Research Paper:
Synthesis of Comic-Style Portraits Using Combination of CycleGAN and Pix2Pix
Yen-Chia Chen*,, Hiroki Shibata*
, Lieu-Hen Chen**, and Yasufumi Takama*

*Tokyo Metropolitan University
6-6 Asahigaoka, Hino, Tokyo 191-0065, Japan
Corresponding author
**National Chi Nan University
#1 University Road, Puli, Nantao, Taiwan
This paper proposes a system for converting face photos into portraits with specific charcoal sketch-like style. NPR (non-photorealistic rendering) for converting real photo images into anime-style images have been studied. Its promising application is the creation of users’ portraits on social networks for preventing leaks of personal information. An image-to-image transformation using GAN (Generative Adversarial Network), such as CycleGAN or Pix2Pix, is expected to be used for this goal. However, it is difficult to generate portraits with specific comic styles that satisfy two conditions at the same time: preserving photorealistic features and reproducing the comic styles. For example, replacing too many photorealistic features on the face with comic style will destroy users’ identity, such as eyes shape. To solve this problem, the proposed system combines CycleGAN and Pix2Pix. CycleGAN is used to generate paired examples for Pix2Pix and Pix2Pix learns photo-to-comic transformation. To further emphasize a comic style, this paper also proposes two extensions, which divides face images into several parts such as hair and eye parts. The quality of generated images are evaluated with questionnaire by 50 answerers. The results show that the images generated by the proposed system are highly evaluated than the images generated by CycleGAN in terms of preserving the features in a photo and reproducing a comic style. Although the segmentation tend to collapse the generated images, it can reproduce a comic style effectively, especially on facial parts.
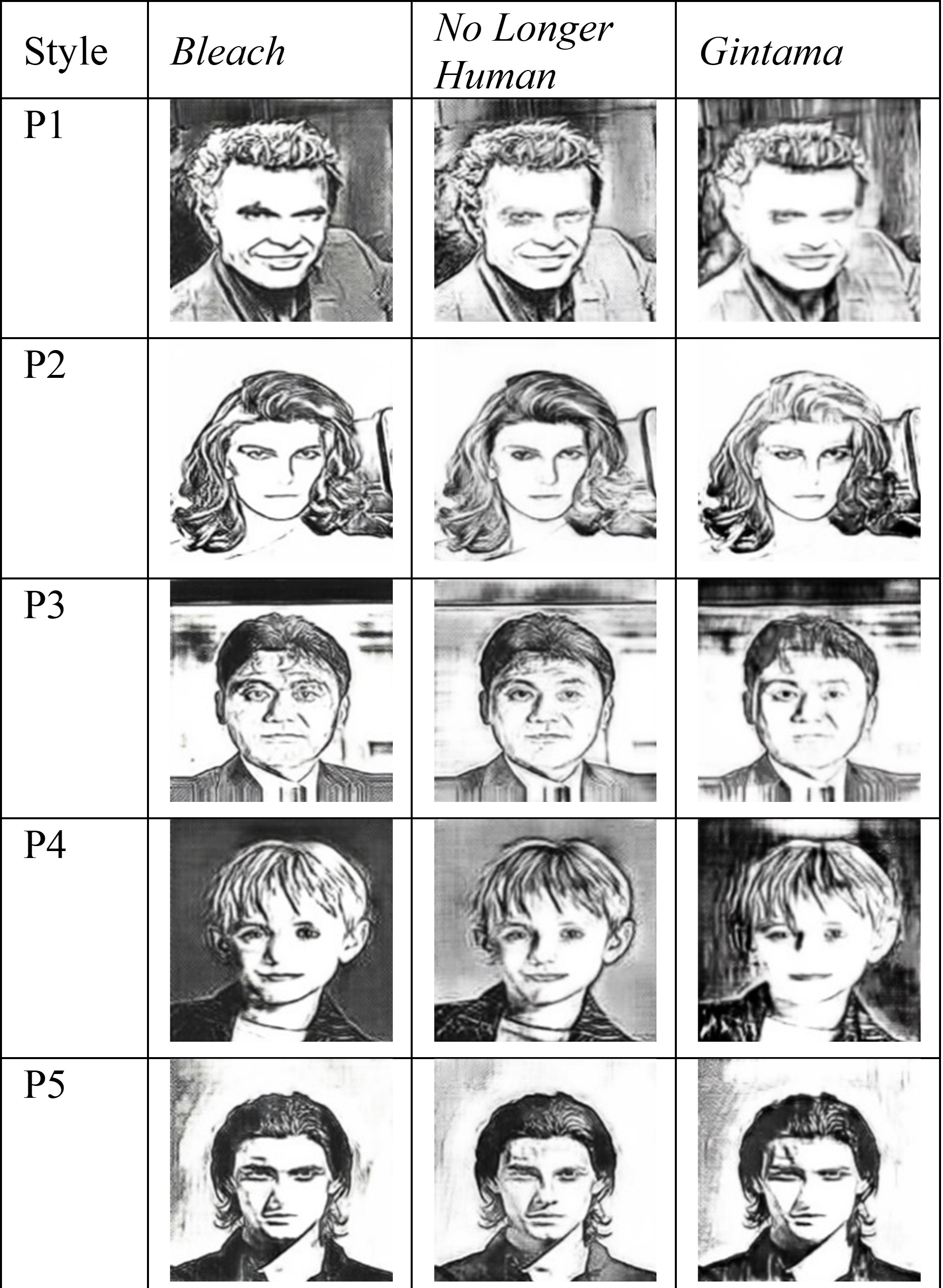
Images generated by proposed method
- [1] X. Chen, C. Xu, X. Yang, L. Song, and D. Tao, “Gated-GAN: Adversarial gated networks for multi-collection style transfer,” IEEE Trans. on Image Processing, Vol.28, No.2, pp. 546-560, 2018.
- [2] P. Isola, J. Y. Zhu, T. Zhou, and A. A. Efros, “Image-to-image translation with conditional adversarial networks,” Proc. of the IEEE Conf. on Computer Vision and Pattern Recognition, pp. 1125-1134, 2017.
- [3] J. Y. Zhu, T. Park, P. Isola, and A. A. Efros, “Unpaired image-to-image translation using cycle-consistent adversarial networks,” Proc. of the IEEE Int. Conf. on Computer Vision, pp. 2223-2232, 2017.
- [4] H. Zhang, T. Xu, H. Li, S. Zhang, X. Wang, X. Huang, and D. N. Metaxas, “StackGAN: Text to photo-realistic image synthesis with stacked generative adversarial networks,” Proc. of the IEEE Int. Conf. on Computer Vision, pp. 5907-5915, 2017.
- [5] R. Rombach, A. Blattmann, D. Lorenz, P. Esser, and B. Ommer, “High-resolution image synthesis with latent diffusion models,” Proc. of the IEEE/CVF Conf. on Computer Vision and Pattern Recognition, pp. 10684-10695, 2022.
- [6] Y. C. Chen, L. H. Chen, H. Shibata, and Y. Takama, “Styled comic portrait synthesis based on GAN,” N. Matsumura, K. Yada, M. Matsushita, D. Katagami, A. Abe, H. Kashima, T. Hiraoka, T. Uchiya, and R. Rzepka (Eds.), “Advances in Artificial Intelligence: Selected Papers From the Annual Conf. of Japanese Society of Artificial Intelligence (JSAI 2021),” Springer Nature, pp. 69-80, 2021.
- [7] H. Fukushima, Y. Uchida, and K. Araki, “Characteristic analysis of onomatopoeia that appears in comic panel images,” Proc. of the 38th Fuzzy System Symp., pp. 576-579, 2022 (in Japanese).
- [8] S. Watanabe, H. Mochizuki, K. Ninomiya, M. Kajita, and S. Nakamura, “Study on automatic clothing region extraction and impression estimation for impression-based comic search,” Proc. of 4th Conf. on Comic Computing, pp. 38-45, 2020 (in Japanese).
- [9] R. Yamashita, B. Park, and M. Matsushita, “Support for exploratory information access based on comic content information,” Trans. of the Japanese Society for Artificial Intelligence, Vol.32, No.1, WII-D_1, 2017 (in Japanese).
- [10] J. Chen, G. Liu, and X. Chen, “AnimeGAN: a novel lightweight GAN for photo animation,” Int. Symp. on Intelligence Computation and Applications, pp. 242-256, 2020.
This article is published under a Creative Commons Attribution-NoDerivatives 4.0 Internationa License.