Paper:
Characteristic Behavior of Human Multi-Joint Spatial Trajectory in Slalom Skiing
Peizhang Li, Qing Fei, Zhen Chen, Xiaolan Yao, and Yijia Zhang
School of Automation, Beijing Institute of Technology
No.5 Zhong Guan Cun South Street, Haidian District, Beijing 100081, China
Corresponding author
The scientific analysis of the slalom training process can significantly improve the performance of athletes. In this paper, the P matrix is defined by extracting the multi-joint space coordinate trajectories of the athletes in the video to analyze the slalom training pattern. The principal component analysis was used to extract the main eigenvalues and eigenvectors of the P matrix, which were defined as the main eigenbehaviors of slalom skiing, and six main eigenbehaviors were used to achieve a similarity of 96% between the reconstructed skiing sequence and the original sequence. Similarly, the group characteristic S matrix is constructed by using the individual eigenbehaviors, and the eigenvectors of the matrix are used to define the characteristic behavior of the group to classify the hierarchical group and determine the group to which the individual belongs. Results show that this method can better identify the movement pattern of the human body’s multi-joint space trajectory in indoor or outdoor slalom skiing, and provide scientific guidance for skiing training, so that athletes can achieve better training effectiveness.
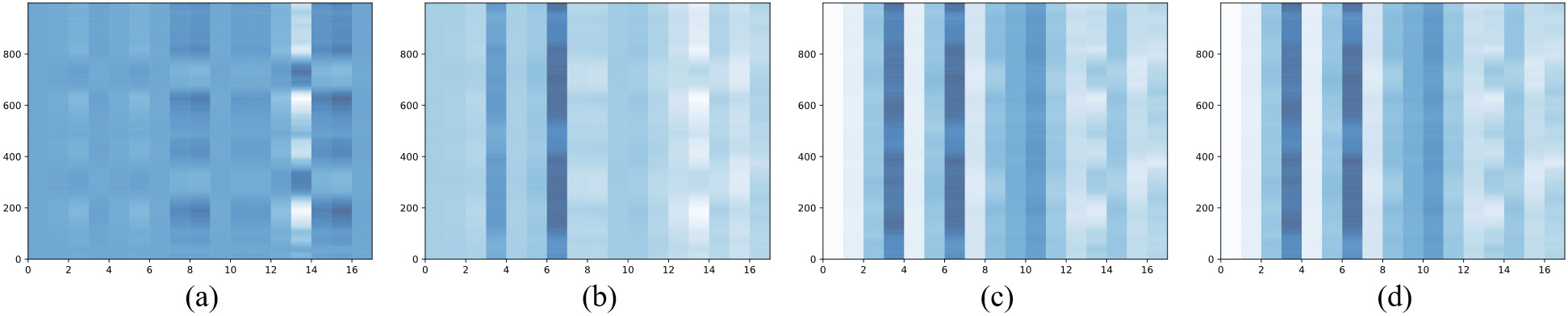
Main eigenbehaviors of slalom skiing
- [1] T. Nozawa, E. Wu, and H. Koike, “VR ski coach: Indoor ski training system visualizing difference from leading skier,” IEEE Conf. on Virtual Reality and 3D User Interfaces (VR), pp. 1341-1342, 2019.
- [2] C. M. N. Brigante et al., “Towards miniaturization of a MEMS-based wearable motion capture system,” IEEE Trans. on Industrial Electronics, Vol.58, No.8, pp. 3234-3241, 2011.
- [3] Y. Mengüç et al., “Soft wearable motion sensing suit for lower limb biomechanics measurements,” IEEE Int. Conf. on Robotics and Automation, pp. 5309-5316, 2013.
- [4] X. Wei, P. Zhang, and J. Chai, “Accurate realtime full-body motion capture using a single depth camera,” ACM Trans. on Graphics, Vol.31, No.6, Article No.188, 2012.
- [5] S. Li and A. B. Chan, “3D human pose estimation from monocular images with deep convolutional neural network,” Asian Conf. on Computer Vision, pp. 332-347, 2014.
- [6] H. Rhodin et al., “Learning monocular 3D human pose estimation from multi-view images,” Proc. of the IEEE Conf. on Computer Vision and Pattern Recognition (CVPR), pp. 8437-8446, 2018.
- [7] S. Schwarcz and T. Pollard, “3D human pose estimation from deep multi-view 2D pose,” 24th Int. Conf. on Pattern Recognition (ICPR), pp. 2326-2331, 2018.
- [8] Y. Zheng and X. Zhou (Eds.), “Computing with spatial trajectories,” Springer, 2011.
- [9] J.-G. Lee, J. Han, and K.-Y. Whang, “Trajectory clustering: a partition-and-group framework,” Proc. of the ACM SIGMOD Int. Conf. on Management of Data, pp. 593-604, 2007.
- [10] P. Kalnis, N. Mamoulis, and S. Bakiras, “On discovering moving clusters in spatio-temporal data,” Int. Symp. on Spatial and Temporal Databases, pp. 364-381, 2005.
- [11] H. Jeung, H. T. Shen, and X. Zhou, “Convoy queries in spatio-temporal databases,” IEEE 24th Int. Conf. on Data Engineering, pp. 1457-1459, 2008.
- [12] Z. Li et al., “Swarm: Mining relaxed temporal moving object clusters,” Proc. of the VLDB Endowment, Vol.3, Issues 1-2, pp. 723-734, 2010.
- [13] T. Kohonen, “The self-organizing map,” Proc. of the IEEE, Vol.78, No.9, pp. 1464-1480, 1990.
- [14] N. Eagle and A. S. Pentland, “Eigenbehaviors: Identifying structure in routine,” Behavioral Ecology and Sociobiology, Vol.63, pp. 1057-1066, 2009.
- [15] S. Wold, K. Esbensen, and P. Geladi, “Principal component analysis,” Chemometrics and Intelligent Laboratory Systems, Vol.2, Issues 1-3, pp. 37-52, 1987.
- [16] K. Farrahi and D. Gatica-Perez, “Discovering routines from large-scale human locations using probabilistic topic models,” ACM Trans. on Intelligent Systems and Technology (TIST), Vol.2, No.1, Article No.3, 2011.
- [17] P. Li, Q. Fei, and X. Yao, “Characteristic Behavior: Identifying Body Spatial Trajectory Structure In Indoor Skiing,” The 7th Int. Workshop on Advanced Computational Intelligence and Intelligent Informatics (IWACIII), Article No.T1-1-1, 2021.
- [18] H. S. Fang et al., “RMPE: Regional multi-person pose estimation,” IEEE Int. Conf. on Computer Vision (ICCV), pp. 2353-2362, 2017.
- [19] D. Pavllo et al., “3D human pose estimation in video with temporal convolutions and semi-supervised training,” Proc. of the IEEE/CVF Conf. on Computer Vision and Pattern Recognition (CVPR), pp. 7753-7762, 2019.
This article is published under a Creative Commons Attribution-NoDerivatives 4.0 Internationa License.