in a person’s chest were acquired from the depth images generated by the Kinect sensor and recorded as waveform data. The extracted waveform data were frequency-analyzed using a wavelet transform for the autoencoder learning. The autoencoder was trained exclusively on normal respiratory data. Respiratory data were identified based on the differences between the autoencoder’s inputs and outputs. To automatically differentiate respiration from other movements, a threshold was set using Hotelling’s theory. The proposed respiration differentiation method was experimentally evaluated to verify its high recognition rates, even for untrained individuals, using cross-validation. Respiration differentiation was also applied to the entire image to confirm that the model could accurately recognize chest movements resulting from respiration.
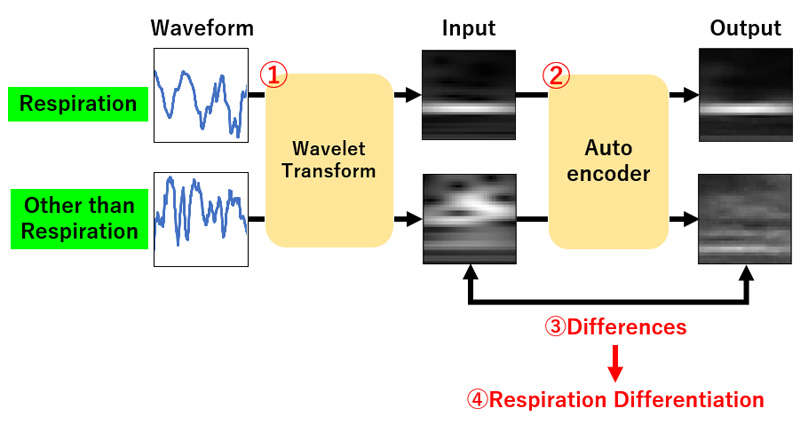
Respiration differentiating
- [1] J. Morimoto et al., “Estimation model for emotions based on pulse,” J. Robot. Mechatron., Vol.35, No.3, pp. 788-798, 2023. https://doi.org/10.20965/jrm.2023.p0788
- [2] K. Matsuo, T. Mukai, and S. Guo, “Unconstrained measurement of heart rate considering harmonics of respiratory signal using flexible tactile sensor sheet,” J. Robot. Mechatron., Vol.33, No.4, pp. 826-832, 2021. https://doi.org/10.20965/jrm.2021.p0826
- [3] H. Aoki et al., “Non-contact respiration measurement using structured light 3-D Sensor,” 2012 Proc. of SICE Annual Conf., pp. 614-618, 2012.
- [4] Q. Li, H. Cao, Y. Li, and Y. Lu, “How do you breathe—A non-contact monitoring method using depth data,” 2017 IEEE 19th Int. Conf. on e-Health Networking, Applications and Services (Healthcom), 2017. https://doi.org/10.1109/HealthCom.2017.8210796
- [5] N. Bernacchia et al., “Non contact measurement of heart and respiration rates based on Kinect™,” 2014 IEEE Int. Symp. on Medical Measurements and Applications (MeMeA), 2014. https://doi.org/10.1109/MeMeA.2014.6860065
- [6] Y. Matsuura et al., “Screening sleep disordered breathing with noncontact measurement in a clinical site,” J. Robot. Mechatron., Vol.29, No.2, pp. 327-337, 2017. https://doi.org/10.20965/jrm.2017.p0327
- [7] F. Deng et al., “Design and implementation of a noncontact sleep monitoring system using infrared cameras and motion sensor,” IEEE Trans. on Instrumentation and Measurement, Vol.67, No.7, pp. 1555-1563, 2018. https://doi.org/10.1109/TIM.2017.2779358
- [8] H. Aoki, H. Nakamura, K. Fumoto, K. Nakahara, and M. Teraoka, “Basic study on non-contact respiration measurement during exercise tolerance test by using Kinect sensor,” 2015 IEEE/SICE Int. Symp. on System Integration, pp. 217-222, 2015. https://doi.org/10.1109/SII.2015.7404981
- [9] J. Satake, T. Ushijima, and Y. Kudo, “Development of respiration measuring robot,” J. Robot. Mechatron., Vol.31, No.5, pp. 715-718, 2019. https://doi.org/10.20965/jrm.2019.p0715
- [10] J. Satake, F. Jogo, K. Hiraki, K. Iwasaki, and T. Shirouzu, “Monitoring system using ceiling camera and mobile robot,” J. Robot. Mechatron., Vol.35, No.1, pp. 206-211, 2023. https://doi.org/10.20965/jrm.2023.p0206
This article is published under a Creative Commons Attribution-NoDerivatives 4.0 Internationa License.