Paper:
Utilization of Unmanned Aerial Vehicle, Artificial Intelligence, and Remote Measurement Technology for Bridge Inspections
Pang-jo Chun*1, Ji Dang*2, Shunsuke Hamasaki*1, Ryosuke Yajima*1, Toshihiro Kameda*3, Hideki Wada*4, Tatsuro Yamane*5, Shota Izumi*6, and Keiji Nagatani*1
*1The University of Tokyo
7-3-1 Bunkyo-ku, Hongo, Tokyo 113-8656, Japan
*2Saitama University
255 Shimo-Okubo, Sakura-ku, Saitama 338-8570, Japan
*3University of Tsukuba
1-1-1 Tennodai, Tsukuba, Ibaraki 305-8573, Japan
*4Sin Nippon Nondestructive Inspection Co., Ltd.
4-10-13 Ibori, Kokura-Kitaku, Kitakyushu 803-8517, Japan
*5The University of Tokyo
5-1-5 Kashiwanoha, Kashiwa, Chiba 277-8561, Japan
*6Ehime University
3 Bunkyo-cho, Matsuyama, Ehime 790-8577, Japan
In recent years, aging of bridges has become a growing concern, and the danger of bridge collapse is increasing. To appropriately maintain bridges, it is necessary to perform inspections to accurately understand their current state. Until now, bridge inspections have involved a visual inspection in which inspection personnel come close to the bridges to perform inspection and hammering tests to investigate abnormal noises by hammering the bridges with an inspection hammer. Meanwhile, as there are a large number of bridges (for example, 730,000 bridges in Japan), and many of these are constructed at elevated spots; the issue is that the visual inspections are laborious and require huge cost. Another issue is the wide disparity in the quality of visual inspections due to the experience, knowledge, and competence of inspectors. Accordingly, the authors are trying to resolve or ameliorate these issues using unmanned aerial vehicle (UAV) technology, artificial intelligence (AI) technology, and telecommunications technology. This is discussed first in this paper. Next, the authors discuss the future prospects of bridge inspection using robot technology such as a 3-D model of bridges. The goal of this paper is to show the areas in which deployment of the UAV, robots, telecommunications, and AI is beneficial and the requirements of these technologies.
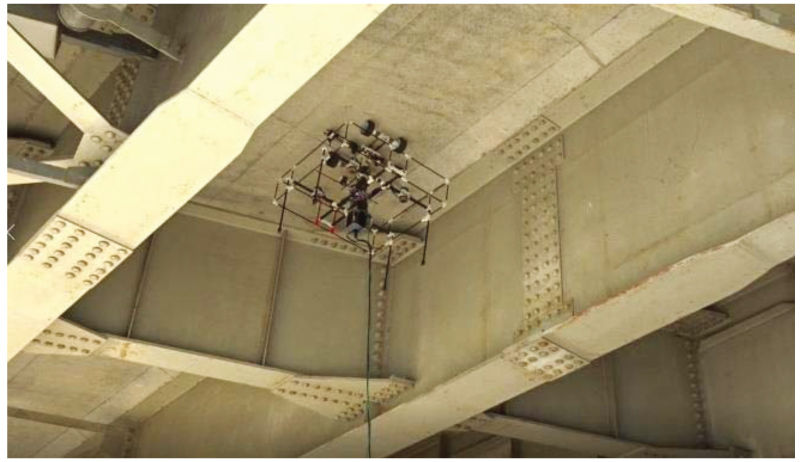
New UAV for bridge inspection
- [1] Ministry of Land, Infrastructure, Transport and Tourism (MLIT), “Roads In Japan,” Road Bureau, 2018.
- [2] “2017 report card for America’s infrastructure,” American Society of Civil Engineers, 2017.
- [3] P. Chun, T. Yamane, S. Izumi, and T. Kameda, “Evaluation of Tensile Performance of Steel Members by Analysis of Corroded Steel Surface Using Deep Learning,” Metals, Vol.9, No.12, p. 1259, 2019.
- [4] P. Chun, K. Tsukada, M. Kusumoto, and K. Okubo, “Investigation and repair plan for abraded steel bridge piers: case study from Japan,” Proc. of the Institution of Civil Engineers-Forensic Engineering, Vol.172, No.1, pp. 11-18, 2019.
- [5] T. Tamakoshi, M. Ookubo, M. Hoshino, Y. Yokoi, and Y. Kowase, “Reference to MLIT’s bridge inspection manual (2013) – Photographs related to damage rating and maintenance urgency ratings –,” Technical Note of NILIM, No.748, 2013.
- [6] Ministry of Land, Infrastructure, Transport and Tourism (MLIT), “On the recent situation in the construction industry and skilled workers,” 2016.
- [7] A. Masuda, A. Tanaka, Y. Higashi, and N. Miura, “Reliable Activation of an EPM-Based Clinging Device for Aerial Inspection Robots,” J. Robot. and Mechatron., Vol.31, No.6, pp. 827-836, 2019.
- [8] M. Nakao, E. Hasegawa, T. Kudo, and N. Sawasaki, “Development of a Bridge Inspection Support Robot System Using Two-Wheeled Multicopters,” J. Robot. and Mechatron., Vol.31, No.6, pp. 837-844, 2019.
- [9] K. Hidaka, D. Fujimoto, and K. Sato, “Autonomous Adaptive Flight Control of a UAV for Practical Bridge Inspection Using Multiple-Camera Image Coupling Method,” J. Robot. and Mechatron., Vol.31, No.6, pp. 845-854, 2019.
- [10] Y. Takada, Y. Tokura, Y. Matsumura, T. Tanaka, and T. Kanada, “Wall Inspection Robot with Maneuvering Assist Control System Against Crosswind,” J. Robot. and Mechatron., Vol.30, No.3, pp. 416-425, 2018.
- [11] T. Miura, M. Nitta, H. Wada, and H. Nakamura, “The development of inner defect detecting method by using UAV having hammering mechanism and application to actual bridges,” J. of Structural Engineering (JSCE), Vol.65, A, pp. 607-614, 2019 (in Japanese).
- [12] P. Chun, H. Yamashita, and S. Furukawa, “Bridge damage severity quantification using multipoint acceleration measurement and artificial neural networks,” Shock and Vibration, Vol.2015, Article ID: 789384, 2015.
- [13] P. Chun, T. Yamane, S. Izumi, and N. Kuramoto, “Development of a Machine Learning-based Damage Identification Method using Multi-point Simultaneous Acceleration Measurement Results,” Sensors, Vol.20, No.10, p. 2780, 2020.
- [14] A. Shrestha, J. Dang, and X. Wang, “Development of a Smart-device based Vibration-measurement System: Effectiveness examination and application cases to existing structure,” Structural Control and Health Monitoring, Vol.25, No.3, p. e2120, 2018.
- [15] A. Shrestha, J. Dang, X. Wang, and S. Matsunaga, “Smartphone based Bridge Seismic Monitoring System and Long-Term Field Application Tests,” J. of Structural Engineering, Vol.146, Issue 2, doi: 10.1061/(ASCE)ST.1943-541X.0002513, 2020.
- [16] S. D. Glaser and A. Tolman, “Sense of Sensing: From Data to Informed Decisions for the Built Environment,” J. of infrastructure systems, Vol.14, No.1, pp. 4-14, 2008.
- [17] S. N. Pakzad, G. L. Fenves, S. Kim, and D. E. Culler, “Design and Implementation of Scalable Wireless Sensor Network for Structural Monitoring,” J. of infrastructure systems, Vol.14, No.1, pp. 89-101, 2008.
- [18] S. Guan, J. A. Bridge, C. Li, and N. J. DeMello, “Smart Radar Sensor Network for Bridge Displacement Monitoring,” J. of Bridge Engineering, Vol.23, No.12, p. 04018102, 2018.
- [19] A. V. T. Bardram, M. D. Larsen, K. M. Malarski, M. N. Petersen, and S. Ruepp, “LoRaWan Capacity Simulation and Field Test in a Harbour Environment,” 2018 Third Int. Conf. on Fog and Mobile Edge Computing (FMEC), pp. 193-198, 2018.
- [20] P. Chun, S. Izumi, and T. Yamane, “Automatic detection method of cracks from concrete surface imagery using two-step Light Gradient Boosting Machine,” Computer-Aided Civil and Infrastructure Engineering, pp. 1-12, 2020.
- [21] Y. Okazaki, S. Okazaki, S. Asamoto, and P. Chun, “Applicability of machine learning to a crack model in concrete bridges,” Computer-Aided Civil and Infrastructure Engineering, pp. 1-18, 2020.
- [22] T. Yamane and P. Chun, “Crack detection from a concrete surface image based on semantic segmentation using deep learning,” J. of Advanced Concrete Technol., Vol.18, No.9, pp. 493-504, 2020.
- [23] T. Yamane, Y. Ueno, K. Kanai, S. Izumi, and P. Chun, “Reflection of Crack Location to 3D Model of Bridge Using Semantic Segmentation,” Intelligence, Informatics and Infrastructure (in press).
- [24] P. Chun, I. Ujike, K. Mishima, M. Kusumoto, and S. Okazaki, “Random Forest-based evaluation technique for internal damage in reinforced concrete featuring multiple nondestructive testing results,” Construction and Building Materials, Vol.253, p. 119238, 2020.
- [25] H. S. Moon, S. Ok, P. Chun, and Y. M. Lim, “Artificial neural network for vertical displacement prediction of a bridge from strains (Part 1): Girder bridge under moving vehicles,” Applied Sciences, Vol.9, No.14, p. 2881, 2019.
- [26] H. S. Moon, P. Chun, M. K. Kim, and Y. M. Lim, “Artificial Neural Network for Vertical Displacement Prediction of a Bridge from Strains (Part 2): Optimization of Strain-Measurement Points by a Genetic Algorithm under Dynamic Loading,” Applied Sciences, Vol.10, No.3, p. 777, 2020.
- [27] C. N. N. Karina, P. Chun, and K. Okubo, “Tensile strength prediction of corroded steel plates by using machine learning approach,” Steel and Composite Structures, Vol.24, No.5, pp. 635-641, 2017.
- [28] J. Shi, J. Dang, and R. Zuo, “Bridge Damage Cropping-and-Stitching Segmentation Using Fully Convolutional Network Based on Images from UAVs,” Proc. of IABMAS 2020, 2021 (in press).
This article is published under a Creative Commons Attribution-NoDerivatives 4.0 Internationa License.