Paper:
Screening Sleep Disordered Breathing with Noncontact Measurement in a Clinical Site
Yutaka Matsuura*1, Hieyong Jeong*2,†, Kenji Yamada*3, Kenji Watabe*4, Kayo Yoshimoto*5, and Yuko Ohno*1
*1Division of Health Science, Graduate School of Medicine, Osaka University
1-7 Yamadaoka, Suita, Osaka 565-0871, Japan
*2Department of Robotics & Design for Innovative Healthcare, Graduate School of Medicine, Osaka University
1-7 Yamadaoka, Suita, Osaka 565-0871, Japan
*3Department of Biodesign for Healthcare Innovation, Graduate School of Medicine, Osaka University
1-3 Yamadaoka, Suita, Osaka 565-0871, Japan
*4Osaka University Hospital
2-15 Yamadaoka, Suita, Osaka 565-0871, Japan
*5Department of Electrical and Information Engineering, Graduate School of Engineering, Osaka City University
3-3-138 Sugimoto, Sumiyoshi-ku, Osaka 545-8585, Japan
†Corresponding author
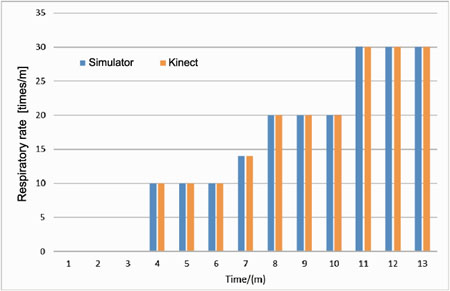
Respiratory rate from simulator and Kinect
- [1] Y. Koike, A. Noda, S. Nakata, and N. Ozaki, “The foundation of sleep medicine,” 1st edition, Vol.1, The University of Nagoya Press, pp. 30-40, 2010 (in Japanese).
- [2] S. Momomura, T. Akashiba, E. Asanoi, S. Ando, K. Kario, T. Shiomi et al., “Guidelines for diagnosis and treatment of sleep disordered breathing in cardiovascular disease,” Circulation J., Vol.74, Suppl II, pp. 963-1051, 2010 (in Japanese).
- [3] R. Ellen, S. C. Marshall, M. Palayew, F. J. Molnar, K. Wilson, and M. Man-Son-Hing, “Systematic review of motor vehicle crash risk in persons with sleep apnea,” J. Clin Sleep Med. Vol.2, No.2, pp. 193-200, 2006.
- [4] A. S. Gami, D. E. Howard, E. J. Olson, and V. K. Somers, “Day-night pattern of sudden death in obstructive sleep apnea,” New England J. of Medicine, Vol.352, No.12, pp. 1206-1214, 2005.
- [5] A. Kales, R. Cadieux, L. Shaw, A. Vela-Bueno, E. Bixler, D. Schneck et al., “Sleep apnoea in a hypertensive population,” The Lancet, Vol.324, No.8410, pp. 1005-1008, 1984.
- [6] A. G. Logan, S. M. Perlikowski, A. Mente, A. Tisler, R. Tkacova, M. Niroumand et al., “High prevalence of unrecognized sleep apnoea in drug-resistant hypertension,” J. of hypertension, Vol.19, No.12, pp. 2271-2277, 2001.
- [7] O. Oldenburg, B. Lamp, L. Faber, H. Teschler, D. Horstkotte, and V. Töpfer, “Sleep-disordered breathing in patients with symptomatic heart failure A contemporary study of prevalence in and characteristics of 700 patients,” European J. of heart failure, Vol.9, No.3, pp. 251-257, 2007.
- [8] M. Shimpo, “The effect of SDB on prognosis of heart failure,” Progress in Medicine, Vol.09, No.9, pp. 2033-2037, 2011 (in Japanese).
- [9] Y. Kayukawa, “Prevalence, sex difference and age difference of obstructive sleep apnea syndrome,” Biomedicene and Therapeutics, Vol.30, pp. 179-182, 1996 (in Japanese).
- [10] H. Miwa, “Heart Rate and Respiratory Rate Measurement Using Body-Sound,” J. of Robotics and Mechatronics, Vol.23, No.3, pp. 434-42, 2011.
- [11] N. Sasaki, M. Kagawa, K. Suzumura, and T. Matsui, “Sleep stage estimation by body movement index and respiratory interval indices using microwave radars,” Medical and Biological Engineering, Vol.53, No.4, pp. 209-216, 2015 (in Japanese).
- [12] M. Schätz, F. Centonze, J. Kuchyňka, O. Ťupa, O. Vyšata, O. Geman et al., “Statistical recognition of breathing by MS Kinect depth sensor,” 2015 Int. Workshop on Computational Intelligence for Multimedia Understanding (IWCIM), pp. 1-4, 2015.
- [13] M. Kagawa, H. Tojima, and T. Matsui, “Non-contact diagnostic system for sleep apnea-hypopnea syndrome based on amplitude and phase analysis of thoracic and abdominal Doppler radars,” Medical & biological engineering & computing. Vol.54, No.5, pp. 789-798, 2015.
- [14] H. Aoki, M. Miyazaki, H. Nakamura, R. Furukawa, R. Sagawa, and H. Kawasak, “Simple Non-contact Respiration Measurement Using Active 3-D Sensor,” The institute of electronics, information and communication engineers (IEICE) Technical Report, Vol.111, No.482, pp. 101-106, 2012 (in Japanese).
- [15] M. C. Yu, J. L. Liou, S. W. Kuo, M. S. Lee, and Y. P. Hung, “Noncontact respiratory measurement of Volume change using depth camera,” Conf. Proc., Annual Int. Conf. of the IEEE Engineering in Medicine and Biology Society (EMBC), Osaka, Japan, pp. 2371-2374, 2012.
- [16] F. Snyder, J. A. Hobson, D. F. Morrison, and F. Goldfrank, “Changes in respiration, heart rate, and systolic blood pressure in human sleep,” J. of Applied Physiology, Vol.19, No.3, pp. 417-422. 1964.
- [17] E. Tabachnik, N. L. Muller, A. C. Bryan, and H. Levison, “Changes in ventilation and chest wall mechanics during sleep in normal adolescents,” J. of Applied Physiology, Vol.51, No.3, pp. 557-564, 1981.
- [18] K. Jo and H, Hagiwara, “Evaluation of Body Movement during sleep using an infrared motion sensor,” Medical and Biological Engineering, Vol.47, No.1, pp. 7-14, 2009 (in Japanese).
- [19] X. Long, P. Fonseca, J. Foussier, R. Haakma, and R. M. Aarts, “Sleep and wake classification with actigraphy and respiratory effort using dynamic warping,” IEEE J. of biomedical and health informatics, Vol.18, No.4, pp. 1272-1284, 2014.
- [20] F. Kawana, “Clinical Evaluation of Sleep Disorders,” Revised Edition Life science, pp. 43-55, 2016 (in Japanese).
- [21] Y. Matsumoto, K. Nakajima, T. Tamura, K. Tanaka, and S. Tanaka, “A real time monitor for evaluating posture change and respiration rate using image sequence analysis,” Trans. of the Institute of Systems, Control and Information Engineers, Vol.14, No.4, pp. 173-179, 2009 (in Japanese).
- [22] M. kagawa, Y. Yoshida, S. Suzuki, A. Kurita, and T. Matui, “Reduction of body motion noise in a non-contact vital sign monitoring system for aged care settings using two microwave radars,” An Official J. of the Japan Association for Medical Informatics, Vol.30, No.2, pp. 85-94, 2010 (in Japanese).
- [23] Y. Mori and S. Kido, “Monitoring system for elderly people using passive RFID tags,” J. of Robotics and Mechatronics, Vol.26, No.5, 2014.
- [24] K. Soga, H. Chikaoka, and Y. Iikura, “Sudden infant death syndrome,” J. of the showa medical association, Vol.60, No.2, pp. 154-156, 2000 (in Japanese).
- [25] M. Al-Mardini, F. Aloul, A. Sagahyroon, and L. Al-Husseini, “Classifying obstructive sleep apnea using smartphones,” J. of biomedical informatics, Vol.52, pp. 251-259, 2014.
- [26] E. Dafna, T. Rosenwein, A. Tarasiuk, and Y. Zigel, “Breathing rate estimation during sleep using audio signal analysis,” 37th Annual Int. Conf. of the IEEE Engineering in Medicine and Biology Society (EMBC), Milan, Italy, pp. 5981-5984, 2015.
- [27] Y. Nam, B. A. Reyes, and K. H. Chon, “Estimation of respiratory rates using the built-in microphone of a smartphone or headset,” IEEE J. of biomedical and health informatics, Vol.20, No.6, 2016.
- [28] Y. Ren, C. Wang, J. Yang, and Y. Chen, “Fine-grained sleep monitoring: hearing your breathing with smartphones,” 2015 IEEE Conf. on Computer Communications (INFOCOM), Hong Kong, pp. 1194-1202, 2015.
This article is published under a Creative Commons Attribution-NoDerivatives 4.0 Internationa License.