Research Paper:
License Plate Recognition Using Three-Dimensional Rotated Character Recognition and Instance Segmentation by Deep Learning
Tetsuro Sasaki, Kento Morita
, and Tetsushi Wakabayashi
Graduate School of Engineering, Mie University
1577 Kurimamachiya-cho, Tsu, Mie 514-8507, Japan
Corresponding author
License plate recognition is currently used in various situations, such as parking lot vehicle management and the tracking of wanted vehicles. These applications require recognition from all possible camera angles. Therefore, we propose a method for license plate recognition in images captured from various camera angles. First, the license plate area is detected from the input image using the YOLOv5 object detection method. The subsequent process can be performed in two manners: by rotating the license plate to the front using projective transformation and trapezoidal correction, or by using graph matching. The first method performs Hough transforms or linear approximations as preprocessing, and then calculates the rectangle surrounding the license plate from the YOLOv5 output. After performing trapezoidal correction, the maximally stable extremal regions (MSER) are used to detect character candidates, and characters are recognized using 3D rotated character recognition. The second method performs character candidate detection and recognition without trapezoidal correction, followed by graph matching. These methods are versatile because they do not depend on the layout of license plates, which varies among countries and regions. Two types of datasets were used: a set of images containing Japanese license plates collected by ourselves, and a set of publicly available images containing Taiwanese license plates. Two recognition rates were evaluated: the license plate character recognition rate and license plate recognition rate in which all characters in one plate are recognized. The license plate character recognition rates using graph matching were 91.9% for the Japanese license plates and 89.3% for the Taiwanese license plates. The license plate recognition rates were 84.0% and 84.5%, respectively.
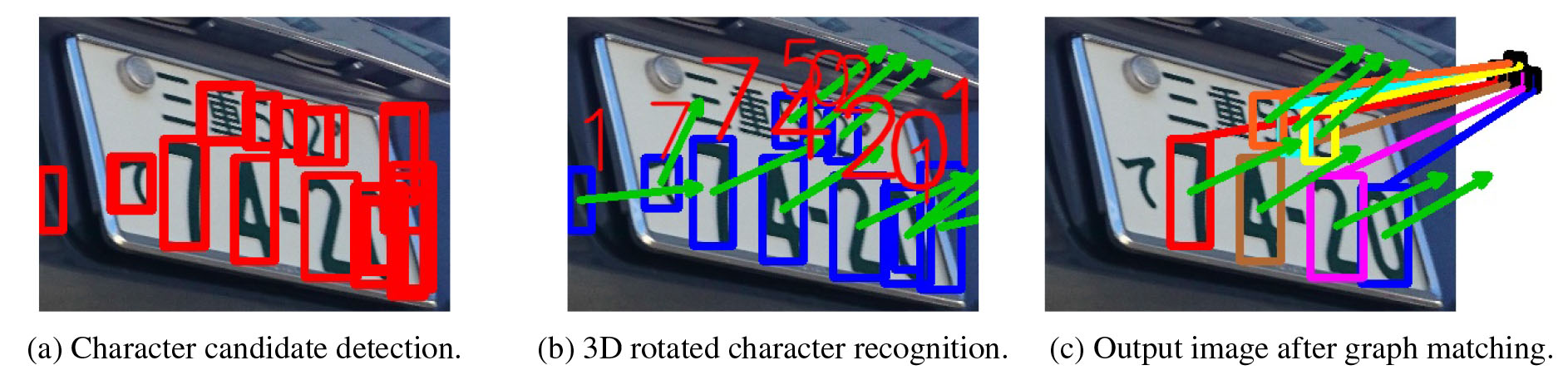
Output images following license plate detection
- [1] S.-L. Chang, L.-S. Chen, Y.-C. Chung, and S.-W. Chen, “Automatic license plate recognition,” IEEE Trans. on Intelligent Transportation Systems, Vol.5, No.1, pp. 42-53, 2004. https://doi.org/10.1109/TITS.2004.825086
- [2] G.-W. Chen, C.-M. Yang, and T.-U. İk, “Real-Time License Plate Recognition and Vehicle Tracking System Based on Deep Learning,” 2021 22nd Asia-Pacific Network Operations and Management Symp. (APNOMS), pp. 378-381, 2021. https://doi.org/10.23919/APNOMS52696.2021.9562691
- [3] Y. Kessentini, M. D. Besbes, S. Ammar, and A. Chabbouh, “A two-stage deep neural network for multi-norm license plate detection and recognition,” Expert Systems with Applications, Vol.136, pp. 159-170, 2019. https://doi.org/10.1016/j.eswa.2019.06.036
- [4] W. Wang and J. Tu, “Research on License Plate Recognition Algorithms Based on Deep Learning in Complex Environment,” IEEE Access, Vol.8, pp. 91661-91675, 2020. https://doi.org/10.1109/ACCESS.2020.2994287
- [5] J. Shashirangana, H. Padmasiri D. Meedeniya, and C. Perera, “Automated license plate recognition: a survey on methods and techniques,” IEEE Access, Vol.9, pp. 11203-11225, 2020. https://doi.org/10.1109/ACCESS.2020.3047929
- [6] I. V. Pustokhina, D. A. Pustokhin, J. J. Rodrigues, D. Gupta, A. Khanna, K. Shankar, C. Seo, and G. P. Joshi, “Automatic vehicle license plate recognition using optimal K-means with convolutional neural network for intelligent transportation systems,” IEEE Access, Vol.8, pp. 92907-92917, 2020. https://doi.org/10.1109/ACCESS.2020.2993008
- [7] S. Raj, Y. Gupta, and R. Malhotra, “License plate recognition system using yolov5 and cnn,” 2022 8th Int. Conf. on Advanced Computing and Communication Systems (ICACCS), Vol.1, pp. 372-377, 2022. https://doi.org/10.1109/ICACCS54159.2022.9784966
- [8] C. Henry, S. Y. Ahn, and S. W. Lee, “Multinational license plate recognition using generalized character sequence detection,” IEEE Access, Vol.8, pp. 35185-35199, 2020. https://doi.org/10.1109/ACCESS.2020.2974973
- [9] S. M. Silva and C. R. Jung, “A flexible approach for automatic license plate recognition in unconstrained scenarios,” IEEE Trans. on Intelligent Transportation Systems, Vol.23, No.6, pp. 5693-5703, 2021. https://doi.org/10.1109/TITS.2021.3055946
- [10] S. Luo and J. Liu, “Research on car license plate recognition based on improved YOLOv5m and LPRNet,” IEEE Access, Vol.10, pp. 93692-93700, 2022. https://doi.org/10.1109/ACCESS.2022.3203388
- [11] Y. Nakamura, W. Ohyama, T. Wakabayashi, and F. Kimura, “License-Plate Recognition via 3 Dimensional OCR,” Proc. of 3rd ICET: 2nd Int. Conf. on Information Technology (ICIT2016), pp. 33-37, 2016.
- [12] G. Jocher et al., “ultralytics/yolov5: v7.0 - YOLOv5 SOTA Realtime Instance Segmentation,” Zenodo, 2022. https://doi.org/10.5281/zenodo.7347926
- [13] D. H. Ballard, “Generalizing the Hough transform to detect arbitrary shapes,” Pattern Recognition, Vol.13, No.2, pp. 111-122, 1981. https://doi.org/10.1016/0031-3203(81)90009-1
- [14] J. Sklansky and V. Gonzalez, “Fast polygonal approximation of digitized curves,” Pattern Recognition, Vol.12, No.5, pp. 327-331, 1980. https://doi.org/10.1016/0031-3203(80)90031-X
- [15] J. Matas, O. Chum, M. Urban, and T. Pajdla, “Robust wide-baseline stereo from maximally stable extremal regions,” Image and Vision Computing, Vol.22, No.10, pp. 761-767, 2004. https://doi.org/10.1016/j.imavis.2004.02.006
- [16] R. Narita, W. Ohyama, T. Wakabayashi, and F. Kimura, “A Study on Three Dimensional Rotation-Free Character Recognition and Rotation Angle Estimation of Characters,” Proc. of the 21st Int. Conf. on Pattern Recognition, pp. 677-680, 2012.
- [17] G.-S. Hsu, J.-C. Chen, and Y.-Z. Chung, “Application-oriented license plate recognition,” IEEE Trans. on Vehicular Technology, Vol.62, No.2, pp. 552-561, 2013. https://doi.org/10.1109/TVT.2012.2226218
- [18] T. Sasaki, K. Morita, and T. Wakabayashi, “License plate recognition using 3D rotated character recognition and deep learning,” 2022 Joint 12th Int. Conf. on Soft Computing and Intelligent Systems and 23rd Int. Symp. on Advanced Intelligent Systems (SCIS&ISIS), pp. 1-6, 2022. https://doi.org/10.1109/SCISISIS55246.2022.10002128
- [19] S. T. Wu and M. R. G. Marquez, “A non-self-intersection Douglas-Peucker algorithm,” 16th Brazilian Symp. on Computer Graphics and Image Processing (SIBGRAPI 2003), pp. 60-66, 2003. https://doi.org/10.1109/SIBGRA.2003.1240992
This article is published under a Creative Commons Attribution-NoDerivatives 4.0 Internationa License.