Paper:
Interval Data Envelopment Analysis for Inter-Group Data Usage
Tomoe Entani
University of Hyogo
7-1-28 Minatojima-Minamimachi, Chuo, Kobe 650-0047, Japan
Organizations are interested in exploiting the data from the other organizations for better analyses. Therefore, the data related policies of organizations should be sensitive to the data privacy issue, which has been widely discussed recently. The present study is focused on inter-group data usage for a relative evaluation. This research is based on the data envelopment analysis (DEA), which is used to measure the efficiency of a decision making unit (DMU) relatively employed within a group. In DEA, establishing an efficient frontier consisting of efficient DMUs is essential. We can obtain the efficiency values of a DMU by projecting it to the efficient frontier, and including in the efficiency interval via the interval DEA. When the original data of multiple groups are not open to each other, the alternative is to exchange the information corresponding to the efficient frontiers to estimate the efficiency intervals of a DMU in such a manner that the alternative is in the other groups. Therefore, in this paper, we propose a method to replace the efficient frontier with a weight vector set, from which it is not possible to reconstruct the original data. Considering the weight vector sets of multiple groups, a DMU has three types of efficiency intervals: in its own group, in each of the other groups, and in the integrated group. They provide rich insights on the DMU from a broad perspective, and this encourages inter-group data usage. In this process, we focus on two types of information reduction: one is from the efficient frontier to the weight vector set, and the other is from a union of the groups to the integrated group.
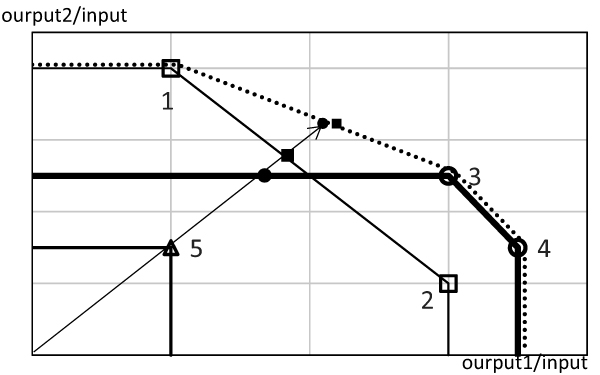
- [1] A. Charnes, W. W. Cooper, and E. Rhodes, “Measuring the efficiency of decision making units,” European J. of Operational Research, Vo.2, No.6, pp. 429-444, 1978.
- [2] M. J. Rezaeiani and A. A. Foroughi, “Ranking efficient decision making units in data envelopment analysis based on reference frontier share,” European J. of Operational Research, Vol.264, No.2, pp. 665-674, 2018.
- [3] W. D. Cook, J. L. Ruiz, I. Sirvent, and J. Zhu, “Within-group common benchmarking using DEA,” European J. of Operational Research, Vol.256, No.3, pp. 901-910, 2017.
- [4] T. Entani, Y. Maeda, and H. Tanaka, “Dual models of interval DEA and its extension to interval data,” European J. of Operational Research, Vol.136, No.1, pp. 32-45, 2002.
- [5] P. Bogetoft and L. Kromann, “Evaluating treatment effects using data envelopment analysis on matched samples: An analysis of electronic information sharing and firm performance,” European J. of Operational Research, Vol.270, No.1, pp. 302-313, 2018.
- [6] V. Torra and G. Navarro-Arribas, “Big Data Privacy and Anonymization,” pp. 15-26, Springer, 2016.
- [7] J. Henriksen-Bulmer, S. Faily, and S. Jeary, “Privacy risk assessment in context: A meta-model based on contextual integrity,” Computers & Security, Vol.82, pp. 270-283, 2019.
- [8] P. J. Agrell and R. E. Steuer, “ACADEA-Decision Support System for Faculty Performance Reviews,” J. of Multi-Criteria Decision Analysis, Vol.9, No.5, pp. 191-204, 2000.
- [9] J. Aparicio, E. Crespo-Cebada, F. Pedraja-Chaparro, and D. Santín, “Comparing school ownership performance using a pseudo-panel database: A Malmquist-type index approach,” European J. of Operational Research, Vol.256, No.2, pp. 533-542, 2017.
- [10] A. S. Camanho and R. G. Dyson, “Data envelopment analysis and Malmquist indices for measuring group performance,” J. of Productivity Analysis, Vol.26, No.1, pp. 35-49, 2006.
- [11] V. V. Podinovski, “Optimal weights in DEA models with weight restrictions,” European J. of Operational Research, Vol.254, No.3, pp. 916-924, 2016.
- [12] C. Kao, “Efficiency measurement and frontier projection identification for general two-stage systems in data envelopment analysis,” European J. of Operational Research, Vol.261, No.2, pp. 679-689, 2017.
- [13] T. Entani, “Measuring Efficiency Intervals in Multiple Groups with Privacy Concerns,” Proc. of The 6th Int. Symp. on Integrated Uncertainty in Knowledge Modelling and Decision Making, pp. 48-59, 2018.
This article is published under a Creative Commons Attribution-NoDerivatives 4.0 Internationa License.