Paper:
Study on Human Behavior Classification by Using High-Performance Shoes Equipped with Pneumatic Actuators
Yasuhiro Hayakawa*, Yuta Kimata**, and Keisuke Kida**
*Department of Control Engineering, National Institute of Technology, Nara College
22 Yata-cho, Yamatokoriyama, Nara 639-1058, Japan
**Advanced Mechanical Engineering Course, National Institute of Technology, Nara College
22 Yata-cho, Yamatokoriyama, Nara 639-1058, Japan
In Japan, accidents involving the falling of elderly people are increasingly becoming a problem. To solve this problem, walking training is effective for preventing falls of elderly people. In this study, a walking training system was developed in which high-performance shoes are used to improve the efficiency of walking training. The high-performance shoes have three functions: 1) measurement of plantar pressure using changes in the inner pressure of the insole, 2) leg movement measurement using a six-axis motion sensor, and 3) applying stimulus to the sole of the foot by changing the shape of the insole. A unique rubber element was developed for these functions. Furthermore, a system to predict the behavior of patients during walking training was developed. Based on experimental results, four types of behavior of patients during walking training were predicted. Moreover, leave-one-person-out cross validation was performed by the random forest (RF) machine-learning algorithm, and the F-measure was calculated. As a result, the four types of behavior were classified with an F-measure of 78.6%.
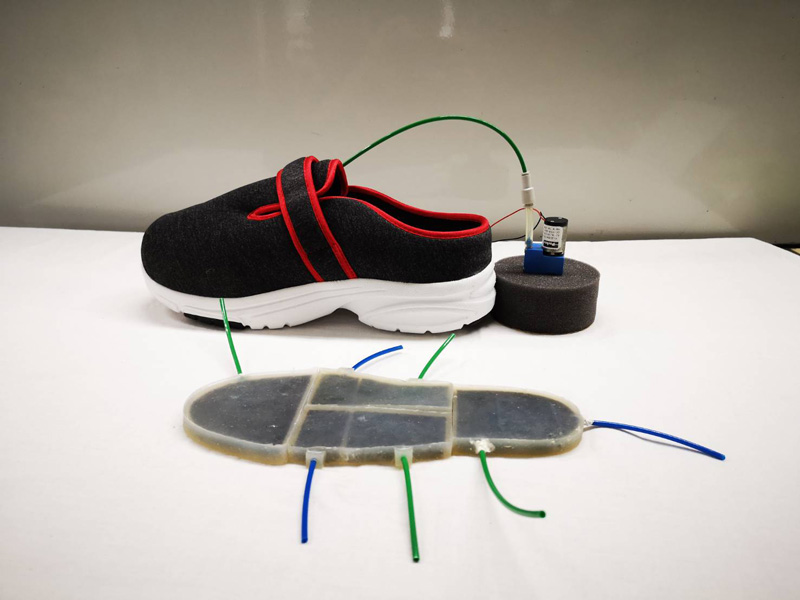
The shoes and dedicated insole elements
- [1] Cabinet Office, “Situation of the Ageing Population,” Annual Report on the Ageing Society, pp. 3-9, 2019.
- [2] Tokyo Fire Department, “Accidents by type,” Actual Situation of Daily Life Accidents From Emergency Transport Data, pp. 21-37, 2019.
- [3] M. F. Antwi-Afari, H. Li, J. Seo, and A. Y. L. Wong, “Wearable insole pressure system for automated detection and classification of awkward working postures in construction workers,” Automation in Construction, Vol.96, pp. 189-199, 2018.
- [4] S. Lee, S. T. Cho, and S. Choi, “Classification of Gait Type Based on Deep Learning Using Various Sensors with Smart Insole,” Sensors, Vol.19, No.8, 1757, 2019.
- [5] A. B. Putti, G. P. Arnold, L. Cochrane, and R. J. Abboud, “The Pedar® in-shoe system: Repeatability and normal pressure values,” Gait & Posture, Vol.25, pp. 401-405, 2007.
- [6] S. W. Park, P. S. Das, and J. Y. Park, “Development of wearable and flexible insole type capacitive pressure sensor for continuous gait signal analysis,” Organic Electronics, Vol.53, pp. 213-220, 2018.
- [7] Q. Zhang, Y. L. Wang, Y. Xia, X. Wu, T. V. Kirk, and X. D. Chen, “A low-cost and highly integrated sensing insole for plantar pressure measurement,” Sensing and Bio-Sensing Research, Vol.26, 100298, 2019.
- [8] I. P. I. Pappas, M. R. Popovic, T. Keller, V. Dietz, and M. Morari, “A Reliable Gait Phase Detection System,” IEEE Trans. on Neural Systems and Rehabilitation Engineering, Vol.9, pp. 113-125, 2001.
- [9] P. H. Truong, J. Lee, A. Kwon, and G. Jeong, “Stride Counting in Human Walking and Walking Distance Estimation Using Insole Sensors,” Sensors, Vol.16, No.6, 823, 2016.
- [10] M. F. Antwi-Afari, H. Li, Y. Yu, and L. Kong, “Automated detection and classification of construction workers’ loss of balance events using wearable insole pressure sensors,” Automation in Construction, Vol.96, pp. 189-199, 2018.
- [11] Y. Hayakawa, S. Kawanaka, K. Kanezaki, K. Minami, and S. Doi, “Study on Presentation System for Walking Training using High-Performance Shoes,” J. Robot. Mechatron, Vol.27, No.6, pp. 706-713, 2015.
- [12] T. Mori, M. Hamatani, H. Noguchi, M. Oe, and H. Sanada, “Insole-Type Simultaneous Measurement System of Plantar Pressure and Shear Force During Gait for Diabetic Patients,” J. Robot. Mechatron, Vol.24, No.5, pp. 766-772, 2012.
- [13] P. Jacqueline, “Gait Analysis: Normal and Pathological Function (2nd Ed.),” Slack Inc., pp. 2-47, 2007.
- [14] K. Ueda, M. Tamaki, and K. Yasumoto, “A System for Daily Living Activities Recognition Based on Multiple Sensing Data in a Smart Home,” Proc. of Multimedia, Distributed, Cooperative, and Mobile Symp. (DICOMO2014), pp. 1884-1891, 2014 (in Japanese).
- [15] F. Pedregosa, G. Varoquaux, A. Gramfort, V. Michel, B. Thirion, O. Grisel, M. Blondel, P. Prettenhofer, R. Weiss, V. Dubourg et al., “Scikit-learn: Machine Learning in Python,” J. of Machine Learning Re-Search, Vol.12, pp. 2825-2830, 2011.
- [16] J. Grus, “Data Science from Scratch,” O’Reilly, 2017.
This article is published under a Creative Commons Attribution-NoDerivatives 4.0 Internationa License.