Paper:
Market Forecasting by Variable Selection of Indicators and Emotion Scores from Text Data
Eri Domoto*, Koji Okuhara**, and Antonio Oliveira Nzinga Rene**
*Department of Media Business, Faculty of Media Business, Hiroshima University of Economics
5-37-1 Gion, Asaminami, Hiroshima-shi, Hiroshima 731-0192, Japan
**Department of Information Systems Engineering, Graduate School of Engineering, Toyama Prefectural University
5180 Kurokawa, Imizu, Toyama 939-0398, Japan
The size of financial markets has become huge, research on mechanisms is becoming more critical, and research is progressing. In addition to research on financial market modelling, there have been increasing attempts to clarify the mechanism of the Financial Markets Commission by associating markets with events that occur outside the market. Therefore, in this study, we investigate the effects of factors outside the market on exchange rates and consider the mechanisms necessary to consider the effects of automatic trading. We propose an analysis method for automatic trading of foreign exchange that considers external and internal factors in the market.
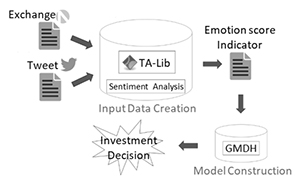
Construction system flow
- [1] H. T. P. Thanh and P. Meesad, “Stock Market Trend Prediction Based on Text Mining of Corporate Web and Time Series Data,” J. Adv. Comput. Intell. Intell. Inform., Vol.18, No.1, pp. 22-31, 2014.
- [2] K. Izumi, T. Goto, and T. Matsui, “Analysis of Financial Markets’ Fluctuation by Textual Information,” Trans. of the Japanese Society for Artificial Intelligence, Vol.25, Issue 3, pp. 383-387, 2010.
- [3] R. Inaishi, K. Toya, F. Zhai, and E. Kita, “Effect of Overconfident Investor Behavior to Stock Market,” J. Adv. Comput. Intell. Intell. Inform., Vol.14, No.6, pp. 661-668, 2010.
- [4] M. Ito, “On the controversy over the efficient market hypothesis,” Keio J. of Economics, Vol.100, No.3, pp. 793-813, 2007.
- [5] S. Basu, “Investment performance of common stocks in relation to their price-earnings ratios: A test of the efficient market hypothesis,” The J. of Finance, Vol.32, No.3, 1977.
- [6] R. Inaishi, F. Zhai, and E. Kita, “Effect of Overconfident Investor to Stock Market Behaviour,” Information Processing Society of Japan, Vol.2, No.2, pp. 10-21, 2009.
- [7] Y. Yamamoto, T. Kumamoto, and A. Nadamoto, “Determining the Twitter emotional axis based on the emotional relationship of tweets,” DEIM Forum 2015, F5-2, 2015 (in Japanese).
- [8] S. Nagaya, Z. Chenli, and O. Hasegawa, “A Proposal of Stock Price Predictor Using Associated Memory,” J. Adv. Comput. Intell. Intell. Inform., Vol.15, No.2, pp. 145-155, 2011.
- [9] L. J. Bettman, S. J. Sault, and E. L. Schultz, “Fundamental and technical a analysis: substitutes or complement,” Accounting and Finance, Vol.49, No.1, pp. 21-36, 2009.
- [10] T. Liu, N. Qiu, and W. Gu, “A Stock Trading Strategy Based on Time-Varying Quantile Theory,” J. Adv. Comput. Intell. Intell. Inform., Vol.19, No.3, pp. 417-422, 2015.
- [11] J. Bollen, H. Mao, and X. Zeng, “Twitter mood predicts the stock market,” J. of Computational Science, Vol.2, No.1, pp. 1-8, 2011.
- [12] K. Maruyama, E. Umehara, H. Suwa, and T. Ohta, “Is Content of Stock BBS related to the Stock Index?,” The Japanese Society for Artificial Intelligence Technical Report, SIG-FIN-002-09, 2008.
- [13] J. Paparrizos and L. Gravano, “k-Shape: Efficient and Accurate Clustering of Time Series,” ACM SIGMOD Record, Vol.45, No.1, pp. 69-76, 2016.
- [14] D. Gruhl, R. Guha, R. Kumar, J. Novak, and A. Tomkins, “The predictive power of online chatter,” Proc. of the eleventh ACM SIGKDD Int. Conf. on Knowledge Discovery in Data Mining, 2005.
- [15] A. Ohnishi, K. Mori, K. Kohriyama, M. Miyata, Y. Murai, and M. Ikeda, “Japanese Translation of Profile of Mood States (POMS) – Interpretation of the Fifty Six Items of Mood Factors and Their Application in a Manufacturing Automotive Parts Factory –,” J. of The University of Occupational and Environmental Health, Vol.15, No.2, pp. 147-154, 1993.
- [16] J. Pennington, R. Socher, and C. D. Manning, “GloVe: Global Vectors for Word Representation,” Proc. of the 2014 Conf. on Empirical Methods in Natural Language Processing (EMNLP), pp. 1532-1543, 2014.
- [17] N. Colneric and J. Demsar, “Emotion Recognition on Twitter: Comparative Study and Training a Unison Model,” IEEE Trans. on Affective Computing, pp. 1-14, 2018.
- [18] T. Kondo, “Revised GMDH Algorithm Using Principal Component-Regression Analysis,” Trans. of the Institute of Systems, Control and Information Engineers, Vol.5, No.10, pp. 391-399, 1992.
- [19] T. Kondo, J. Ueno, and K. Kondo, “Revised GMDH-Type Neural Networks Using AIC or PSS Criterion and Their Application to Medical Image Recognition,” J. Adv. Comput. Intell. Intell. Inform., Vol.9, No.3, pp. 257-267, 2005.
- [20] T. Kondo and J. Ueno, “Logistic GMDH-Type Neural Network and its Application to Identification of X-Ray Film Characteristic Curve,” J. Adv. Comput. Intell. Intell. Inform., Vol.11, No.3, pp. 312-318, 2007.
- [21] T. Kondo, J. Ueno, and A. S. Pandya, “Multilayered GMDH-Type Neural Network with Radial Basis Functions and its Application to 3-Dimensional Medical Image Recognition of the Liver,” J. Adv. Comput. Intell. Intell. Inform., Vol.11, No.1, pp. 96-104, 2007.
This article is published under a Creative Commons Attribution-NoDerivatives 4.0 Internationa License.