Paper:
Toilet Floor Trash Detection System for Unidentified Solid Waste
Rama Okta Wiyagi*,** and Kazuyoshi Wada*

*Graduate School of Systems Design, Tokyo Metropolitan University
6-6 Asahigaoka, Hino, Tokyo 191-0065, Japan
**Department of Electrical Engineering, Universitas Muhammadiyah Yogyakarta
Jln. Brawijaya, Kasihan, Bantul, Yogyakarta 55183, Indonesia
The maintenance of public toilets, such as those found in convenience stores, presents challenges in the era of limited human resources. Consequently, the development of an automatic toilet cleaning system is necessary. The detection of trash on the toilet floor is an essential component of the automatic toilet cleaning system. However, this process presents its own set of challenges, including the unpredictability of the types and locations of the trash that may be present. This study proposes a system for detecting solid waste on the toilet floor by applying the structure and feature similarity index method of image. The difference in the structure and features of the reference and actual images can indicate the trash that appears on the toilet floor. This study also proposes a method for determining the threshold value of similarity feature measurement. The experimental results demonstrate that the proposed detection system is able to produce a detection success rate of up to 96.5%. Additionally, the system proves capable of detecting small objects, such as human hair, under specific conditions. This method offers a resource-efficient solution to the challenges faced in maintaining public toilet cleanliness.
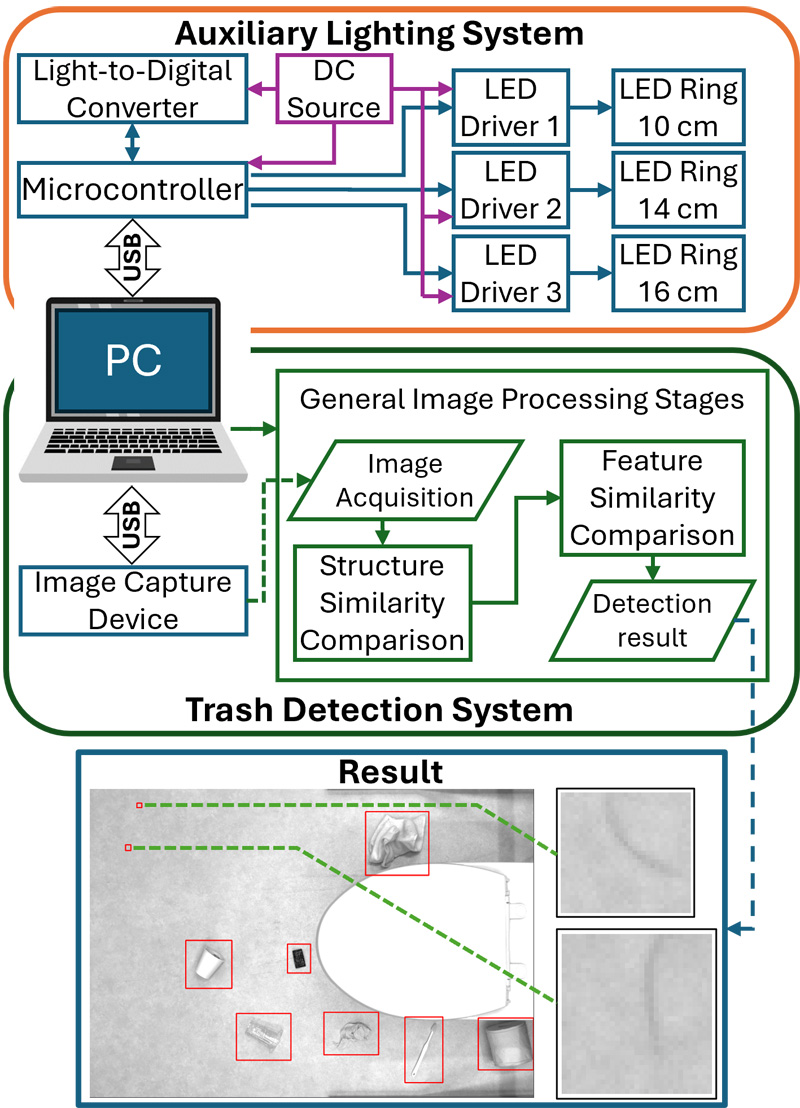
Toilet floor trash detection system
- [1] S. Isaksson and M. Bergentall, “Sensor-based hygiene monitoring in restrooms and related areas – A review,” RISE Report, Vol.2023, No.138, p. 34, 2023.
- [2] Y. Komatsu, “Role of the convenience stores as a new public toilet part 2 analysis of toilet use by location,” Annual Meeting of the Society of Heating, Air-Conditioning and Sanitary Engineers of Japan 2007, pp. 9-12, 2007. https://doi.org/10.18948/shasetaikai.2007.1.0_9
- [3] Japan Ministry of Land, Infrastructure, Transport and Tourism, “Survey on users’ awareness of public toilets and actual usage 2020,” 2020.
- [4] Japan Ministry of Land, Infrastructure, Transport and Tourism, “Questionnaire survey on toilets frequently used in daily life,” 2016.
- [5] B. O. Olatunji and T. Armstrong, “Contamination fear and effects of disgust on distress in a public restroom,” Emotion, Vol.9, No.4, pp. 592-597, 2009. https://doi.org/10.1037/a0016109
- [6] J.-P. Roy, “Managing a demographically driven labor shortage: An assessment of japan’s strategic approach,” Int. Business in the New Asia-Pacific: Strategies, Opportunities and Threats, pp. 75-125, 2022. https://doi.org/10.1007/978-3-030-87621-0_4
- [7] H. Molotch and L. Norén, “Toilet: Public Restrooms and the Politics of Sharing,” NYU Press, Vol.1, 2010.
- [8] S. Kose, “How can we assure that everyone will have a toilet s/he can use?,” Session 4A-Designing with People: Achieving Cohesion, p. 324, 2009.
- [9] J. Poggiali and S. Margolin, “Peeking at the potty: Learning from academic library bathrooms and advocating change,” J. of Library Administration, Vol.59, No.7, pp. 743-767, 2019. https://doi.org/10.1080/01930826.2019.1649965
- [10] J. Kobayashi and S. Nagasawa, “Design and maintenance for toilet of commercial facilities,” J. of Architecture and Planning (Trans. of AIJ), Vol.79, No. 699, pp. 1099-1108, 2014. https://doi.org/10.3130/aija.79.1099
- [11] A. Grünauer, G. Halmetschlager-Funek, J. Prankl, and M. Vincze, “The power of gmms: unsupervised dirt spot detection for industrial floor cleaning robots,” Annual Conf. Towards Autonomous Robotic Systems, pp. 436-449, 2017. https://doi.org/10.1007/978-3-319-64107-2_34
- [12] R. Bormann, J. Fischer, G. Arbeiter, F. Weisshardt, and A. Verl, “A visual dirt detection system for mobile service robots,” ROBOTIK 2012: 7th German Conf. on Robotics, 2012.
- [13] B. Ramalingam, P. Veerajagadheswar, M. Ilyas, M. R. Elara, and A. Manimuthu, “Vision-based dirt detection and adaptive tiling scheme for selective area coverage,” J. of Sensors, Vol.2018, 2018. https://doi.org/10.1155/2018/3035128
- [14] R. Bormann, F. Weisshardt, G. Arbeiter, and J. Fischer, “Autonomous dirt detection for cleaning in office environments,” 2013 IEEE Int. Conf. on Robotics and Automation, pp. 1260-1267, 2013. https://doi.org/10.1109/ICRA.2013.6630733
- [15] R. Bormann, X. Wang, J. Xu, and J. Schmidt, “Dirtnet: visual dirt detection for autonomous cleaning robots,” 2020 IEEE Int. Conf. on Robotics and Automation (ICRA), pp. 1977-1983, 2020. https://doi.org/10.1109/ICRA40945.2020.9196559
- [16] J. Xue, Z. Li, M. Fukuda, T. Takahashi, M. Suzuki, Y. Mae, Y. Arai, and S. Aoyagi, “Garbage detection using yolov3 in nakanoshima challenge,” J. Robot. Mechatron., Vol.32, No.6, pp. 1200-1210, 2020. https://doi.org/10.20965/jrm.2020.p1200
- [17] R. Miyagusuku, Y. Arai, Y. Kakigi, T. Takebayashi, A. Fukushima, and K. Ozaki, “Toward autonomous garbage collection robots in terrains with different elevations,” J. Robot. Mechatron., Vol.32, No.6, pp. 1164-1172, 2020. https://doi.org/10.20965/jrm.2020.p1164
- [18] R. Harada, T. Oyama, K. Fujimoto, T. Shimizu, M. Ozawa, J. S. Amar, and M. Sakai, “Trash detection algorithm suitable for mobile robots using improved yolo,” J. Adv. Comput. Intell. Intell. Inform., Vol.27, No.4, pp. 622-631, 2023. https://doi.org/10.20965/jaciii.2023.p0622
- [19] D. Canedo, P. Fonseca, P. Georgieva, and A. J. Neves, “A deep learning-based dirt detection computer vision system for floor-cleaning robots with improved data collection,” Technologies, Vol.9, No.4, Article No.94, 2021. https://doi.org/10.3390/technologies9040094
- [20] N. Shinoki, K. Wada, A. Hori, T. Tomizawa, and T. Suzuki, “Evaluation of repeated cleaning ability of a restroom cleaning system for scattered urine,” 2020 IEEE/SICE Int. Symp. on System Integration (SII), pp. 976-979, 2020. https://doi.org/10.1109/SII46433.2020.9026220
- [21] G. Garcia Ricardez, N. Koganti, P.-C. Yang, S. Okada, P. Uriguen Eljuri, A. Yasuda, L. El Hafi, M. Yamamoto, J. Takamatsu, and T. Ogasawara, “Adaptive motion generation using imitation learning and highly compliant end effector for autonomous cleaning,” Advanced Robotics, Vol.34, Nos.3-4, pp. 189-201, 2020. https://doi.org/10.1080/01691864.2019.1698461
- [22] L. Jayasinghe, N. Wijerathne, and C. Yuen, “A deep learning approach for classification of cleanliness in restrooms,” 2018 Int. Conf. on Intelligent and Advanced System (ICIAS), 2018. https://doi.org/10.1109/ICIAS.2018.8540592
- [23] L. Jayasinghe, N. Wijerathne, C. Yuen, and M. Zhang, “Feature learning and analysis for cleanliness classification in restrooms,” IEEE Access, Vol.7, pp. 14871-14882, 2019. https://doi.org/10.1109/ACCESS.2019.2894006
- [24] R. O. Wiyagi and K. Wada, “Water droplet detection system on toilet floor using heat absorption capacity of liquid,” J. Robot. Mechatron., Vol.36, No.2, pp. 388-395, 2024. https://doi.org/10.20965/jrm.2024.p0388
- [25] R. O. Wiyagi and K. Wada, “Development of convenience stores toilet floor trash detection,” 23rd Society of Instrument and Control Engineers System Integration Division Lecture (SI2022), Article No.3A2-F03, 2022.
- [26] S. Chandra, S. Srivastava, and A. Roy, “Public toilet hygiene monitoring and reporting system,” 2018 IEEE SENSORS, 2018. https://doi.org/10.1109/ICSENS.2018.8589894
- [27] C. Manigandaa, V. A. Kumar, M. Nabeel, A. K. Sabai, R. Selvalingeshwaran, V. A. Kumar, and R. Priya, “Toilet odour detection using fuzzy logic technique,” 2022 Third Int. Conf. on Intelligent Computing Instrumentation and Control Technologies (ICICICT), pp. 917-923, 2022. https://doi.org/10.1109/ICICICT54557.2022.9917797
- [28] S. Kirithika, L. M. Kumar, M. K. Kumar, E. Keerthana, and R. Lohalavanya, “Smart public toilets using ioe,” 2020 Int. Conf. on Emerging Trends in Information Technology and Engineering (ic-ETITE), 2020. https://doi.org/10.1109/ic-ETITE47903.2020.429
- [29] Z. Wang, A. C. Bovik, H. R. Sheikh, and E. P. Simoncelli, “Image quality assessment: From error visibility to structural similarity,” IEEE Trans. on Image Processing, Vol.13, No.4, pp. 600-612, 2004. https://doi.org/10.1109/TIP.2003.819861
- [30] D. M. Rouse and S. S. Hemami, “Understanding and simplifying the structural similarity metric,” 2008 15th IEEE Int. Conf. on Image Processing, pp. 1188-1191, 2008. https://doi.org/10.1109/ICIP.2008.4711973
- [31] G. Palubinskas, “Image similarity/distance measures: what is really behind mse and ssim?,” Int. J. of Image and Data Fusion, Vol.8, No.1, pp. 32-53, 2017. https://doi.org/10.1080/19479832.2016.1273259
- [32] U. Sara, M. Akter, and M. S. Uddin, “Image quality assessment through fsim, ssim, mse and psnr—A comparative study,” J. of Computer and Communications, Vol.7, No.3, pp. 8-18, 2019. https://doi.org/10.4236/jcc.2019.73002
- [33] J. Peng, C. Shi, E. Laugeman, W. Hu, Z. Zhang, S. Mutic, and B. Cai, “Implementation of the structural similarity (ssim) index as a quantitative evaluation tool for dose distribution error detection,” Medical Physics, Vol.47, No.4, pp. 1907-1919, 2020. https://doi.org/10.1002/mp.14010
- [34] A. Sciarra, S. Chatterjee, M. Dünnwald, G. Placidi, A. Nürnberger, O. Speck, and S. Oeltze-Jafra, “Automated ssim regression for detection and quantification of motion artefacts in brain mr images,” arXiv preprint, arXiv:2206.06725, 2022. https://doi.org/10.48550/arXiv.2206.06725
- [35] A. Tolba and H. M. Raafat, “Multiscale image quality measures for defect detection in thin films,” The Int. J. of Advanced Manufacturing Technology, Vol.79, pp. 113-122, 2015. https://doi.org/10.1007/s00170-014-6758-7
- [36] B. Xia, J. Cao, and C. Wang, “Ssim-net: Real-time pcb defect detection based on ssim and mobilenet-v3,” 2019 2nd World Conf. on Mechanical Engineering and Intelligent Manufacturing (WCMEIM), pp. 756-759, 2019. https://doi.org/10.1109/WCMEIM48965.2019.00159
- [37] M. Zhou, G. Wang, J. Wang, C. Hui, and W. Yang, “Defect detection of printing images on cans based on ssim and chromatism,” 2017 3rd IEEE Int. Conf. on Computer and Communications (ICCC), pp. 2127-2131, 2017. https://doi.org/10.1109/CompComm.2017.8322912
- [38] A. Bionda, L. Frittoli, and G. Boracchi, “Deep autoencoders for anomaly detection in textured images using cw-ssim,” Int. Conf. on Image Analysis and Processing, pp. 669-680, 2022. https://doi.org/10.1007/978-3-031-06430-2_56
- [39] L. Zhang, L. Zhang, X. Mou, and D. Zhang, “Fsim: A feature similarity index for image quality assessment,” IEEE Trans. on Image Processing, Vol.20, No.8, pp. 2378-2386, 2011. https://doi.org/10.1109/TIP.2011.2109730
- [40] W. Preedanan, T. Kondo, P. Bunnun, and I. Kumazawa, “A comparative study of image quality assessment,” 2018 Int. Workshop on Advanced Image Technology (IWAIT), 2018. https://doi.org/10.1109/IWAIT.2018.8369657
- [41] L. Zhang, L. Zhang, X. Mou, and D. Zhang, “A comprehensive evaluation of full reference image quality assessment algorithms,” 2012 19th IEEE Int. Conf. on Image Processing, pp. 1477-1480, 2012. https://doi.org/10.1109/ICIP.2012.6467150
- [42] S. Moldovanu, L. Moraru, V. Stefanescu, and M. Georgescu, “The hough transform and the fsim similarity index for the sagittal axis identification,” Annals of the University Dunarea de Jos of Galati: Fascicle II, Mathematics, Physics, Theoretical Mechanics, Vol.38, No.1, 2015.
- [43] J. John, M. S. Nair, P. A. Kumar, and M. Wilscy, “A novel approach for detection and delineation of cell nuclei using feature similarity index measure,” Biocybernetics and Biomedical Engineering, Vol.36, No.1, pp. 76-88, 2016. https://doi.org/10.1016/j.bbe.2015.11.002
- [44] N. A. Shnain, Z. M. Hussain, and S. F. Lu, “A feature-based structural measure: An image similarity measure for face recognition,” Applied Sciences, Vol.7, No.8, Article No.786, 2017. https://doi.org/10.3390/app7080786
- [45] N. A. Shnain, S. F. Lu, and Z. M. Hussain, “Hos image similarity measure for human face recognition,” 2017 3rd IEEE Int. Conf. on Computer and Communications (ICCC), pp. 1621-1625, 2017. https://doi.org/10.1109/CompComm.2017.8322814
- [46] Y. Liu, K. Fan, D. Wu, and W. Zhou, “Filter pruning by quantifying feature similarity and entropy of feature maps,” Neurocomputing, Vol.544, Article No.126297, 2023. https://doi.org/10.1016/j.neucom.2023.126297
This article is published under a Creative Commons Attribution-NoDerivatives 4.0 Internationa License.