Research Paper:
Automated Impaction Angulation Measurement of Mandibular Third Molars for Winter’s Classification Using Deep Learning
Md. Anas Ali*, Daisuke Fujita*
, Hiromitsu Kishimoto**
, Yuna Makihara**, Kazuma Noguchi**, and Syoji Kobashi*,

*Graduate School of Engineering, University of Hyogo
2167 Shosha, Himeji, Hyogo 671-2280, Japan
Corresponding author
**Hyogo Medical University
1-1 Mukogawa-cho, Nishinomiya, Hyogo 663-8501, Japan
Impacted third molar extraction, particularly of mandibular teeth, is a common procedure performed to alleviate pain, infection, and misalignment. Accurate diagnosis and classification of impaction types are crucial for effective treatment planning. This study introduces a novel algorithm for automatically measuring the impaction angles of mandibular third molars (T32 and T17) from orthopantomogram (OPG) images. The proposed method is based on deep learning techniques, including segmentation and key point detection models. It categorizes impactions into Winter’s classification: distoangular, mesioangular, horizontal, vertical, and other on both sides, using the measured angles. The proposed method used 450 OPGs, achieving high mandibular molar segmentation accuracy with dice similarity coefficients (DSC) values of 0.9058–0.9162 and intersection over union (IOU) scores of 0.82–0.84. The object keypoint similarity (OKS) for detecting the four corner points of each molar was 0.82. Angle measurement analysis showed 80% accuracy within ±5° deviation for distoangular impaction of T32 and within ±8° for T17. The F1-scores for mesioangular classifications were 0.88 for T32 and 0.91 for T17, with varying performance in other categories. Nonetheless, the predicted angles aid in identifying impaction types, showcasing the method’s potential to enhance dental diagnostics and treatment planning.
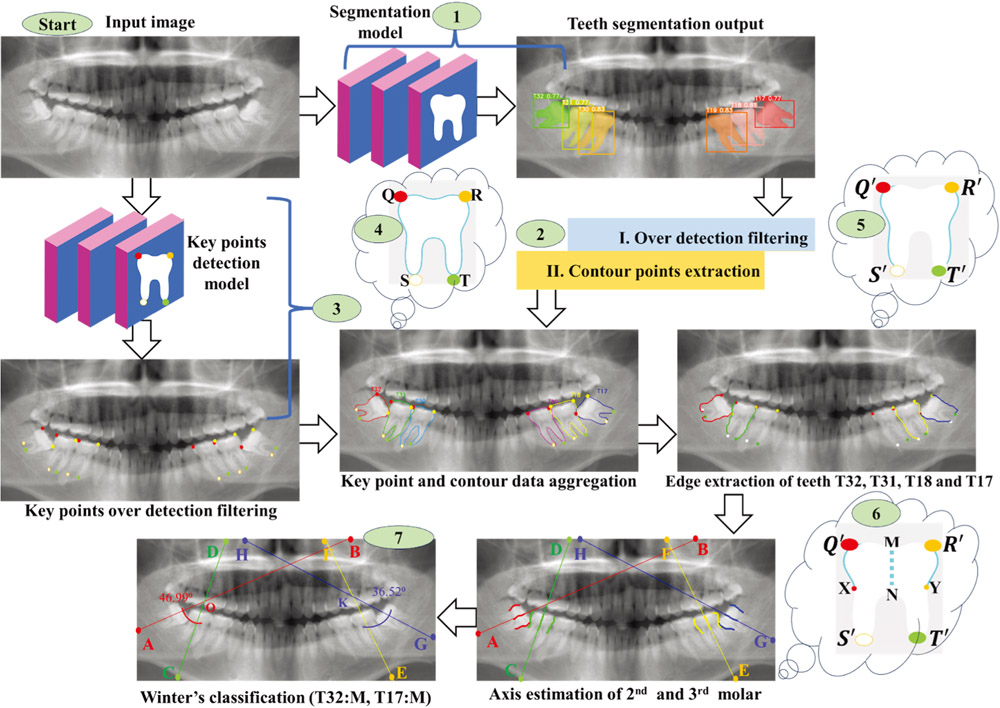
Block diagram of the proposed method
- [1] E. AteşYı ldı rım, N. Türker, D. Göller Bulut, and G. Ustaoğlu, “The relationship of the position of mandibular third molar impaction with the development of dental and periodontal lesions in adjacent second molars,” J. of Stomatology, Oral and Maxillofacial Surgery, Vol.125, No.1, Article No.101610, 2024. https://doi.org/10.1016/j.jormas.2023.101610
- [2] A. K. Demyati et al., “Assessment of the relationship between impacted mandibular third molars’ angulation pattern and crowding severity: A cross-sectional study,” The Saudi Dental J., Vol.36, No.5, pp. 789-794, 2024. https://doi.org/10.1016/j.sdentj.2024.02.008
- [3] J. F. Choi and P. Chang, “Oral surgery, extraction of unerupted teeth,” PubMed, 2024. https://www.ncbi.nlm.nih.gov/books/NBK592391/ [Accessed February 21, 2025]
- [4] Y.-H. Jung and B.-H. Cho, “Radiographic evaluation of third molar development in 6- to 24-year-olds,” Imaging Science in Dentistry, Vol.44, No.3, pp. 185-191, 2014. https://doi.org/10.5624/isd.2014.44.3.185
- [5] M. K. Sujon, M. K. Alam, S. A. Rahman, and S. N. F. Mohd Noor, “Third molar impactions prevalence and pattern among adults using 5923 digital orthopantomogram,” Bangladesh J. of Medical Science, Vol.21, No.3, pp. 717-729, 2022. https://doi.org/10.3329/bjms.v21i3.59590
- [6] M. Alsadat-Hashemipour, M. Tahmasbi-Arashlow, and F. Fahimi-Hanzaei, “Incidence of impacted mandibular and maxillary third molars-a radiographic study in a Southeast Iran population,” Medicina Oral Patología Oraly Cirugia Bucal, pp. e140-e145, 2013. https://doi.org/10.4317/medoral.18028
- [7] P. Santosh, “Impacted mandibular third molars: Review of literature and a proposal of a combined clinical and radiological classification,” Annals of Medical and Health Sciences Research, Vol.5, No.4, pp. 229-234, 2015. https://doi.org/10.4103/2141-9248.160177
- [8] K. Alesia and H. S. Khalil, “Reasons for and patterns relating to the extraction of permanent teeth in a subset of the Saudi population,” Clinical, Cosmetic and Investigational Dentistry, pp. 51-56, 2013. https://doi.org/10.2147/ccide.s49403
- [9] A. N. Faadiya, R. Widyaningrum, P. K. Arindra, and S. F. Diba, “The diagnostic performance of impacted third molars in the mandible: A review of deep learning on panoramic radiographs,” The Saudi Dental J., Vol.36, No.3, pp. 404-412, 2024. https://doi.org/j.sdentj.2023.11.025
- [10] F. G. Repass, “The impacted mandibular third molar,” The J. of the American Dental Association, Vol.28, No.9, pp. 1409-1415, 1941. https://doi.org/10.14219/jada.archive.1941.0242
- [11] G. J. Pell and B. T. Gregory, “Impacted mandibular third molars: Classification and modified techniques for removal,” Dent Digest, Vol.39, pp. 330-338, 1933. https://www.scienceopen.com/document?vid=faf9b57f-bd09-44f6-88e3-b9ac4e34859e
- [12] L. H. Matzen and A. Wenzel, “Efficacy of CBCT for assessment of impacted mandibular third molars: A review – based on a hierarchical model of evidence,” Dentomaxillofacial Radiology, Vol.44, No.1, Article No.20140189, 2015. https://doi.org/10.1259/dmfr.20140189
- [13] A. Jaroń, E. Gabrysz-Trybek, J. Bladowska, and G. Trybek, “Correlation of panoramic radiography, cone-beam computed tomography, and three-dimensional printing in the assessment of the spatial location of impacted mandibular third molars,” J. of Clinical Medicine, Vol.10, No.18, Article No.4189, 2021. https://doi.org/10.3390/jcm10184189
- [14] G. Krishnan et al., “Artificial intelligence in clinical medicine: catalyzing a sustainable global healthcare paradigm,” Frontiers in artificial intelligence, Vol.6, No.6, Article No.1227091, 2023. https://doi.org/10.3389/frai.2023.1227091
- [15] O. H. Milani et al., “A fully automated classification of third molar development stages using deep learning,” Scientific Reports, Vol.14, No.1, Article No.13082, 2024. https://doi.org/10.1038/s41598-024-63744-y
- [16] D. Torul, H. Akpinar, I. S. Bayrakdar, O. Celik, and K. Orhan, “Prediction of extraction difficulty for impacted maxillary third molars with deep learning approach,” J. of Stomatology, Oral and Maxillofacial Surgery, Vol.125, No.4, Article No.101817, 2024. https://doi.org/10.1016/j.jormas.2024.101817
- [17] J.-H. Yoo et al., “Deep learning based prediction of extraction difficulty for mandibular third molars,” Scientific Reports, Vol.11, No.1, Article No.1954, 2021. https://doi.org/10.1038/s41598-021-81449-4
- [18] J. Lee, J. Park, S. Y. Moon, and K. Lee, “Automated prediction of extraction difficulty and inferior alveolar nerve injury for mandibular third molar using a deep neural network,” Applied Sciences, Vol.12, No.1, Article No.475, 2022. https://doi.org/10.3390/app12010475
- [19] V. Trachoo, U. Taetragool, P. Pianchoopat, C. Sukitporn-udom, N. Morakrant, and K. Warin, “Deep learning for predicting the difficulty level of removing the impacted mandibular third molar,” Int. Dental J., Vol.75, No.1, pp. 144-150, 2024. https://doi.org/10.1016/j.identj.2024.06.021
- [20] F. P. Mahdi, K. Motoki, and S. Kobashi, “Optimization technique combined with deep learning method for teeth recognition in dental panoramic radiographs,” Scientific Reports, Vol.10, No.1, Article No.19261, 2020. https://doi.org/10.1038/s41598-020-75887-9
- [21] M. Ali, D. Fujita, and S. Kobashi, “Teeth and prostheses detection in dental panoramic X-rays using CNN-based object detector and a priori knowledge-based algorithm,” Scientific Reports, Vol.13, No.1, Article No.16542, 2023. https://doi.org/10.1038/s41598-023-43591-z
- [22] D. Kwon, J. Ahn, C.-S. Kim, D. O. Kang, and J.-Y. Paeng, “A deep learning model based on concatenation approach to predict the time to extract a mandibular third molar tooth,” BMC oral health, Vol.22, No.1, Article No.571, 2022. https://doi.org/10.1186/s12903-022-02614-3
- [23] S.-Y. Chun et al., “Automatic classification of 3D positional relationship between mandibular third molar and inferior alveolar canal using a distance-aware network,” BMC Oral Health, Vol.23, No.1, Article No.794, 2023. https://doi.org/10.1186/s12903-023-03496-9
- [24] K. J. Jeon, H. Choi, C. Lee, and S.-S. Han, “Automatic diagnosis of true proximity between the mandibular canal and the third molar on panoramic radiographs using deep learning,” Scientific Reports, Vol.13, No.1, Article No.22022, 2023. https://doi.org/10.1038/s41598-023-49512-4
- [25] E. Choi et al., “Artificial intelligence in positioning between mandibular third molar and inferior alveolar nerve on panoramic radiography,” Scientific Reports, Vol.12, No.1, Article No.2456, 2022. https://doi.org/10.1038/s41598-022-06483-2
- [26] S. Vinayahalingam, T. Xi, S. Bergé, T. Maal, and G. de Jong, “Automated detection of third molars and mandibular nerve by deep learning,” Scientific Reports, Vol.9, No.1, Article No.9007, 2019. https://doi.org/10.1038/s41598-019-45487-3
- [27] C. Kuwada et al., “Deep learning systems for detecting and classifying the presence of impacted supernumerary teeth in the maxillary incisor region on panoramic radiographs,” Oral Surgery, Oral Medicine, Oral Pathology and Oral Radiology, Vol.130, No.4, pp. 464-469, 2020. https://doi.org/10.1016/j.oooo.2020.04.813
- [28] M. Aljabri et al., “Canine impaction classification from panoramic dental radiographic images using deep learning models,” Informatics in Medicine Unlocked, Vol.30, Article No.100918, 2022. https://doi.org/10.1016/j.imu.2022.100918
- [29] K. J. Jeon, E.-G. Ha, H. Choi, C. Lee, and S. Han, “Performance comparison of three deep learning models for impacted mesiodens detection on periapical radiographs,” Scientific Reports, Vol.12, No.1, Article No.15402, 2022. https://doi.org/10.1038/s41598-022-19753-w
- [30] M. E. Celik, “Deep learning based detection tool for impacted mandibular third molar teeth,” Diagnostics, Vol.12, No.4, Article No.942, 2022. https://doi.org/10.3390/diagnostics12040942
- [31] T. Zirek, M. Ü. Öziç, and M. Tassoker, “AI-driven localization of all impacted teeth and prediction of winter angulation for third molars on panoramic radiographs: Clinical user interface design,” Computers in Biology and Medicine, Vol.178, pp. 108755-108755, 2024. https://doi.org/10.1016/j.compbiomed.2024.108755
- [32] M. Vranckx et al., “Artificial intelligence (AI)-driven molar angulation measurements to predict third molar eruption on panoramic radiographs,” Int. J. of Environmental Research and Public Health, Vol.17, No.10, Article No.3716, 2020. https://doi.org/10.3390/ijerph17103716
- [33] N. Kourehpaz et al., “Comparison of consequences of operculectomy using conventional surgical knife and laser: A systematic review and meta-analysis,” Crescent J. of Medical and Biological Sciences, Vol.12, No.1, pp. 13-20, 2024. https://doi.org/10.34172/cjmb.2024.3009
- [34] S. Yilmaz, M. Z. Adisen, M. Misirlioglu, and S. Yorubulut, “Assessment of third molar impaction pattern and associated clinical symptoms in a Central Anatolian Turkish population,” Medical Principles and Practice, Vol.25, No.2, pp. 169-175, 2015. https://doi.org/10.1159/000442416
- [35] T. Di Giovanni, T. Vogiatzi, V. Koretsi, T. Walsh, N. Silikas, and S. N. Papageorgiou, “Effect of orthodontic extraction of mandibular premolars on third molar angulation after treatment with fixed appliances,” J. Orofac. Orthop., Vol.85, pp. 392-403, 2023. https://doi.org/10.1007/s00056-023-00465-3
- [36] C.-Y. Wang, A. Bochkovskiy, and H.-Y. M. Liao, “YOLOv7: Trainable bag-of-freebies sets new state-of-the-art for real-time object detectors,” arXiv preprint, arXiv:2207.02696, 2022. https://doi.org/10.48550/arxiv.2207.02696
- [37] G. Jocher, A. Chaurasia, and J. Qiu, “YOLOv8 by ultralytics,” GitHub, Jan. 01, 2023. https://github.com/ultralytics/ultralytics [Accessed February 21, 2025]
- [38] D. Müller, D. Hartmann, P. Meyer, F. Auer, I. Soto-Rey, and F. Kramer, “MISeval: A metric library for medical image segmentation evaluation,” Studies in health technology and informatics, Vol.294, pp. 33-37, 2022. https://doi.org/10.3233/shti220391
This article is published under a Creative Commons Attribution-NoDerivatives 4.0 Internationa License.