Paper:
Estimation Model for Emotions Based on Pulse
Jiro Morimoto*1
, Akihiro Murakawa*2, Hiroki Fujita*2, Makoto Horio*3, Junji Kawata*1
, Yoshio Kaji*4
, Mineo Higuchi*1
, and Shoichiro Fujisawa*1

*1Faculty of Science and Engineering, Tokushima Bunri University
1314-1 Shido, Sanuki, Kagawa 769-2193, Japan
*2Graduate School of Engineering, Tokushima Bunri University
1314-1 Shido, Sanuki, Kagawa 769-2193, Japan
*3Art and Information Research Institute
1968 Hara, Mure, Takamatsu, Kagawa 761-0123, Japan
*4Faculty of Human Life Sciences, Tokushima Bunri University
180 Nishihama-Boji, Yamashiro-cho, Tokushima 770-8514, Japan
The progressive aging of society has increased expectations for the spread of nursing care robots to support long-term care and welfare services. This research had the goal of developing a communication system as one of the elemental technologies of nursing care robots, along with a method that allows care robots to consider a user’s emotions. The estimation of emotions based on a user’s electroencephalogram and heartbeat has attracted attention. However, users may experience stress when wearing the sensors needed for such measurements. To prevent this system from causing stress in users, we had the goal of developing an estimation model for emotions based on the pulse, which is relatively easy to measure. Various autonomic nervous activity indices (pNN50, RMSSD, LF, HF, LF/HF) were adopted for the estimation model, and transfer functions were established. These indices were considered in time domain and frequency domain analyses of the heart rate variability. The pulse was measured while the user was watching a video and converted into an accelerated plethysmogram using second order differentiation. Then, the autonomic nervous activity indices were calculated. The transfer function from the input to output was identified using these autonomic nervous activity indices as inputs and the responses to a questionnaire that was administered after watching the video as outputs.
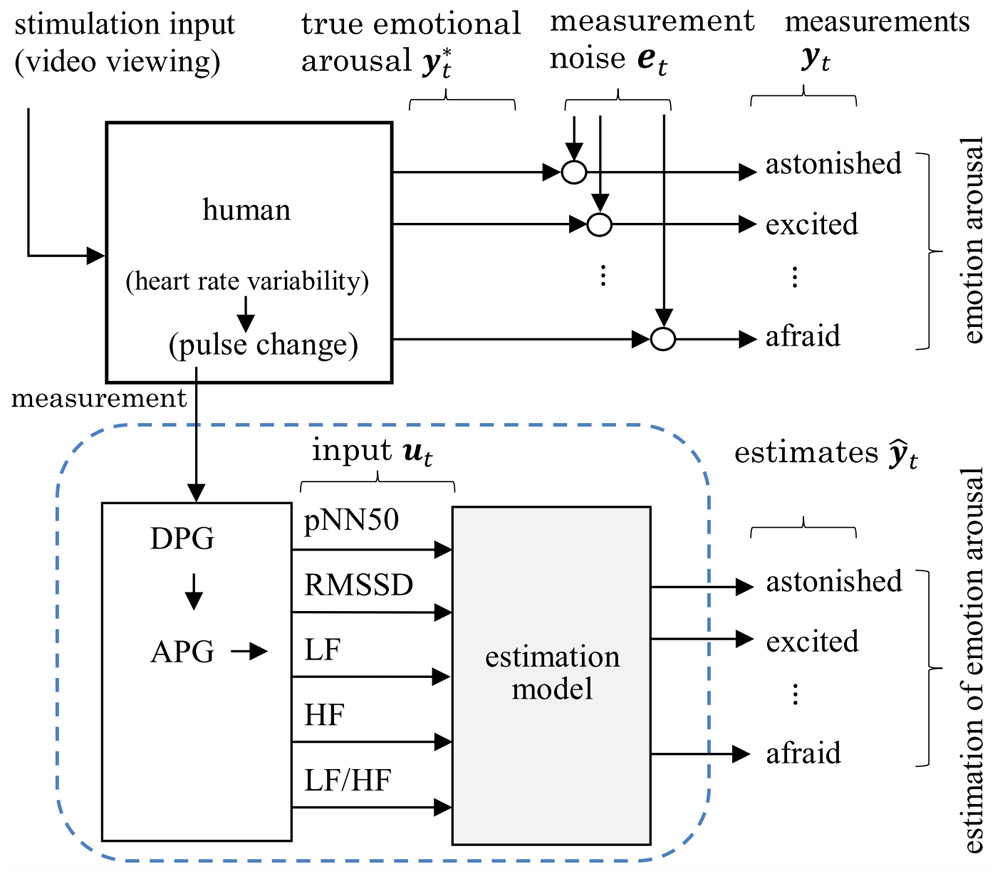
Construction of emotion estimation model
- [1] J. Kawata, J. Morimoto, Y. Kaji, M. Higuchi, K. Matsumoto, M. Booka, and S. Fujisawa, “Development of a Care Robot Based on Needs Survey,” J. Robot. Mechatron., Vol.33, No.4, pp. 739-746, 2021. https://doi.org/10.20965/jrm.2021.p0739
- [2] K. Rattanyu and M. Mizukawa, “Emotion Recognition Based on ECG Signals for Service Robots in the Intelligent Space During Daily Life,” J. Robot. Mechatron., Vol.15, No.5, pp. 582-591, 2011. https://doi.org/10.20965/jaciii.2011.p0582
- [3] J. Chen, D. Jiang, and Y. Zhang, “A Common Spatial Pattern and Wavelet Packet Decomposition Combined Method for EEG-Based Emotion Recognition,” J. Robot. Mechatron., Vol.23, No.2, pp. 274-281, 2019. https://doi.org/10.20965/jaciii.2019.p0274
- [4] S. Ueno, J. Narumon, T. Laohakangvalvi, and M. Sugaya, “Integration of emotion map based on EEG and heart rate variability indices and evaluation of comfort in autonomous vehicles by SD method,” IPSJ SIG Technical Report, Vol.2021-UBI-70, No.7, pp. 1-9, 2021 (in Japanese).
- [5] K. Suzuki, T. Laohakangvalvit, R. Matsubara, and M. Sugaya, “Constructing an Emotion Estimation Model Based on EEG/HRV Indexes Using Feature Extraction and Feature Selection Algorithms,” Sensors, Vol.21, No.9, Article No.2910, 2021. https://doi.org/10.3390/s21092910
- [6] X.-W. Wang, D. Nie, and B.-L. Lu, “Emotional state classification from EEG data using machine learning approach,” Neurocomputing, Vol.129, pp. 94-106, 2014. https://doi.org/10.1016/j.neucom.2013.06.046
- [7] S. Katsigiannis and N. Ramzan, “DREAMER: A Database for Emotion Recognition Through EEG and ECG Signals From Wireless Low-cost Off-the-Shelf Devices,” IEEE J. of Biomedical and Health Informatics, Vol.22, pp. 98-107, 2018. https://doi.org/10.1109/JBHI.2017.2688239
- [8] Y. Kaji, Y. Yamamoto, J. Kawata, J. Morimoto, and S. Fujisawa, “EEG Variations During Measurement of Cognitive Functions Using Biosignal Acquisition Toolkit,” J. Robot. Mechatron., Vol.32, No.4, pp. 753-760, 2020. https://doi.org/10.20965/jrm.2020.p0753
- [9] H. Madokoro and K. Sato, “Estimation of psychological stress levels based on dynamic diversity of facial expressions,” IEICE Technical Report, Vol.109, No.306, pp. 285-290, 2009.
- [10] Y. Ikeda, Y. Okada, R. Horie, and M. Sugaya, “Estimate Emotion Method to Use Facial Expressions and Biological Information,” 2016 Multimedia, Distributed, Cooperative, and Mobile Symposium (DICOMO2016), pp. 149-161, 2016.
- [11] K. Yokoyama and I. Takahashi, “Feasibility Study on Estimating Subjective Fatigue from Heart Rate Time Series,” The IEICE Trans. on Fundamentals of Electronics, Vol.96, No.11, pp. 756-762, 2013 (in Japanese).
- [12] K. Suzuki, R. Matsubara, and M. Sugaya, “Construction of an Emotion Estimation Model Using EEG and Heart Rate Variability Indices as Features by Machine Learning,” The 35th Annual Conf. of the Japanese Society for Artificial Intelligence, Article No.3F2GS10j03, 2021 (in Japanese).
- [13] M. Murugappan, S. Murugappan, and B. S. Zheng, “Frequency Band Analysis of Electrocardiogram (ECG) Signals for Human Emotional State Classification Using Discrete Wavelet Transform (DWT),” J. of Physical Therapy Science, Vol.25, No.7, pp. 753-759, 2013. https://doi.org/10.1589/jpts.25.753
- [14] G. Gulli, R. Cemin, P. Pancera, G. Menegatti, C. Vassanelli, and A. Cevese, “Evidence of parasympathetic impairment in some patients with cardiac syndrome X,” Cardiovascular Research, Vol.52, No.2, pp. 208-216, 2001. https://doi.org/10.1016/S0008-6363(01)00369-8
- [15] H. Fujinaga, “Heart Rate Fluctuation and Affect,” Political Economy Quarterly, Vol.314, pp. 23-57, 2003 (in Japanese). https://doi.org/10.19002/AN00071425.314.23
- [16] H. Takada, M. Takada, and A. Kanayama, “The Significance of ‘LF-component and HF-component which Resulted from Frequency Analysis of Heart Rate’ and ‘the Coefficient of the Heart Rate Variability’ – Evaluation of Autonomic Nerve Function by Acceleration Plethysmography –,” Health Evaluation and Promotion, Vol.32, No.6, pp. 504-512, 2005 (in Japanese). https://doi.org/10.7143/jhep.32.504
- [17] H. Tatsuta, N. Kitano, H. Hoshino, T. Kamo, M. Nohara, T. Tai, T. Tamaki, and K. Nanjo, “The Evaluation of Fatigue by Acceleration Plethysmogram in the Women’s Clinic,” Japanese J. of Occupational Medicine and Traumatology, Vol.61, No.3, pp. 175-179, 2013 (in Japanese).
- [18] B. Takase, “Implication of heart rate variability in critical care medicine,” J. of the Japanese Society of Intensive Care Medicine, Vol.12, No.2, pp. 89-92, 2005 (in Japanese). https://doi.org/10.3918/jsicm.12.89
- [19] H. Hayashi, “Clinical application of heart rate variability,” pp. 28-36, Igaku-Shoin Ltd., 1999 (in Japanese).
- [20] K. Yamaji, “Heart rate to know the mind and the body,” Kyorin-Shoin Publishers, pp. 223-229, 2013 (in Japanese).
- [21] H. Takada, K. Okino, and Y. Niwa, “An Evaluation Method for Heart Rate Variability, by using Acceleration Plethysmography,” Health Evaluation and Promotion, Vol.31, No.4, pp. 547-551, 2004. https://doi.org/10.7143/jhep.31.547
- [22] F. Moscato, M. Granegger, M. Edelmayer, D. Zimpfer, and H. Schima, “Continuous monitoring of cardiac rhythms in left ventricular assist device patients,” Int. Center for Artificial Organs and Transplantation, Vol.38, No.3, pp. 191-198, 2014. https://doi.org/10.1111/aor.12141
- [23] M. Takada, T. Ebara, and Y. Sakai, “The Acceleration Plethysmography System as a New Physiological Technology for Evaluating Autonomic Modulations,” Health Evaluation and Promotion, Vol.35, No.4, pp. 373-377, 2008. https://doi.org/10.7143/jhep.35.373
- [24] J. A. Russell, “A circumplex model of affect,” J. of Personality and Social Psychology, Vol.39, No.6, pp. 1161-1178, 1980. https://doi.org/10.1037/h0077714
- [25] Y. Ono, “Introduction to Biosignal Processing with MATLAB,” pp. 65-79, Corona Publishing Co., Ltd., 2018 (in Japanese).
- [26] Y. Sakurai and J. Shimizu, “Development of the Real-time Joystick Rating Method for Affect and Establishing its Validity,” The Japanese J. of Research on Emotions, Vol.16, No.1, 2008 (in Japanese). https://doi.org/10.4092/jsre.16.87
This article is published under a Creative Commons Attribution-NoDerivatives 4.0 Internationa License.