Research Paper:
Revisiting the Evaluation for Chinese Grammatical Error Correction
Hongfei Wang*
, Zhousi Chen*
, Zizheng Zhang*, Zhidong Ling*, Xiaomeng Pan*, Wenjie Duan*, Masato Mita*,**
, and Mamoru Komachi***

*Tokyo Metropolitan University
6-6 Asahigaoka, Hino, Tokyo 191-0065, Japan
**CyberAgent, Inc.
2-24-12 Shibuya, Shibuya-ku, Tokyo 150, Japan
***Hitotsubashi University
2-1 Naka, Kunitachi, Tokyo 186-8601, Japan
English grammar error correction (GEC) has been a popular topic over the past decade. The appropriateness of automatic evaluations, e.g., the combination of metrics and reference types, has been thoroughly studied for English GEC. Yet, such systematic investigations on the Chinese GEC are still insufficient. Specifically, we noticed that two representative Chinese GEC evaluation datasets, namely YACLC and MuCGEC, adopt fluency edits-based references with the automatic evaluation metric, which was designed for minimal edits-based references and differs from the convention of English GEC. However, it is unclear whether such evaluation settings are appropriate. Furthermore, we explored other dimensions of Chinese GEC evaluation, such as the number of references and tokenization granularity, and found that the two datasets exhibit significant differences. We hypothesize that these differences are crucial for Chinese GEC automatic evaluation. Thus, we publish the first human-annotated rankings on Chinese GEC system outputs and conducted an analytical meta-evaluation which discovered that 1) automatic evaluation metrics should match the types of reference; 2) the evaluation performance grows with the number of references, a consistent finding with English GEC, while four is the smallest reference number that empirically shows maximum correlation with human annotators; and 3) the granularity of tokenization has a minor impact, which is however a necessary preprocessing step for Chinese texts. We have made the proposed dataset publicly accessible at https://github.com/wang136906578/RevisitCGEC.
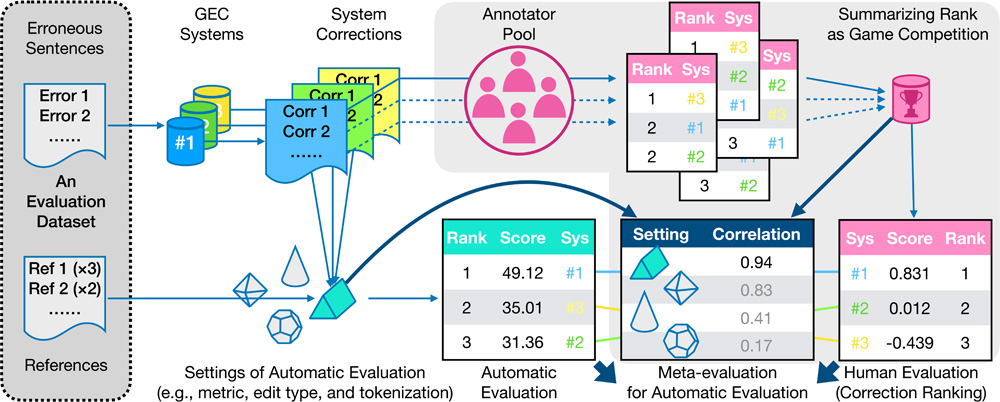
Meta-evaluation for Chinese GEC
- [1] H. Yannakoudakis, T. Briscoe, and B. Medlock, “A New Dataset and Method for Automatically Grading ESOL Texts,” Proc. of the 49th Annual Meeting of the Association for Computational Linguistics: Human Language Technologies, pp. 180-189, 2011.
- [2] H. T. Ng, S. M. Wu, T. Briscoe, C. Hadiwinoto, R. H. Susanto, and C. Bryant, “The CoNLL-2014 Shared Task on Grammatical Error Correction,” Proc. of the 18th Conf. on Computational Natural Language Learning: Shared Task, pp. 1-14, 2014. https://doi.org/10.3115/v1/W14-1701
- [3] C. Napoles, K. Sakaguchi, and J. Tetreault, “JFLEG: A Fluency Corpus and Benchmark for Grammatical Error Correction,” Proc. of the 15th Conf. of the European Chapter of the Association for Computational Linguistics: Vol.2, Short Papers, pp. 229-234, 2017.
- [4] C. Bryant, M. Felice, Ø. E. Andersen, and T. Briscoe, “The BEA-2019 Shared Task on Grammatical Error Correction,” Proc. of the 14th Workshop on Innovative Use of NLP for Building Educational Applications, pp. 52-75, 2019. https://doi.org/10.18653/v1/W19-4406
- [5] K. Sakaguchi, C. Napoles, M. Post, and J. Tetreault, “Reassessing the Goals of Grammatical Error Correction: Fluency Instead of Grammaticality,” Trans. of the Association for Computational Linguistics, Vol.4, pp. 169-182, 2016. https://doi.org/10.1162/tacl_a_00091
- [6] C. Bryant and H. T. Ng, “How Far are We from Fully Automatic High Quality Grammatical Error Correction?,” Proc. of the 53rd Annual Meeting of the Association for Computational Linguistics and the 7th Int. Joint Conf. on Natural Language Processing (Vol.1: Long Papers), pp. 697-707, 2015. https://doi.org/10.3115/v1/P15-1068
- [7] R. Grundkiewicz, M. Junczys-Dowmunt, and E. Gillian, “Human Evaluation of Grammatical Error Correction Systems,” Proc. of the 2015 Conf. on Empirical Methods in Natural Language Processing, pp. 461-470, 2015.
- [8] S. Chollampatt and H. T. Ng, “A Reassessment of Reference-Based Grammatical Error Correction Metrics,” Proc. of the 27th Int. Conf. on Computational Linguistics, pp. 2730-2741, 2018.
- [9] L. Choshen and O. Abend, “Inherent Biases in Reference-based Evaluation for Grammatical Error Correction,” Proc. of the 56th Annual Meeting of the Association for Computational Linguistics (Vol.1, Long Papers), pp. 632-642, 2018. https://doi.org/10.18653/v1/P18-1059
- [10] P. Gong, X. Liu, H. Huang, and M. Zhang, “Revisiting Grammatical Error Correction Evaluation and Beyond,” Proc. of the 2022 Conf. on Empirical Methods in Natural Language Processing, pp. 6891-6902, 2022. https://doi.org/10.18653/v1/2022.emnlp-main.463
- [11] Y. Zhao, N. Jiang, W. Sun, and X. Wan, “Overview of the NLPCC 2018 Shared Task: Grammatical Error Correction,” Natural Language Processing and Chinese Computing, pp. 439-445, 2018. https://doi.org/10.1007/978-3-319-99501-4_41
- [12] Y. Wang, C. Kong, L. Yang, Y. Wang, X. Lu, R. Hu, S. He, Z. Liu, Y. Chen, E. Yang, and M. Sun, “YACLC: A Chinese Learner Corpus with Multidimensional Annotation,” arXiv preprint, arXiv:2112.15043v1, 2021. https://doi.org/10.48550/arXiv.2112.15043
- [13] Y. Zhang, Z. Li, Z. Bao, J. Li, B. Zhang, C. Li, F. Huang, and M. Zhang, “MuCGEC: A Multi-Reference Multi-Source Evaluation Dataset for Chinese Grammatical Error Correction,” Proc. of the 2022 Conf. of the North American Chapter of the Association for Computational Linguistics: Human Language Technologies, pp. 3118-3130, 2022. https://doi.org/10.18653/v1/2022.naacl-main.227
- [14] D. Dahlmeier and H. T. Ng, “Better Evaluation for Grammatical Error Correction,” Proc. of the 2012 Conf. of the North American Chapter of the Association for Computational Linguistics: Human Language Technologies, pp. 568-572, 2012.
- [15] S. Ma, Y. Li, R. Sun, Q. Zhou, S. Huang, D. Zhang, L. Yangning, R. Liu, Z. Li, Y. Cao, H. Zheng, and Y. Shen, “Linguistic Rules-Based Corpus Generation for Native Chinese Grammatical Error Correction,” Findings of the Association for Computational Linguistics (EMNLP 2022), pp. 576-589, 2022. https://doi.org/10.18653/v1/2022.findings-emnlp.40
- [16] L. Xu, J. Wu, J. Peng, J. Fu, and M. Cai, “FCGEC: Fine-Grained Corpus for Chinese Grammatical Error Correction,” Y. Goldberg, Z. Kozareva, and Y. Zhang (Eds.), Findings of the Association for Computational Linguistics (EMNLP 2022), pp. 1900-1918, 2022. https://doi.org/10.18653/v1/2022.findings-emnlp.137
- [17] R. Nagata and K. Sakaguchi, “Phrase Structure Annotation and Parsing for Learner English,” Proc. of the 54th Annual Meeting of the Association for Computational Linguistics (Vol.1: Long Papers), pp. 1837-1847, 2016. https://doi.org/10.18653/v1/P16-1173
- [18] G. Rao, Q. Gong, B. Zhang, and E. Xun, “Overview of NLPTEA-2018 Share Task Chinese Grammatical Error Diagnosis,” Proc. of the 5th Workshop on Natural Language Processing Techniques for Educational Applications, pp. 42-51, 2018. https://doi.org/10.18653/v1/W18-3706
- [19] G. Rao, E. Yang, and B. Zhang, “Overview of NLPTEA-2020 Shared Task for Chinese Grammatical Error Diagnosis,” Proc. of the 6th Workshop on Natural Language Processing Techniques for Educational Applications, pp. 25-35, 2020.
- [20] Y. Li, S. Qin, J. Ye, S. Ma, Y. Li, L. Qin, X. Hu, W. Jiang, H.-T. Zheng, and P. S. Yu, “Rethinking the Roles of Large Language Models in Chinese Grammatical Error Correction,” arXiv preprint, arXiv:2402.11420v2, 2024. https://doi.org/10.48550/arXiv.2402.11420
- [21] Y. Shao, Z. Geng, Y. Liu, J. Dai, F. Yang, L. Zhe, H. Bao, and X. Qiu, “CPT: A Pre-Trained Unbalanced Transformer for Both Chinese Language Understanding and Generation,” arXiv preprint, arXiv:2109.05729v4, 2021. https://doi.org/10.48550/arXiv.2109.05729
- [22] Y. Wang, C. Kong, X. Liu, X. Fang, Y. Zhang, N. Liang, T. Zhou, T. Liao, L. Yang, Z. Li, G. Rao, Z. Liu, C. Li, E. Yang, M. Zhang, and M. Sun, “Overview of CLTC 2022 Shared Task: Chinese Learner Text Correction,” Proc. of the 21st China National Conf. on Computational Linguistics (CCL 2022), 2022.
- [23] K. Omelianchuk, V. Atrasevych, A. Chernodub, and O. Skurzhanskyi, “GECToR – Grammatical Error Correction: Tag, Not Rewrite,” Proc. of the 15th Workshop on Innovative Use of NLP for Building Educational Applications, pp. 163-170, 2020.
- [24] J. Su, “Chinese T5 PEGASUS,” Technical report, ZhuiyiAI, 2021.
- [25] J. Zhang, Y. Zhao, M. Saleh, and P. J. Liu, “PEGASUS: Pre-training with Extracted Gap-sentences for Abstractive Summarization,” 37th Int. Conf. on Machine Learning, Article No.1051, 2020.
- [26] H. Wang, M. Kurosawa, S. Katsumata, M. Mita, and M. Komachi, “Chinese Grammatical Error Correction Using Pre-Trained Models and Pseudo Data,” ACM Trans. Asian Low-Resour. Lang. Inf. Process., Vol.22, Issue 3, Article No.89, 2023. https://doi.org/10.1145/3570209
- [27] A. Vaswani, N. Shazeer, N. Parmar, J. Uszkoreit, L. Jones, A. N. Gomez, L. Kaiser, and I. Polosukhin, “Attention is All you Need,” Advances in Neural Information Processing Systems, pp. 6000-6010, 2017.
- [28] T. Kudo and J. Richardson, “SentencePiece: A simple and language independent subword tokenizer and detokenizer for neural text processing,” Proc. of the 2018 Conf. on Empirical Methods in Natural Language Processing: System Demonstrations, pp. 66-71, 2018.
- [29] X. Liang, Z. Zhou, H. Huang, S. Wu, T. Xiao, M. Yang, Z. Li, and C. Bian. “Character, Word, or Both? Revisiting the Segmentation Granularity for Chinese Pre-trained Language Models,” 2023.
- [30] C. Federmann, “Appraise: An Open-Source Toolkit for Manual Phrase-Based Evaluation of Translations,” Proc. of 17th Int. Conf. on Language Resources and Evaluation, 2010.
- [31] J. Cohen, “A Coefficient of Agreement for Nominal Scales,” Educational and Psychological Measurement, Vol.20, Issue 1, pp. 37-46, 1960. https://doi.org/10.1177/001316446002000104
- [32] R. Herbrich, T. Minka, and T. Graepel, “TrueSkill™ : A Bayesian Skill Rating System,” Advances in Neural Information Processing Systems, 2006.
- [33] C. Bryant, M. Felice, and T. Briscoe, “Automatic Annotation and Evaluation of Error Types for Grammatical Error Correction,” Proc. of the 55th Annual Meeting of the Association for Computational Linguistics (Vol.1: Long Papers), pp. 793-805, 2017. https://doi.org/10.18653/v1/P17-1074
This article is published under a Creative Commons Attribution-NoDerivatives 4.0 Internationa License.