Paper:
Muscle Property Optimization Based on Motion Intentionality for Motor Skill Augmentation
Hinako Suzuki*, Ko Ayusawa**
, and Akihiko Murai*,**

*Graduate School of Frontier Sciences, The University of Tokyo
6-2-3 Kashiwanoha, Kashiwa, Chiba 277-0882, Japan
**Human Augmentation Research Center, National Institute of Advanced Industrial Science and Technology
6-2-3 Kashiwanoha, Kashiwa, Chiba 277-0882, Japan
Understanding motion intentionality is important to augment motor skills. This can be explained by the anatomical structure and innate immune mechanisms of humans. This study aimed to analyze human motion using musculoskeletal information and estimate motion intentionality, motion direction, and muscle properties. The calculation method for the easy-to-move musculoskeletal direction in the task space was reversed to calculate the optimal muscle properties and matching rate for the motion direction. The analysis results of each walking and feint motion showed that the muscles were trained toward reduced muscular effort and improved skills for each motion. In addition, the results of the optimization of the matching rate and muscle properties indicate that the optimized muscle properties can express the motion better than the reference values, and the average matching rate increases by 2.41×10-1, particularly for a feint motion. Therefore, skill acquisition and augmentation were achieved.
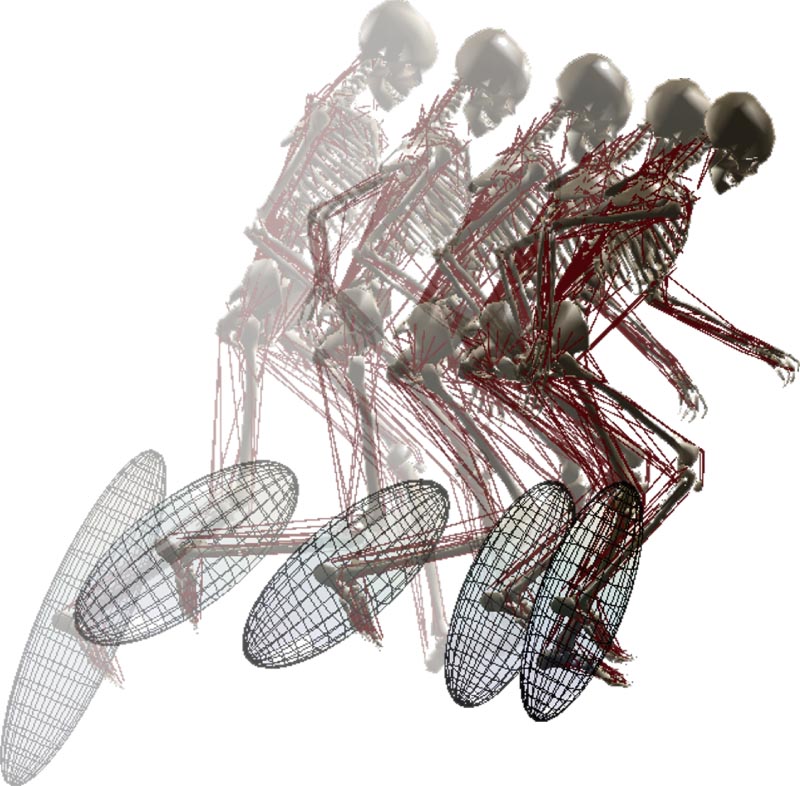
Muscle property optimization for ease of motion
- [1] P. D. MacLean, “The Triune Brain in Evolution: Role in Paleocerebral Functions,” Springer, 1990.
- [2] A. Murai, K. Yamane, and Y. Nakamura, “Effects of nerve signal transmission delay in somatosensory reflex modeling based on inverse dynamics and optimization,” 2010 IEEE Int. Conf. Robot. Autom., pp. 5076-5083, 2010. https://doi.org/10.1109/ROBOT.2010.5509611
- [3] S. Ikemoto, Y. Kimoto, and K. Hosoda, “Shoulder complex linkage mechanism for humanlike musculoskeletal robot arms,” Bioinspir. Biomim., Vol.10, No.6, Article No.066009, 2015. https://doi.org/10.1088/1748-3190/10/6/066009
- [4] Y. Nakamura, K. Yamane, Y. Fujita, and I. Suzuki, “Somatosensory computation for man-machine interface from motion-capture data and musculoskeletal human model,” IEEE Trans. Robot., Vol.21, No.1, pp. 58-66, 2005. https://doi.org/10.1109/TRO.2004.833798
- [5] S. L. Delp et al., “OpenSim: Open-source software to create and analyze dynamic simulations of movement,” IEEE Trans. Biomed. Eng., Vol.54, No.11, pp. 1940-1950, 2007. https://doi.org/10.1109/TBME.2007.901024
- [6] S. Mori and T. Shimada, “Expert anticipation from deceptive action,” Atten. Percept. Psychophys., Vol.75, No.4, pp. 751-770, 2013. https://doi.org/10.3758/s13414-013-0435-z
- [7] E. Wu and H. Koike, “FuturePong: Real-time table tennis trajectory forecasting using pose prediction network,” Ext. Abstr. 2020 CHI Conf. Hum. Factors Comput. Syst. (CHI EA’20), pp. 1-8, 2020. https://doi.org/10.1145/3334480.3382853
- [8] O. Khatib et al., “Robotics-based synthesis of human motion,” J. Physiol. Paris, Vol.103, Nos.3-5, pp. 211-219, 2009. https://doi.org/10.1016/j.jphysparis.2009.08.004
- [9] K. Ayusawa, A. Murai, R. Sagawa, and E. Yoshida, “Fast inverse kinematics based on pseudo-forward dynamics computation: Application to musculoskeletal inverse kinematics,” IEEE Robot. Autom. Lett., Vol.8, No.9, pp. 5775-5782, 2023. https://doi.org/10.1109/LRA.2023.3300207
This article is published under a Creative Commons Attribution-NoDerivatives 4.0 Internationa License.