Paper:
SAR Target Recognition via Joint Classification of Monogenic Components with Discrimination Analysis
Zhenyu Zhang
School of Automation and Electrical Engineering, Zhejiang University of Science and Technology
No.318 Liuhe Road, Hangzhou, Zhejiang 310023, China
This paper proposes a method using joint classification of monogenic components with discrimination analysis for target recognition in synthetic aperture radar (SAR) images. Three monogenic components, namely, phase, amplitude, and orientation, are extracted from the original image and classified by joint sparse representation for target recognition. Considering that the three components may have different discrimination capabilities for different operating conditions, the discrimination analysis is incorporated into the classification scheme. The components with low discriminability are not used in the joint classification. Afterwards, those discriminative components for a certain condition are classified to determine the target type. Experiments are conducted on the moving and stationary target acquisition and recognition (MSTAR) to evaluate the performance of the proposed method.
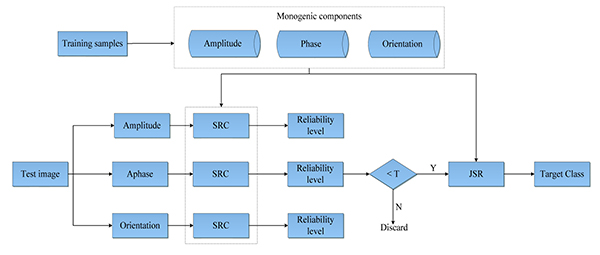
The procedure of the proposed method
- [1] K. El-Darymli, E. W. Gill, P. Mcguire, et al., “Automatic target recognition in synthetic aperture radar imagery: a state-of-the-art review,” IEEE Access, Vol.4, pp. 6014-6058, 2016.
- [2] J. Park, S. Park, and K. Kim, “New discrimination features for SAR automatic target recognition,” IEEE Geosci. Remote Sens. Lett., Vol.10, No.3, pp. 476-480, 2013.
- [3] M. Amoon and G.-A. Rezai-Rad, “Automatic target recognition of synthetic aperture radar (SAR) images based on optimal selection of Zernike moment features,” IET Comput. Vis., Vol.8, No.2, pp. 77-85, 2014.
- [4] B. Ding, G. Wen, C. Ma, et al., “Target recognition in synthetic aperture radar images using binary morphological operations,” J. Appl. Remote Sens., Vol.10, No.4, 046006, 2016.
- [5] A. K. Mishra, “Validation of PCA and LDA for SAR ATR,” Proc. IEEE TENCON, pp. 1-6, 2008.
- [6] Z. Cui, Z. Cao, J. Yang, et al., “Target recognition in synthetic aperture radar via non-negative matrix factorization,” IET Radar Sonar Navig., Vol.9, No.9, pp. 1376-1385, 2015.
- [7] B. Ding, G. Wen, J. Zhong, et al., “Robust method for the matching of attributed scattering centers with application to synthetic aperture radar automatic target recognition,” J. Appl. Remote Sens., Vol.10, No.1, 016010, 2016.
- [8] B. Ding, G. Wen, J. Zhong, et al., “A robust similarity measure for attributed scattering center sets with application to SAR ATR,” Neurocomputing, Vol.219, pp. 130-143, 2017.
- [9] G. Dong, G. Kuang, N. Wang, et al., “SAR target recognition via joint sparse representation of monogenic signal,” IEEE J. of Selected Topics in Applied Earth Observation and Remote Sensing, Vol.8, No.7, pp. 3316-3328, 2015.
- [10] G. Dong and G. Kuang, “Classification on the monogenic scale space: application to target recognition in SAR image,” IEEE Trans. on Image Processing, Vol.24, No.8, pp. 2527-2529, 2015.
- [11] J. Wright, A. Yang, A. Ganesh, S. S. Sastry, and Y. Ma, “Robust face recognition via sparse representation,” IEEE Trans. Pattern Anal. Mach. Intell., Vol.31, No.2, pp. 210-227, 2008.
- [12] J. J. Thiagaraian, K. N. Ramamurthy, P. Knee, A. Spanias, and V. Berisha, “Sparse representations for automatic target classification in SAR images,” Proc. 4th Int. Symp. Commun., Control and Signal Processing, pp. 1-4, 2010.
- [13] J. A. Tropp, A. C. Gilbert, and M. J. Strauss, “Algorithms for simultaneous sparse approximation, Part I: Greedy pursuit,” Signal Processing, Vol.86, Issue 3, pp. 572-588, 2006.
- [14] S. Ji, D. Dunson, and L. Carin, “Multitask compressive sensing,” IEEE Trans. Signal Process., Vol.57, No.1, pp. 92-106, 2009.
- [15] Q. Zhao and J. C. Principe, “Support Vector Machines for SRA Target Recognition,” IEEE Trans. Aerosp. Electron. Syst., Vol.37, No.2, pp. 643-654, 2001.
- [16] S. Chen, H. Wang, F. Xu, and Y. Jin, “Target classification using the deep convolutional networks for SAR images,” IEEE Trans. on Geoscience and Remote Sensing, Vol.54, No.8, pp. 4806-4817, 2016.
- [17] S. Doo, G. Smith, and C. Baker, “Target classification performance as a function of measurement uncertainty,” Proc. 5th Asia-Pacific Conf. on Synthetic Aperture Radar (APSAR), pp. 587-590, 2015.
- [18] B. Ding and G. Wen, “Exploiting multi-view SAR images for robust target recognition,” Remote Sensing, Vol.9, No.11, p. 1150, 2017.
This article is published under a Creative Commons Attribution-NoDerivatives 4.0 Internationa License.