Paper:
Lean Rate of Posts in Different Departments Based on ANP Method
Le Yang*1,*2, Guozhang Jiang*2,*3, Gongfa Li*1,*4, and Xiaowu Chen*3
*1Key Laboratory of Metallurgical Equipment and Control Technology, Ministry of Education, Wuhan University of Science and Technology
No.947 Heping Road, Qingshan District, Wuhan, Hubei 430081, China
*2Hubei Key Laboratory of Mechanical Transmission and Manufacturing Engineering, Wuhan University of Science and Technology
No.947 Heping Road, Qingshan District, Wuhan, Hubei 430081, China
*33D Printing and Intelligent Manufacturing Engineering Institute, Wuhan University of Science and Technology
No.947 Heping Road, Qingshan District, Wuhan, Hubei 430081, China
*4Research Center of Biologic Manipulator and Intelligent Measurement and Control, Wuhan University of Science and Technology
No.947 Heping Road, Qingshan District, Wuhan, Hubei 430081, China
It is critical in a multi-sectoral company to unify the understanding of lean management, and it is conducive to solidly promoting the improvement and innovation of the company’s overall management. The parameter of the lean rate can reflect the lean situation of the enterprise and the company’s departments in a more intuitive method. At the same time, this parameter allows all departments of the company to measure their own management which is based on this, and implement a reasonable lean management.
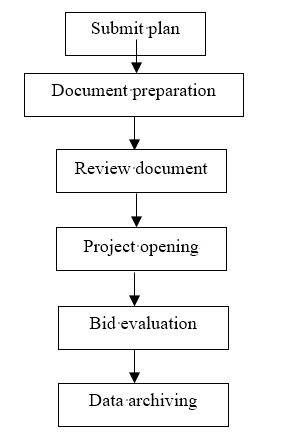
Tender department
- [1] M. Bouzon, K. Govindan, C. M. T. Rodriguez, et al., “Identification and analysis of reverse logistics barriers using fuzzy Delphi method and AHP,” Resources Conservation & Recycling, Vol.108, pp. 182-197, 2016.
- [2] J. Jiao, H. Ren, and S. Sun, “Assessment of surface ship environment adaptability in seaways: A fuzzy comprehensive evaluation method,” Int. J. of Naval Architecture and Ocean Engineering, Vol.8, No.4, pp. 344-359, 2016.
- [3] S. Yousefi, H. Shabanpour, R. Fisher et al., “Evaluating and ranking sustainable suppliers by robust dynamic data envelopment analysis,” Measurement, Vol.83, pp. 72-85, 2016.
- [4] Y. P. Jia, W. Zhao, and L. Li, “An Evaluation Method Based on Grey Relational Grade for Cutting Tool Performance in the Milling of Titanium Aircraft Components,” Materials Science Forum, Vols.836-837, pp. 270-276, 2016.
- [5] G. Charulatha, S. Srinivasalu, O. U. Maheswari, et al., “Evaluation of ground water quality contaminants using linear regression and artificial neural network models,” Arabian J. of Geosciences, Vol.10, No.6, p. 128, 2017.
- [6] W. Y. Hsu, “Fuzzy Hopfield neural network clustering for single-trial motor imagery EEG classification,” Expert Systems with Applications, Vol.39, No.1, pp. 1055-1061, 2016.
- [7] H. Wei and Y. Dong, “Adaptive Fuzzy Neural Network Control for a Constrained Robot Using Impedance Learning,” IEEE Trans. on Neural Networks and Learning Systems, Vol.29, Issue 4, pp. 1-13, 2017.
- [8] A. Graves, G. Wayne, M. Reynolds, et al., “Hybrid computing using a neural network with dynamic external memory,” Nature, Vol.538, Issue 7626, pp. 471-476, 2016.
- [9] T. Wang, H. Gao, and J. Qiu, “A Combined Adaptive Neural Network and Nonlinear Model Predictive Control for Multirate Networked Industrial Process Control,” IEEE Trans. on Neural Networks and Learning Systems, Vol.27, No.2, pp. 416-425, 2016.
- [10] M. Holweg, “The genealogy of lean production,” J. of Operations Management, Vol.25, No.2, pp. 420-437, 2007.
This article is published under a Creative Commons Attribution-NoDerivatives 4.0 Internationa License.