Paper:
Automation of Product Display Using Pose Estimation Based on Height Direction Estimation
Junya Ueda and Tsuyoshi Tasaki

Meijo University
1-501 Shiogamaguchi, Tempaku-ku, Nagoya, Aichi 468-8502, Japan
The development of product display robots in retail stores is progressing as an application of industrial robots. Pose estimation is necessary to enable robots to display products. PYNet is a high-accuracy pose estimation method for the poses of simple-shaped objects such as triangles, rectangles, and cylinders, which are common in retail stores. PYNet improves pose estimation accuracy by first estimating the object’s ground face. However, simple-shaped objects have symmetrical shapes, making it difficult to estimate poses that are inverted by 180°. To solve this problem, we focused on the upper part of the product, which often contains more information, such as logos. We developed a new method, PYNet-zmap, by enabling PYNet to recognize the height direction, defined as the direction from the bottom to the top of the product. Recognizing the height direction suppresses the 180° inversion in pose estimation and facilitates automatic product display. In pose estimation experiments using public 3D object data, PYNet-zmap achieved a correct rate of 79.2% within a 30° error margin, an improvement of 2.8 points compared with the conventional PYNet. We also implemented PYNet-zmap on a 6-axis robot arm and conducted product display experiments. As a result, 82.5% of the products were displayed correctly, an improvement of 8.9 points compared to using PYNet for pose estimation.
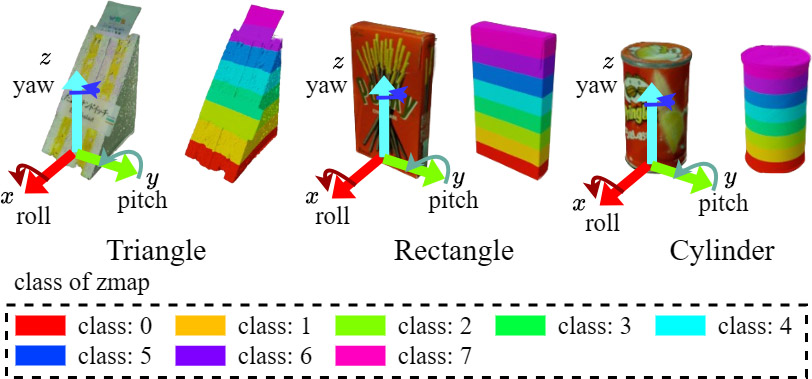
PYNet-zmap estimates height direction
- [1] R. Tomikawa, Y. Ibuki, K. Kobayashi, K. Matsumoto, H. Suito, Y. Takemura, M. Suzuki, T. Tasaki, and K. Ohara, “Development of display and disposal work system for convenience stores using dual-arm robot,” Advanced Robotics, Vol.36, No.23, pp. 1273-1290, 2022. https://doi.org/10.1080/01691864.2022.2136503
- [2] H. Tsuji, M. Shii, S. Yokoyama, Y. Takamido, Y. Murase, S. Masaki, and K. Ohara, “Reusable robot system for display and disposal tasks at convenience stores based on a SysML model and RT middleware,” Advanced Robotics, Vol.34, Nos.3-4, pp. 250-264, 2020. https://doi.org/10.1080/01691864.2019.1700160
- [3] J. Tanaka, D. Yamamoto, H. Ogawa, H. Ohtsu, K. Kamata, and K. Nara, “Portable compact suction pad unit for parallel grippers,” Advanced Robotics, Vol.34, Nos.3-4, pp. 202-218, 2020. https://doi.org/10.1080/01691864.2019.1686421
- [4] R. Sakai, S. Katsumata, T. Miki, T. Yano, W. Wei, Y. Okadome, N. Chihara, N. Kimura, Y. Nakai, I. Matsuo, and T. Shimizu, “A mobile dual-arm manipulation robot system for stocking and disposing of items in a convenience store by using universal vacuum grippers for grasping items,” Advanced Robotics, Vol.34, Nos.3-4, pp. 219-234, 2020. https://doi.org/10.1080/01691864.2019.1705909
- [5] C. Wang, D. Xu, Y. Zhu, R. Martín-Martín, C. Lu, L. Fei-Fei, and S. Savarese, “Densefusion: 6d object pose estimation by iterative dense fusion,” 2019 IEEE/CVF Conf. on Computer Vision and Pattern Recognition (CVPR), pp. 3338-3347, 2019. https://doi.org/10.1109/CVPR.2019.00346
- [6] Y. He, W. Sun, H. Huang, J. Liu, H. Fan, and J. Sun, “PVN3D: A deep point-wise 3d keypoints voting network for 6dof pose estimation,” 2020 IEEE/CVF Conf. on Computer Vision and Pattern Recognition (CVPR), pp. 11629-11638, 2020. https://doi.org/10.1109/CVPR42600.2020.01165
- [7] Y. He, H. Huang, H. Fan, Q. Chen, and J. Sun, “FFB6D: A full flow bidirectional fusion network for 6d pose estimation,” 2021 IEEE/CVF Conf. on Computer Vision and Pattern Recognition (CVPR), pp. 3002-3012, 2021. https://doi.org/10.1109/CVPR46437.2021.00302
- [8] K. Fujita and T. Tasaki, “PYNet: Poseclass and yaw angle output network for object pose estimation,” J. Robot. Mechatron., Vol.35, No.1, pp. 8-17, 2023. https://doi.org/10.20965/jrm.2023.p0008
- [9] B. Calli, A. Singh, A. Walsman, S. Srinivasa, P. Abbeel, and A. M. Dollar, “The ycb object and model set: Towards common benchmarks for manipulation research,” 2015 Int. Conf. on Advanced Robotics (ICAR), pp. 510-517, 2015. https://doi.org/10.1109/ICAR.2015.7251504
- [10] H. Wang, S. Sridhar, J. Huang, J. Valentin, S. Song, and L. J. Guibas, “Normalized object coordinate space for category-level 6d object pose and size estimation,” 2019 IEEE/CVF Conf. on Computer Vision and Pattern Recognition (CVPR), pp. 2637-2646, 2019. https://doi.org/10.1109/CVPR.2019.00275
- [11] G. Li, Y. Li, Z. Ye, Q. Zhang, T. Kong, Z. Cui, and G. Zhang, “Generative category-level shape and pose estimation with semantic primitives,” 6th Conf. on Robot Learning (CoRL 2022), 2022. https://doi.org/10.48550/arXiv.2210.01112
- [12] S. Hinterstoisser, V. Lepetit, S. Ilic, S. Holzer, G. Bradski, K. Konolige, and N. Navab, “Model based training, detection and pose estimation of texture-less 3d objects in heavily cluttered scenes,” Asian Conf. on Computer Vision, 2013. https://doi.org/10.1007/978-3-642-37331-2_42
- [13] M. Zaccaria, F. Manhardt, Y. Di, F. Tombari, J. Aleotti, and M. Giorgini, “Self-supervised category-level 6d object pose estimation with optical flow consistency,” IEEE Robotics and Automation Letters, Vol.8, No.5, pp. 2510-2517, 2023. https://doi.org/10.1109/LRA.2023.3254463
- [14] J. Lin, Z. Wei, Y. Zhang, and K. Jia, “VI-Net: Boosting category-level 6d object pose estimation via learning decoupled rotations on the spherical representations,” 2023 IEEE/CVF Int. Conf. on Computer Vision (ICCV), pp. 13955-13965, 2023. https://doi.org/10.1109/ICCV51070.2023.01287
- [15] H. Wang, W. Li, J. Kim, and Q. Wang, “Attention-guided RGB-D fusion network for category-level 6d object pose estimation,” 2022 IEEE/RSJ Int. Conf. on Intelligent Robots and Systems (IROS), pp. 10651-10658, 2022. https://doi.org/10.1109/IROS47612.2022.9981242
- [16] K. Fujita and T. Tasaki, “Zero-shot pose estimation using image translation maintaining object pose,” 2024 IEEE/SICE Int. Symp. on System Integration (SII), pp. 447-452, 2024. https://doi.org/10.1109/SII58957.2024.10417482
- [17] M. Tan and Q. Le, “EfficientNet: Rethinking model scaling for convolutional neural networks,” 36th Int. Conf. on Machine Learning, 2019. https://doi.org/10.48550/arXiv.1905.11946
- [18] J. Deng, W. Dong, R. Socher, L.-J. Li, K. Li, and L. Fei-Fei, “Imagenet: A large-scale hierarchical image database,” 2009 IEEE Conf. on Computer Vision and Pattern Recognition, pp. 248-255, 2009. https://doi.org/10.1109/CVPR.2009.5206848
- [19] S. Tyree, J. Tremblay, T. To, J. Cheng, T. Mosier, J. Smith, and S. Birchfield, “6-dof pose estimation of household objects for robotic manipulation: An accessible dataset and benchmark,” 2022 IEEE/RSJ Int. Conf. on Intelligent Robots and Systems (IROS), pp. 13081-13088, 2022.
- [20] X. Qin, Z. Zhang, C. Huang, M. Dehghan, O. R. Zaiane, and M. Jagersand, “U2-net: Going deeper with nested u-structure for salient object detection,” Pattern Recognition, Vol.106, Article No.107404, 2020. https://doi.org/10.1016/j.patcog.2020.107404
- [21] H. Okada, T. Inamura, and K. Wada, “What competitions were conducted in the service categories of the world robot summit?,” Advanced Robotics, Vol.33, No.17, pp. 900-910, 2019. https://doi.org/10.1080/01691864.2019.1663608
This article is published under a Creative Commons Attribution-NoDerivatives 4.0 Internationa License.