Research Paper:
A Study of Library Mobile Robot Book Classification and Transportation by Integrating DA and RMM
Dongli Zhang
Jiaozuo Teachers College
Jiaozuo , China
Corresponding author
As the complexity of modern library management tasks increases, it is difficult for traditional mobile robots to meet the task of moving and classifying books. In order to design a mobile robot that can autonomously classify and transport books, the study realizes the tasks of book classification and transportation in libraries by fusing the differential speed algorithm and the robot motion model algorithm. First, the robot operating system is utilized to scan the books, classify the books, and obtain the category information of the books. Then, the differential speed algorithm is used to control the motion of the robot to ensure that the robot can accurately transport the books to the designated location. At the same time, combined with the robot motion model algorithm, the motion trajectory of the robot is planned to ensure that the robot can avoid obstacles and stably complete the book transportation task. Finally, the deep reinforcement learning algorithm is used to train the decision-making model of the robot to improve the intelligence level of the robot. The results of simulation experiments show that the research method has the highest accuracy, with an average accuracy of 99.98%, and the robot is able to accurately categorize the books and quickly avoid obstacles with strong stability. The results of the application experiments show that the research method has the shortest moving distance, with an average moving distance of 132 m and an average completion time of 34 seconds, which are lower than the remaining three types of robots. The research robot showed high accuracy in the task of returning books in four time periods within 10 days in the library, with an average accuracy of 99.58%. The experimental results validate the superiority of the research methodology and show that the robots are capable of accurately recognizing and classifying books and can autonomously perform transportation tasks in libraries. The research results help to improve the automation level and management efficiency of libraries and have important application value.
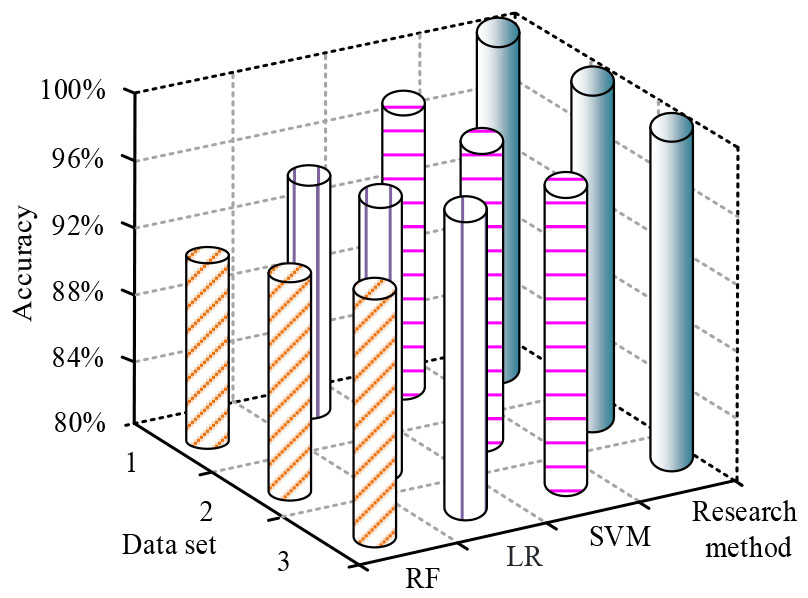
The accuracy of book return under different methods
- [1] Y. Fang, B. Luo, and T. Zhao, “ST-SIGMA: Spatio-temporal semantics and interaction graph aggregation for multi-agent perception and trajectory forecasting,” CAAI Trans. on Intelligence Technology, Vol.7, No.4, pp. 744-757, 2022. https://doi.org/10.1049/cit2.12145
- [2] A. T. Knowles, A. E. Sansom, and C. A. Prieto, “sMILES: A library of semi-empirical MILES stellar spectra with variable abundances,” Monthly Notices of the Royal Astronomical Society, Vol.523, No.3, pp. 3450-3470, 2021. https://doi.org/10.1093/mnras/stab1001
- [3] R. K. Pai, D. Hartman, and D. F. Schaeffer, “Development and initial validation of a deep learning algorithm to quantify histologic features in colorectal carcinoma including tumour budding / poorly differentiated clusters,” Histopathology, Vol.79, No.3, pp. 391-405, 2021. https://doi.org/10.1111/his.14353
- [4] F. Keynia and G. Memarzadeh, “A new financial loss / gain wind power forecasting method based on deep machine learning algorithm by using energy storage system,” IET Generation, Transmission & Distribution, Vol.16, No.5, pp. 851-868, 2021. https://doi.org/10.1049/gtd2.12332
- [5] A. Asemi, A. Ko, and M. Nowkarizi, “Intelligent libraries: A review on expert systems, artificial intelligence, and robot,” Library Hi Tech, Vol.39, No.2, pp. 412-434, 2020. http://creativecommons.org/licences/by/4.0/legalcode
- [6] S. M. Shafaei and H. Mousazadeh, “Development of a mobile robot for safe mechanical evacuation of hazardous bulk materials in industrial confined spaces,” J. of Field Robotics, Vol.39, No.3, pp. 218-231, 2021. https://doi.org/10.1002/rob.22047
- [7] H. Yang, S. Wang, Z. Zuo, and P. Li, “Trajectory tracking for a wheeled mobile robot with an omnidirectional wheel on uneven ground,” IET Control Theory & Amp; Applications, Vol.14, No.7, pp. 921-929, 2020. https://doi.org/10.1049/iet-cta.2019.1074
- [8] D. H. Dos Reis, D. Welfer, M. A. De Souza Leite Cuadros, and D. Fernando Tello Gamarra, “Mobile robot navigation using an object recognition software with RGBD images and the YOLO algorithm,” Applied Artificial Intelligence, Vol.33, No.14, pp. 1290-1350, 2019. https://doi.org/10.1080/08839514.2019.1684778
- [9] M. Gheisarnejad and M. H. Khooban, “Supervised control strategy in trajectory tracking for a wheeled mobile robot,” IET Collaborative Intelligent Manufacturing, Vol.1, No.1, pp. 3-9, 2019. https://doi.org/10.1049/iet-cim.2018.0007
- [10] Y. Noh and Y. Shin, “A study on the library activation plan using autonomous objects,” J. of Korean Library and Information Science Society, Vol.52, No.1, pp. 27-54, 2021. https://doi.org/10.16981/KLISS.52.1.202103.27
- [11] C. E. Luis, M. Vukosavljev, and A. P. Schoellig, “Online trajectory generation with distributed model predictive control for multi-robot motion planning,” IEEE Robotics and Automation Letters, Vol.5, No.2, pp. 604-611, 2020. https://doi.org/10.1109/LRA.2020.2964159
- [12] D. Fridovich-Keil, A. Bajcsy, J. F. Fisac, S. L. Herbert, S. Wang, A. D. Dragan, and C. J. Tomlin, “Confidence-aware motion prediction for real-time collision avoidance1,” The Int. J. of Robotics Research, Vol.39, Nos.2-3, pp. 250-265, 2020. https://doi.org/10.1177/0278364919859
- [13] W. Deng, S. Shang, X. Cai, H. Zhao, Y. Song, and J. Xu, “An improved differential evolution algorithm and its application in optimization problem,” Soft Computing, Vol.25, No.1, pp. 5277-5298, 2021. https://doi.org/10.1007/s00500-020-05527-x
- [14] X. Xia, L. Gui, Y. Zhang, X. Xu, F. Yu, H. Wu, and K. Li, “A fitness-based adaptive differential evolution algorithm,” Information Sciences, Vol.549, pp. 16-141, 2021. https://doi.org/10.1016/j.ins.2020.11.015
- [15] W. Deng, H. Liu, J. Xu, H. Zhao, and Y. Song, “An improved quantum-inspired differential evolution algorithm for deep belief network,” IEEE Trans. on Instrumentation and Measurement, Vol.69, No.10, pp. 7319-7327, 2020. https://doi.org/10.1109/TIM.2020.2983233
- [16] H. Zhou, P. Feng, and W. Chou, “A hybrid obstacle avoidance method for mobile robot navigation in unstructured environment,” Industrial Robot: The Int. J. of Robotics Research and Application, Vol.50, No.1, pp. 94-106, 2023. https://doi.org/10.1108/IR-04-2022-0102
- [17] H. Wang, “Fuzzy control system for visual navigation of autonomous mobile robot based on Kalman filter,” Int. J. of System Assurance Engineering and Management, Vol.14, No.2, pp. 786-795, 2023. https://doi.org/10.1007/s13198-021-01570-5
- [18] S. Zhao and S. H. Hwang, “Complete coverage path planning scheme for autonomous navigation ROS-based robots,” ICT Express, Vol.10, No.1, pp. 83-89, 2024. https://doi.org/10.1016/j.icte.2023.06.009
This article is published under a Creative Commons Attribution-NoDerivatives 4.0 Internationa License.