Paper:
Local Discrimination Based on Piezoelectric Sensing in Robots Composed of Soft Matter with Different Physical Properties
Ikuma Sudo, Jun Ogawa, Yosuke Watanabe, MD Nahin Islam Shiblee, Ajit Khosla, Masaru Kawakami, and Hidemitsu Furukawa
Graduate School of Science and Technology, Yamagata University
4-3-16 Jonan, Yonezawa, Yamagata 992-8510, Japan
The coronavirus epidemic has attracted significant attention to the applications of pet robots which can be used to treat and entertain people in their homes. However, pet robots are fabricated using hard materials and it is difficult for them to communicate with people through contact. Soft robots are expected to realize communication through contact similar to that of actual pets. Soft robots provide people with a sense of healing and security owing to their softness and can extract rich information through external stimuli by applying a machine learning framework called physical-reservoir computing. It is crucial to determine the differences between the physical properties of soft materials that affect the information extracted from a soft body to develop an intelligent soft robot. In this study, two owl-shaped soft robots with different softnesses were developed to analyze the characteristics of the signal data obtained via piezoelectric film sensors embedded in models with different physical properties. An accuracy of 94.2% and 95.9% was obtained for touched part classification using 1D CNN and logistic regression models, respectively. Additionally, the relationship between the softness of material and classification performance was investigated by comparing the distribution of part classification accuracy for different hyper-parameters of two owl models.
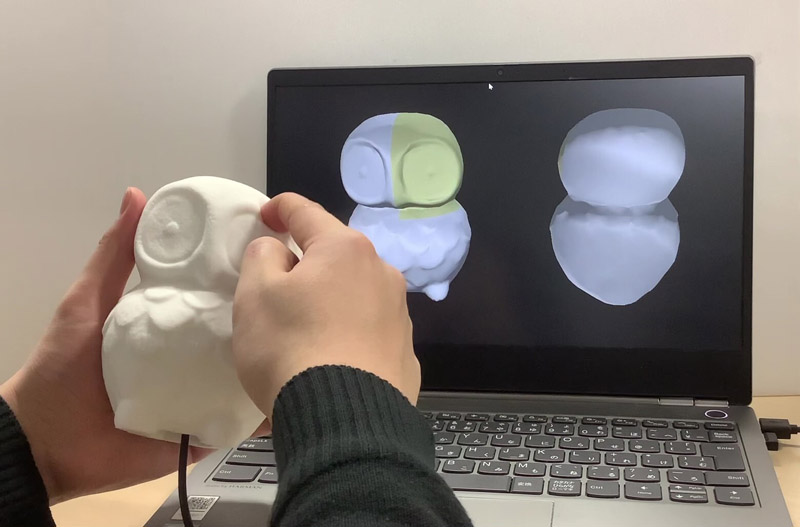
Part recognition with owl soft robot
- [1] T. Nakashima, G. Fukutome, and N. Ishii, “Healing effects of pet robots at an elderly-care facility,” IEEE/ACIS 9th Int. Conf. on Computer and Information Science, pp. 407-412, 2010.
- [2] F. Tanaka, K. Isshiki, F. Takahashi, M. Uekusa, R. Sei, and K. Hayashi, “Pepper Learns together with children: Development of an educational application,” IEEE-RAS Int. Conf. on Humanoid Robots, Vol.2015-December, pp. 270-275, 2015.
- [3] C. Fernando and S. Sojakka, “Pattern recognition in a bucket,” European Conf. on Artificial Life, pp. 588-597, 2003.
- [4] K. Nakajima, H. Hauser, T. Li, and R. Pfeifer, “Information processing via physical soft body,” Scientific Reports, Vol.5, 10487, 2015.
- [5] G. Dion, S. Mejaouri, and J. Sylvestre, “Reservoir computing with a single delay-coupled non-linear mechanical oscillator,” J. of Applied Physics, Vol.124, No.15, 152132, 2018.
- [6] J. Guckenheimer and P. Holmes, “Nonlinear oscillations, dynamical systems, and bifurcations of vector fields (Applied Mathematical Sciences, Vol.42),” Springer, 2013.
- [7] K. P. Dockendorf, I. Park, P. He, J. C. Princípe, and T. B. DeMarse, “Liquid state machines and cultured cortical networks: The separation property,” Biosystems, Vol.95, No.2, pp. 90-97, 2009.
- [8] M. R. Dranias, H. Ju, E. Rajaram, and A. M. J. VanDongen, “Short-term memory in networks of dissociated cortical neurons,” J. of Neuroscience, Vol.33, No.5, pp. 1940-1953, 2013.
- [9] L. Appeltant, M. C. Soriano, G. V. Sande, J. Danckaert, S. Massar, J. Dambre, B. Schrauwen, C. R. Mirasso, and I. Fischer, “Information processing using a single dynamical node as complex system,” Nature Communications, 2011.
- [10] L. Appeltant, G. V. Sande, J. Danckaert, and I. Fischer, “Constructing optimized binary masks for reservoir computing with delay systems,” Scientific Reports, Vol.4, 3629, 2014.
- [11] K. Caluwaerts, J. Despraz, A. Işçen, A. P. Sabelhaus, J. Bruce, B. Schrauwen, and V. SunSpiral, “Design and control of compliant tensegrity robots through simulation and hardware validation,” J. of the Royal Society Interface, Vol.11, No.98, 20140520, 2014.
- [12] Q. Zhao, K. Nakajima, H. Sumioka, H. Hauser, and R. Pfeifer, “Spine dynamics as a computational resource in spine-driven quadruped locomotion,” IEEE/RSJ Int. Conf. on Intelligent Robots and Systems, pp. 1445-1451, 2013.
- [13] M. Eder, F. Hisch, and H. Hauser, “Morphological computation-based control of a modular, pneumatically driven, soft robotic arm,” Advanced Robotics, Vol.32, No.7, pp. 375-385, 2018.
- [14] T. Kawase, T. Miyazaki, T. Kanno, K. Tadano, Y. Nakajima, and K. Kawashima, “Pneumatic Reservoir Computing for Sensing Soft Body: Computational Ability of Air in Tube and Its Application to Posture Estimation of Soft Exoskeleton,” Sensors and Materials, Vol.33, No.8, pp. 2803-2824, 2021.
- [15] Y. Horii, K. Inoue, S. Nishikawa, K. Nakajima, R. Niiyama, and Y. Kuniyoshi, “Physical reservoir computing in a soft swimming robot,” The 2021 Conf. on Artificial Life (ALIFE 2021), MIT Press, 2021.
- [16] P. Bhovad and S. Li, “Physical reservoir computing with origami and its application to robotic crawling,” Scientific Reports, Vol.11, No.1, pp. 1-18, 2021.
- [17] K. Tanaka, S. H. Yang, Y. Tokudome, Y. Minami, Y. Lu, T. Arie, S. Akita, K. Takei, and K. Nakajima, “Flapping – Wing Dynamics as a Natural Detector of Wind Direction,” Advanced Intelligent Systems, Vol.3, No.2, 2000174, 2021.
- [18] K. Nakajima, K inoue, Y. Kuniyoshi, S. Somlor, T. P. Tomo, and A. Schmitz, “Exploiting the Deformation Dynamics of Soft Materials as an Information Processing Device,” The J. of the Institute of Electronics, Information and Communication Engineers, Vol.102, No.2, pp. 121-126, 2019.
- [19] Md. A. Haque, T. Kurokawa, and J. Gong, “Super tough double network hydrogels and their application as biomaterials,” Polymer, Vol.53, No.9, pp. 1805-1822, 2012.
- [20] M. Christ, N. Braun, J. Neuffer, and A. W. Kempa-Liehr, “Time series feature extraction on basis of scalable hypothesis tests (tsfresh – a python package),” Neurocomputing, Vol.307, pp. 72-77, 2018.
- [21] S. Kiranyaz, T. Ince, R. Hamila, and M. Gabbouj, “Convolutional Neural Networks for patient-specific ECG classification,” 2015 37th Annual Int. Conf. of the IEEE Engineering in Medicine and Biology Society (EMBC), pp. 2608-2611, 2015.
- [22] S. Kiranyaz, T. Ince, R Hamila, and M. Gabbouj, “Real-time patient-specific ECG classification by 1-D convolutional neural networks,” IEEE Trans. on Biomedical Engineering, Vol.63, No.3, pp. 664-675, 2015.
- [23] S. Kiranyaz, T. Ince, and M. Gabbouj, “Personalized monitoring and advance warning system for cardiac arrhythmias,” Scientific Reports, Vol.7, No.1, 9270, 2017.
- [24] S. Kiranyaz, O. Avci, O. Abdeljaber, T. Ince, M. Gabbouj, and D. J. Inman, “1D convolutional neural networks and applications: A survey,” Mechanical Systems and Signal Processing, Vol.151, 2021.
- [25] F. Pedregosa, G. Varoquaux, A. Gramfort, V. Michel, B. Thirion, O. Grisel, M. Blondel, P. Prettenhofer, R. Weiss, V. Dubourg, J. Vanderplas, A. Passos, D. Cournapeau, M. Brucher, M. Perrot, and E. Duchesnay, “Scikit-learn: Machine Learning in Python,” J. of Machine Learning Research, Vol.12, pp. 2825-2830, 2011.
This article is published under a Creative Commons Attribution-NoDerivatives 4.0 Internationa License.