Paper:
Lightweight Encoder with Attention Mechanism for Pipe Recognition Network
Yang Tian
, Xinyu Li, and Shugen Ma
Department of Robotics, Ritsumeikan University
1-1-1 Nojihigashi, Kusatsu, Shiga 525-8577, Japan
Utilizing building information modeling (BIM) for the analysis of existing pipelines necessitates the development of a swift and precise recognition method. Deep learning-based object recognition through imagery has emerged as a potent solution for tackling various recognition tasks. However, the direct application of these models is unfeasible due to their substantial computational requirements. In this research, we introduce a lightweight encoder explicitly for pipe recognition. By optimizing the network architecture using attention mechanisms, it ensures high-precision recognition while maintaining computational efficiency. The experimental results showcased in this study underscore the efficacy of the proposed lightweight encoder and its associated networks.
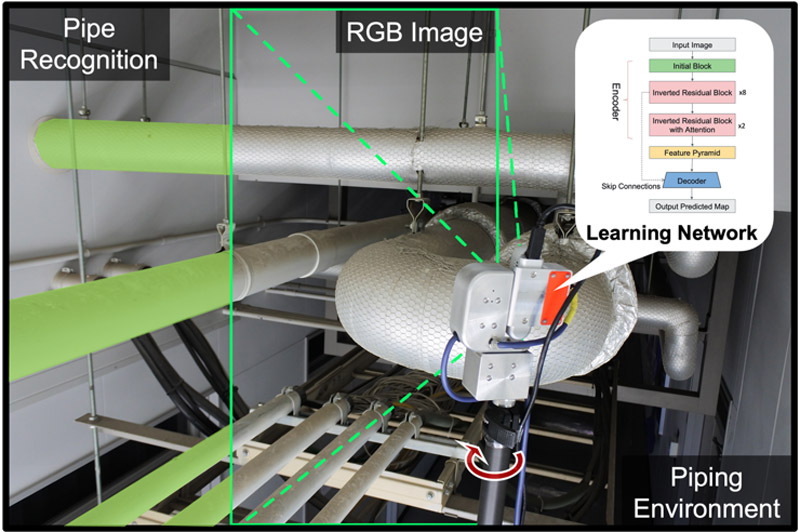
Recognition system in piping environment
- [1] R. Sacks, C. Eastman, G. Lee, and P. Teicholz, “BIM handbook: A guide to building information modeling for owners, designers, engineers, contractors, and facility managers,” John Wiley & Sons, 2018. https://doi.org/10.1002/9781119287568
- [2] S. Alizadehsalehi, A. Hadavi, and J. C. Huang, “From bim to extended reality in aec industry,” Automation in Construction, Vol.116, Article No.103254, 2020. https://doi.org/10.1016/j.autcon.2020.103254
- [3] A. Watson, “Digital buildings–challenges and opportunities,” Advanced Engineering Informatics, Vol.25, No.4, pp. 573-581, 2011. https://doi.org/10.1016/j.aei.2011.07.003
- [4] D. W. Green and M. Z. Southard, “Perry’s chemical engineers’ handbook,” McGraw-Hill Education, 2019.
- [5] D. Huber, B. Akinci, A. A. Oliver, E. Anil, B. E. Okorn, and X. Xiong, “Methods for automatically modeling and representing as-built building information models,” Proc. of the NSF CMMI Research Innovation Conf., Article No.856558, 2011.
- [6] Y.-J. Liu, J.-B. Zhang, J.-C. Hou, J.-C. Ren, and W.-Q. Tang, “Cylinder detection in large-scale point cloud of pipeline plant,” IEEE Trans. on Visualization and Computer Graphics, Vol.19, No.10, pp. 1700-1707, 2013. https://doi.org/10.1109/TVCG.2013.74
- [7] A. K. Patil, P. Holi, S. K. Lee, and Y. H. Chai, “An adaptive approach for the reconstruction and modeling of as-built 3d pipelines from point clouds,” Automation in Construction, Vol.75, pp. 65-78, 2017. https://doi.org/10.1016/j.autcon.2016.12.002
- [8] Y. Lin, Y. Tian, and X. Li, “Development of a pipe recognition network based on attention mechanism,” Proc. of JSME Annual Conf. on Robotics and Mechatronics (Robomech) 2022, 2P1-R08, 2022. https://doi.org/10.1299/jsmermd.2022.2P1-R08
- [9] F. Remondino and S. El-Hakim, “Image-based 3d modelling: A review,” The Photogrammetric Record, Vol.21, No.115, pp. 269-291, 2006. https://doi.org/10.1111/j.1477-9730.2006.00383.x
- [10] J. D. Markley, J. R. Stutzman, and E. N. Harris, “Hybridization of photogrammetry and laser scanning technology for as-built 3d cad models,” Proc. of 2008 IEEE Aerospace Conf., 2008. https://doi.org/10.1109/AERO.2008.4526650
- [11] R. B. Rusu, “Semantic 3d object maps for everyday manipulation in human living environments,” KI – Künstliche Intelligenz, Vol.24, No.4, pp. 345-348, 2010. https://doi.org/10.1007/s13218-010-0059-6
- [12] D. D. Patil and S. G. Deore, “Medical image segmentation: A review,” Int. J. of Computer Science and Mobile Computing, Vol.2, No.1, pp. 22-27, 2013.
- [13] Y. Guo, H. Wang, Q. Hu, H. Liu, L. Liu, and M. Bennamoun, “Deep learning for 3d point clouds: A survey,” IEEE Trans. on Pattern Analysis and Machine Intelligence, Vol.43, No.12, pp. 4338-4364, 2020. https://doi.org/10.1109/TPAMI.2020.3005434
- [14] S. Albawi, T. A. Mohammed, and S. Al-Zawi, “Understanding of a convolutional neural network,” Proc. of 2017 Int. Conf. on Engineering and Technology (ICET), 2017. https://doi.org/10.1109/ICEngTechnol.2017.8308186
- [15] R. Q. Charles, H. Su, M. Kaichun, and L. J. Guibas, “Pointnet: Deep learning on point sets for 3d classification and segmentation,” Proc. of the IEEE Conf. on Computer Vision and Pattern Recognition, pp. 652-660, 2017. https://doi.org/10.1109/CVPR.2017.16
- [16] C. R. Qi, L. Yi, H. Su, and L. J. Guibas, “Pointnet++: Deep hierarchical feature learning on point sets in a metric space,” Advances in Neural Information Processing Systems, Vol.30, 2017.
- [17] R. Geirhos, P. Rubisch, C. Michaelis, M. Bethge, F. A. Wichmann, and W. Brendel, “Imagenet-trained cnns are biased towards texture: Increasing shape bias improves accuracy and robustness,” arXiv preprint, arXiv:1811.12231, 2018. https://doi.org/10.48550/arXiv.1811.12231
- [18] Z. Wu, S. Song, A. Khosla, F. Yu, L. Zhang, X. Tang, and J. Xiao, “3d shapenets: A deep representation for volumetric shapes,” Proc. of the IEEE Conf. on Computer Vision and Pattern Recognition, pp. 1912-1920, 2015. https://doi.org/10.1109/CVPR.2015.7298801
- [19] W. Yuan, T. Khot, D. Held, C. Mertz, and M. Hebert, “Pcn: Point completion network,” Proc. of 2018 Int. Conf. on 3D Vision (3DV), pp. 728-737, 2018. https://doi.org/10.1109/3DV.2018.00088
- [20] M. Aharchi and M. A. Kbir, “A review on 3d reconstruction techniques from 2d images,” Innovations in Smart Cities Applications Edition 3: Proc. of the 4th Int. Conf. on Smart City Applications, Vol.4, pp. 510-522, 2020. https://doi.org/10.1007/978-3-030-37629-1_37
- [21] K. Simonyan and A. Zisserman, “Very deep convolutional networks for large-scale image recognition,” arXiv preprint, arXiv:1409.1556, 2014. https://doi.org/10.48550/arXiv.1409.1556
- [22] K. He, X. Zhang, S. Ren, and J. Sun, “Deep residual learning for image recognition,” Proc. of the IEEE Conf. on Computer Vision and Pattern Recognition, pp. 770-778, 2016. https://doi.org/10.1109/CVPR.2016.90
- [23] C. Szegedy, W. Liu, Y. Jia, P. Sermanet, S. Reed, D. Anguelov, D. Erhan, V. Vanhoucke, and A. Rabinovich, “Going deeper with convolutions,” Proc. of the IEEE Conf. on Computer Vision and Pattern Recognition, 2015. https://doi.org/10.1109/CVPR.2015.7298594
- [24] A. G. Howard, M. Zhu, B. Chen, D. Kalenichenko, W. Wang, T. Weyand, M. Andreetto, and H. Adam, “Mobilenets: Efficient convolutional neural networks for mobile vision applications,” arXiv preprint, arXiv:1704.04861, 2017. https://doi.org/10.48550/arXiv.1704.04861
- [25] X. Zhang, X. Zhou, M. Lin, and J. Sun, “Shufflenet: An extremely efficient convolutional neural network for mobile devices,” Proc. of the IEEE Conf. on Computer Vision and Pattern Recognition, pp. 6848-6856, 2018. https://doi.org/10.1109/CVPR.2018.00716
- [26] M. Tan and Q. Le, “Efficientnet: Rethinking model scaling for convolutional neural networks,” Proc. of Int. Conf. on Machine Learning, pp. 6105-6114, 2019.
- [27] L.-C. Chen, G. Papandreou, I. Kokkinos, K. Murphy, and A. L. Yuille, “Deeplab: Semantic image segmentation with deep convolutional nets, atrous convolution, and fully connected crfs,” IEEE Trans. on Pattern Analysis and Machine Intelligence, Vol.40, No.4, pp. 834-848, 2017. https://doi.org/10.1109/TPAMI.2017.2699184
- [28] L.-C. Chen, Y. Zhu, G. Papandreou, F. Schroff, and H. Adam, “Encoder-decoder with atrous separable convolution for semantic image segmentation,” Proc. of the European Conf. on Computer Vision (ECCV), pp. 801-818, 2018. https://doi.org/10.1007/978-3-030-01234-2_49
- [29] O. Ronneberger, P. Fischer, and T. Brox, “U-net: Convolutional networks for biomedical image segmentation,” Proc. of 18th Int. Conf. on Medical Image Computing and Computer-Assisted Intervention (MICCAI 2015), Munich, Germany, October 5-9, 2015, Part III, pp. 234-241, 2015. https://doi.org/10.1007/978-3-319-24574-4_28
- [30] K. He, X. Zhang, S. Ren, and J. Sun, “Deep residual learning for image recognition,” Proc. of the IEEE Conf. on Computer Vision and Pattern Recognition, pp. 770-778, 2016. https://doi.org/10.1109/CVPR.2016.90
- [31] X. Ding, X. Zhang, J. Han, and G. Ding, “Scaling up your kernels to 31x31: Revisiting large kernel design in cnns,” Proc. of the IEEE/CVF Conf. on Computer Vision and Pattern Recognition, pp. 11963-11975, 2022. https://doi.org/10.1109/CVPR52688.2022.01166
- [32] M. Sandler, A. Howard, M. Zhu, A. Zhmoginov, and L.-C. Chen, “Mobilenetv2: Inverted residuals and linear bottlenecks,” Proc. of the IEEE Conf. on Computer Vision and Pattern Recognition, pp. 4510-4520, 2018. https://doi.org/10.1109/CVPR.2018.00474
- [33] J. Hu, L. Shen, and G. Sun, “Squeeze-and-excitation networks,” Proc. of the IEEE Conf. on Computer Vision and Pattern Recognition, pp. 7132-7141, 2018. https://doi.org/10.1109/CVPR.2018.00745
- [34] P. Ramachandran, B. Zoph, and Q. V. Le, “Searching for activation functions,” arXiv preprint, arXiv:1710.05941, 2017. https://doi.org/10.48550/arXiv.1710.05941
- [35] Y. Tian, C. Ding, Y. F. Lin, S. Ma, and L. Li, “Automatic feature type selection in digital photogrammetry of piping,” Computer-Aided Civil and Infrastructure Engineering, Vol.37, No.10, pp. 1335-1348, 2022. https://doi.org/10.1111/mice.12840
- [36] Y. Liu, L. Chu, G. Chen, Z. Wu, Z. Chen, B. Lai, and Y. Hao, “Paddleseg: A high-efficient development toolkit for image segmentation,” arXiv preprint, arXiv:2101.06175, 2021. https://doi.org/10.48550/arXiv.2101.06175
- [37] A. Howard, M. Sandler, G. Chu, L.-C. Chen, B. Chen, M. Tan, W. Wang, Y. Zhu, R. Pang, V. Vasudevan et al., “Searching for mobilenetv3,” Proc. of the IEEE/CVF Int. Conf. on Computer Vision, pp. 1314-1324, 2019. https://doi.org/10.1109/ICCV.2019.00140
- [38] Y. Tang, K. Han, J. Guo, C. Xu, C. Xu, and Y. Wang, “Ghostnetv2: Enhance cheap operation with long-range attention,” Advances in Neural Information Processing Systems, Vol.35, pp. 9969-9982, 2022.
- [39] M. Fan, S. Lai, J. Huang, X. Wei, Z. Chai, J. Luo, and X. Wei, “Rethinking bisenet for real-time semantic segmentation,” Proc. of the IEEE/CVF Conf. on Computer Vision and Pattern Recognition, pp. 9716-9725, 2021. https://doi.org/10.1109/CVPR46437.2021.00959
- [40] S. Tang, T. Sun, J. Peng, G. Chen, Y. Hao, M. Lin, Z. Xiao, J. You, and Y. Liu, “Pp-mobileseg: Explore the fast and accurate semantic segmentation model on mobile devices,” arXiv preprint, arXiv:2304.05152, 2023. https://doi.org/10.48550/arXiv.2304.05152
- [41] J. Peng, Y. Liu, S. Tang, Y. Hao, L. Chu, G. Chen, Z. Wu, Z. Chen, Z. Yu, Y. Du, Q. Dang, B. Lai, Q. Liu, X. Hu, D. Yu, and Y. Ma, “Pp-liteseg: A superior real-time semantic segmentation model,” arXiv preprint, arXiv:2204.02681, 2022. https://doi.org/10.48550/arXiv.2204.02681
- [42] C. Yu, C. Gao, J. Wang, G. Yu, C. Shen, and N. Sang, “Bisenet v2: Bilateral network with guided aggregation for real-time semantic segmentation,” Int. J. of Computer Vision, Vol.129, pp. 3051-3068, 2021. https://doi.org/10.1007/s11263-021-01515-2
- [43] E. Xie, W. Wang, Z. Yu, A. Anandkumar, J. M. Alvarez, and P. Luo, “Segformer: Simple and efficient design for semantic segmentation with transformers,” Advances in Neural Information Processing Systems, Vol.34, pp. 12077-12090, 2021.
This article is published under a Creative Commons Attribution-NoDerivatives 4.0 Internationa License.