Paper:
Generation of Optimal Coverage Paths for Mobile Robots Using Hybrid Genetic Algorithm
Tobias Rainer Schäfle*, Marcel Mitschke**, and Naoki Uchiyama*
*Department of Mechanical Engineering, Toyohashi University of Technology
1-1 Hibarigaoka, Tempaku, Toyohashi, Aichi 441-8580, Japan
**Andreas Stihl AG & Co. KG
Badstraße 115, Waiblingen 71336, Germany
This paper presents new optimal offline approaches to solve the coverage path planning problem. A novel hybrid genetic algorithm (HGA), which uses, the turn-away starting point and backtracking spiral algorithms for performing local search, is proposed for grid-based environmental representations. The HGA algorithm is validated using the following three different fitness functions: the number of cell visits, traveling time, and a new energy fitness function based on experimentally acquired energy values of fundamental motions. Computational results show that compared to conventional methods, HGA improves paths up to 38.4%; moreover, HGAs have a consistent fitness for different starting positions in an environment. Furthermore, experimental results prove the validity of the fitness function.
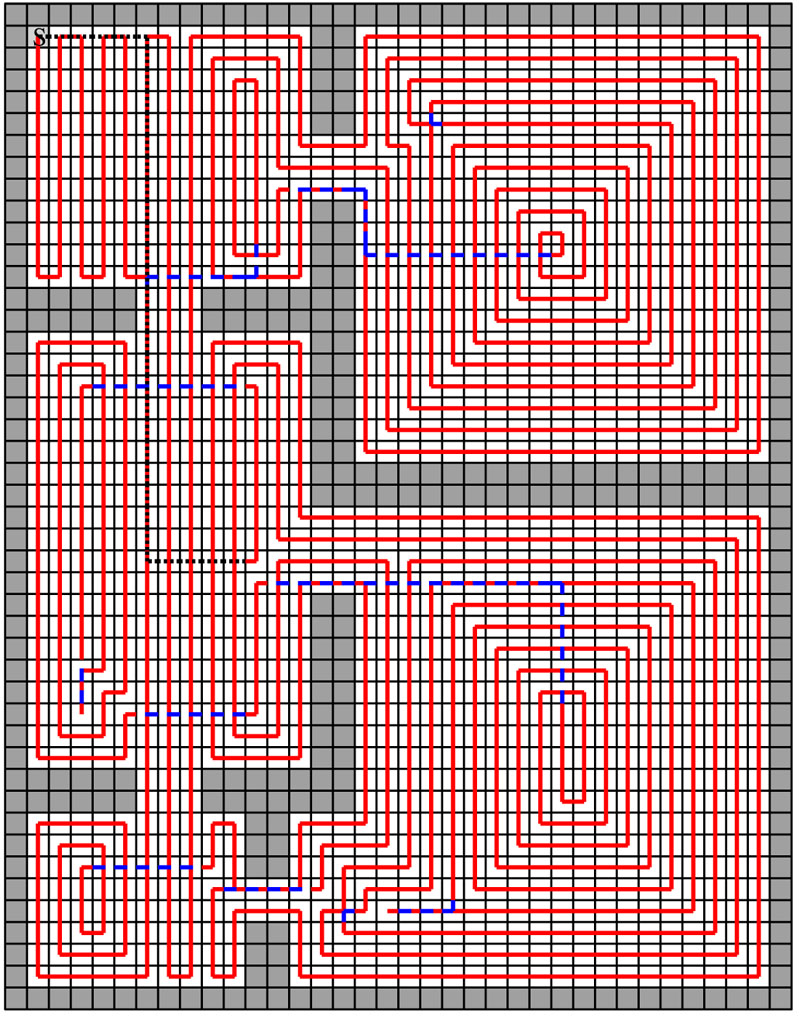
Generation of optimal coverage paths for mobile robots
- [1] K. R. Simba, N. Uchiyama, and S. Sano, “Real-time smooth trajectory generation for nonholonomic mobile robots using bézier curves,” Robotics and Computer-Integrated Manufacturing, Vol.41, pp. 31-42, 2016.
- [2] N. Uchiyama, T. Dewi, and S. Sano, “Collision avoidance control for a human-operated four-wheeled mobile robot,” Proc. of the Institution of Mechanical Engineers, Part C: J. of Mechanical Engineering Science, Vol.228, No.13, pp. 2278-2284, 2014.
- [3] S. Thrun et al., “Robotic mapping: A survey,” Exploring Artificial Intelligence in the New Millennium, Vol.1, pp. 1-35, 2002.
- [4] S. Mohamed, T. R. Schäfle, and N. Uchiyama, “Robust control of a redundant wheeled drive system for energy saving and fail safe motion,” Advances in Mechanical Engineering, Vol.9, No.5, 1687814017702343, 2017.
- [5] B. Yang, C. Guo, C. S. Jensen, M. Kaul, and S. Shang, “Stochastic skyline route planning under time-varying uncertainty,” Proc. of 2014 IEEE 30th Int. Conf. on Data Engineering, pp. 136-147, 2014.
- [6] C. Guo, B. Yang, O. Andersen, C. S. Jensen, and K. Torp, “Ecosky: Reducing vehicular environmental impact through eco-routing,” Proc. of 2015 IEEE 31st Int. Conf. on Data Engineering, pp. 1412-1415, 2015.
- [7] B. Yang, C. Guo, Y. Ma, and C. S. Jensen, “Toward personalized, context-aware routing,” The VLDB J. of the Int. J. on Very Large Data Bases, Vol.24, No.2, pp. 297-318, 2015.
- [8] A. Farid and T. Matsumaru, “Path planning in outdoor pedestrian settings using 2d digital maps,” J. Robot. Mechatron., Vol.31, No.3, pp. 464-473, 2019.
- [9] R. Takemura and G. Ishigami, “Traversability-based rrt* for planetary rover path planning in rough terrain with lidar point cloud data,” J. Robot. Mechatron., Vol.29, No.5, pp. 838-846, 2017.
- [10] E. M. Arkin, S. P. Fekete, and J. S. B. Mitchell, “Approximation algorithms for lawn mowing and milling,” Computational Geometry, Vol.17, Nos.1-2, pp. 25-50, 2000.
- [11] E. Galceran and M. Carreras, “A survey on coverage path planning for robotics,” Robotics and Autonomous Systems, Vol.61, No.12, pp. 1258-1276, 2013.
- [12] A. Khan, I. Noreen, and Z. Habib, “On complete coverage path planning algorithms for non-holonomic mobile robots: Survey and challenges,” J. Inf. Sci. Eng., Vol.33, No.1, pp. 101-121, 2017.
- [13] H. Choset and P. Pignon, “Coverage path planning: The boustrophedon cellular decomposition,” Field and Service Robotics, pp. 203-209, 1998.
- [14] E. U. Acar, H. Choset, A. A. Rizzi, P. N. Atkar, and D. Hull, “Morse decompositions for coverage tasks,” The Int. J. of Robotics Research, Vol.21, No.4, pp. 331-344, 2002.
- [15] E. U. Acar, H. Choset, and J. Y. Lee, “Sensor-based coverage with extended range detectors,” IEEE Trans. on Robotics, Vol.22, No.1, pp. 189-198, 2006.
- [16] A. Zelinsky, R. A. Jarvis, J. C. Byrne, and S. Yuta, “Planning paths of complete coverage of an unstructured environment by a mobile robot,” Proc. of Int. Conf. on Advanced Robotics, Vol.13, pp. 533-538, 1993.
- [17] Y. Gabriely and E. Rimon, “Spanning-tree based coverage of continuous areas by a mobile robot,” Annals of Mathematics and Artificial Intelligence, Vol.31, Nos.1-4, pp. 77-98, 2001.
- [18] Y. Gabriely and E. Rimon, “Competitive on-line coverage of grid environments by a mobile robot,” Computational Geometry, Vol.24, No.3, pp. 197-224, 2003.
- [19] E. Gonzalez, O. Alvarez, Y. Diaz, C. Parra, and C. Bustacara, “BSA: a complete coverage algorithm,” Proc. of the 2005 IEEE Int. Conf. on Robotics and Automation (ICRA), pp. 2040-2044, 2005.
- [20] S. X. Yang and C. Luo, “A neural network approach to complete coverage path planning,” IEEE Trans. on Systems, Man, and Cybernetics, Part B (Cybernetics), Vol.34, No.1, pp. 718-724, 2004.
- [21] C. Luo and S. X. Yang, “A bioinspired neural network for real-time concurrent map building and complete coverage robot navigation in unknown environments,” IEEE Trans. on Neural Networks, Vol.19, No.7, pp. 1279-1298, 2008.
- [22] M. Kapanoglu, M. Ozkan, O. Parlaktuna et al., “Pattern-based genetic algorithm approach to coverage path planning for mobile robots,” Proc. of Int. Conf. on Computational Science, pp. 33-42, 2009.
- [23] X. Miao, J. Lee, and B.-Y. Kang, “Scalable coverage path planning for cleaning robots using rectangular map decomposition on large environments,” IEEE Access, Vol.6, pp. 38200-38215, 2018.
- [24] J. Song and S. Gupta, “ϵ*: An online coverage path planning algorithm,” IEEE Trans. on Robotics, Vol.34, pp. 526-533, 2018.
- [25] M. Mitschke, N. Uchiyama, and O. Sawodny, “Online coverage path planning for a mobile robot considering energy consumption,” IEEE Int. Conf. on Automation Science and Engineering (CASE), pp. 1473-1478, 2018.
- [26] T. Sasaki, G. Enriquez, T. Miwa, and S. Hashimoto, “Adaptive path planning for cleaning robots considering dust distribution,” J. Robot. Mechatron., Vol.30, No.1, pp. 5-14, 2018.
- [27] P. A. Jimenez, B. Shirinzadeh, A. Nicholson, and G. Alici, “Optimal area covering using genetic algorithms,” Proc. of the 2007 IEEE/ASME Int. Conf. on Advanced Intelligent Mechatronics, pp. 1-5, 2007.
- [28] R. Mannadiar and I. Rekleitis, “Optimal coverage of a known arbitrary environment,” Proc. of the 2010 IEEE Int. Conf. on Robotics and Automation (ICRA), pp. 5525-5530, 2010.
- [29] T.-K. Lee, S.-H. Baek, Y.-H. Choi, and S.-Y. Oh, “Smooth coverage path planning and control of mobile robots based on high-resolution grid map representation,” Robotics and Autonomous Systems, Vol.59, No.10, pp. 801-812, 2011.
- [30] S. Bochkarev and S. L. Smith, “On minimizing turns in robot coverage path planning,” Proc. of the 2016 IEEE Int. Conf. on Automation Science and Engineering (CASE), pp. 1237-1242, 2016.
- [31] A. E. F. Ryerson and Q. Zhang, “Vehicle path planning for complete field coverage using genetic algorithms,” Agricultural Engineering Int.: CIGR J., Vol.IX, pp. 1-11, 2007.
- [32] M. A. Yakoubi and M. T. Laskri, “The path planning of cleaner robot for coverage region using genetic algorithms,” J. of Innovation in Digital Ecosystems, Vol.3, No.1, pp. 37-43, 2016.
- [33] M. G. Sadek, A. E. Mohamed, and A. M. El-Garhy, “Augmenting multi-objective genetic algorithm and dynamic programming for online coverage path planning,” Proc. 13th Int. Conf. on Computer Engineering and Systems (ICCES), pp. 475-480, 2018.
- [34] T. R. Schäfle, S. Mohamed, N. Uchiyama, and O. Sawodny, “Coverage path planning for mobile robots using genetic algorithm with energy optimization,” Proc. of Int. Electronics Symp. (IES), pp. 99-104, 2016.
- [35] R. Siegwart, I. R. Nourbakhsh, and D. Scaramuzza, “Introduction to Autonomous Mobile Robots,” MIT Press, 2011.
- [36] D. E. Goldberg, “Genetic algorithms in search optimization and machine learning,” Addison-Wesley Professional, 1989.
- [37] M. Mitchell, “An Introduction to Genetic Algorithms,” MIT Press, 1998.
- [38] E. H. L. Aarts and J. K. Lenstra, “Local Search in Combinatorial Optimization,” Princeton University Press, 2003.
- [39] P. Moscato, “Memetic algorithms,” P. M. Pardalos and M. G. C. Resende (Eds.), “Handbook of Applied Optimization,” pp. 157-167, Oxford University Press, 2002.
- [40] P. Moscato and C. Cotta, “A gentle introduction to memetic algorithms,” F. Glover and G. A. Kochenberger (Eds.), “Handbook of Metaheuristics,” pp. 105-144, Springer, 2003.
This article is published under a Creative Commons Attribution-NoDerivatives 4.0 Internationa License.