Paper:
A Hybrid Nonlinear ANC for Speech Recovery Using Both Bone- and Air-Conducted Measurements
Ran Xiao*1, Yaping Ma*2, Boyan Huang*2, Yegui Xiao*3, and Koji Hasegawa*4
*1NEC Solution Innovators, Ltd.
1-40-1 Tomominami, Asaminami-ku, Hiroshima 731-3168, Japan
*2Harbin Institute of Technology
Box 351, Harbin 150001, China
*3Prefectural University of Hiroshima
1-1-71 Ujina-Higashi, Minami-ku, Hiroshima 734-8558, Japan
*4Hiroshima Prefectural Technology Research Institute
10-52 Motomachi, Naka-ku, Hiroshima 730-8511, Japan
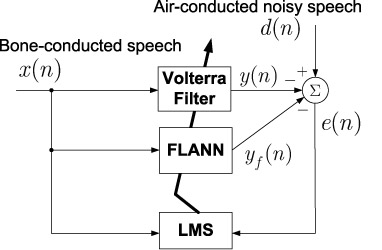
- [1] S. Gannot, D. Burshtein, and E. Weinstein, “Iterative and sequential Kalman filter-based speech enhancement algorithms,” IEEE Trans. Speech, Audio Process., Vol.6, No.4, pp. 373-385, Jul. 1998.
- [2] S. Boll, “Suppression of acoustic noise in speech using spectral subtraction,” IEEE Trans. Acoust. Speech Signal Process., Vol.27, No.2, pp. 113-120, 1979.
- [3] N. Upadhyay and A. Karmakar, “Single channel speech enhancement utilizing iterative processing of multi-band spectral subtraction algorithm,” Proc. 2012 Int. Conf. Power, Control, and Embedded Systems, 2012.
- [4] X. Zou, P. Jancovic, J. Liu, and M. Kokuer, “Speech signal enhancement based on MAP algorithm in the ICA space,” IEEE Trans. Signal Process., Vol.56, No.5, pp. 1812-1820, May 2008.
- [5] N. Yousefian and P. C. Loizou, “A dual-microphone speech enhancement algorithm based on the coherence function,” IEEE Trans. Audio, Speech, Lang. Process., Vol.20, No.2, pp. 599-609, Feb. 2012.
- [6] J. Yu, L. Zhang, and Z. Zhou, “A novel voice collection scheme based on bone-conduction,” Proc. ISCIT2005, pp. 1126-1129, 2005.
- [7] T. Shimamura and T. Tamiya, “Learning for bone-conducted speech via adaptive and neural filters,” Proc. Int. Conf. Signals and Electronic Systems, Sep. 2006.
- [8] Y. Xiao, R. Xiao, B. Huang, and K. Hasegawa, “A nonlinear adaptive noise canceller for speech enhancement using Volterra filter,” Proc. of 2014 Int. Conf. Advanced Mechatronic Systems, pp. 204-208, Aug. 2014.
- [9] B. Huang, Y. Xiao, J. Sun, G. Wei, and H. Wei, “Speech enhancement based on FLANN using both bone- and air-conducted measurements,” Proc. APSIPA 2014, Dec. 2014.
- [10] V. Volterra, “Theory of functionals and of integrals and integro-differential equations,” New York: Dover Publications, 1959.
- [11] S. Orcioni, M. Pirani, and C. Turchetti, “Advances in Lee-Schetzen method for Volterra filter identification,” Multidimensional Systems and Signal Processing, Vol.16, No.3, pp. 265-284, 2005.
- [12] Y. Zhang, S. Bai, P. Chen, and S. Qu, “A novel second-order DFP-based Volterra filter and its applications to chaotic time series prediction,” Proc. 31st Chinese Control Conf., pp. 1036-1039, 2012.
- [13] Y. H. Pao, “Adaptive pattern recognition and neural networks,” Reading, MA: Addison-Wesley, 1989.
- [14] G. Panda and D. P. Das, “Functional link artificial neural network for active control of nonlinear noise processes,” Proc. Int. Workshop on Acoustic Echo and Noise Control, pp. 163-166, Sep. 2003.
This article is published under a Creative Commons Attribution-NoDerivatives 4.0 Internationa License.