Short Paper:
High-Speed Serial Data Transmission Error Control Based on Fuzzy Classification
Zhimin Zhang
College of Applied Technology, Xi’an International University
North Campus, Middle section of Fuyu Road, Yanta District, Xi’an City, Shaanxi 710077, China
At present, the error control method for high-speed serial data transmission obtains the errors by comparison and then controls them. If the data transmission channel is not denoised, the packet loss and error codes become serious, and energy consumption increases. The use of fuzzy classification is proposed to control data transmission errors. The method uses the combination of wavelet transform and transform domain difference to double denoise the channel, and it completes the clustering of data transmission errors by fuzzy classification. Considering packet loss, error codes, and energy consumption in data transmission error control, when the communication distance between two nodes is small, automatic repeat request is used to control data transmission errors. As the distance between nodes increases, forward error correction is used to control data transmission errors. When the communication distance gradually increases, data transmission errors are controlled by hybrid automatic repeat request. Experiments showed that the proposed method can reduce the data transmission error, control energy consumption, packet loss rate, and bit error rate, and enhance the denoising effect.
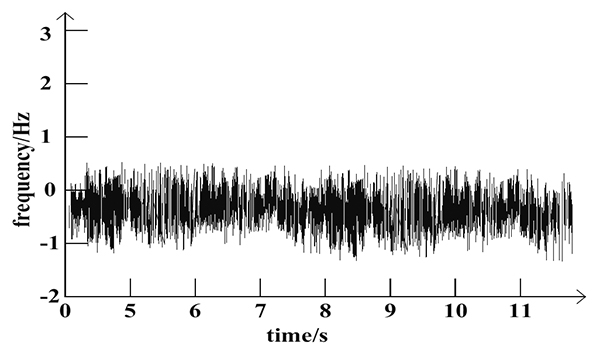
The proposed method improved the channel denoising effect and data transmission error control accuracy
- [1] G. Yang, W. Tan, H. Jin, T. Zhao, and L. Tu, “Review wearable sensing system for gait recognition,” Cluster Computing, pp. 1-9, 2018.
- [2] N. Akkari, J. M. Jornet, P. Wang, E. Fadel, L. Elrefaei, M. G. A. Malik, S. Almasri, and I. F. Akyildiz, “Joint physical and link layer error control analysis for nanonetworks in the Terahertz band,” Wireless Networks, Vol.22, No.4, pp. 1221-1233, 2016.
- [3] N. H. Tran, L. J. Rodríguez, and T. Le-Ngoc, “Optimal Power Control and Error Performance for Full-Duplex Dual-Hop AF Relaying Under Residual Self-Interference,” IEEE Communications Letters, Vol.19, No.2, pp. 291-294, 2015.
- [4] H. Q. Xiao, Y. He, M. Wu, and S.-P. Xiao, “New Results on H∞ Tracking Control for Sampled-Data Networked Control System in T–S Fuzzy Model,” IEEE Trans. on Fuzzy Systems, Vol.23, No.6, pp. 2439-2448, 2015.
- [5] N. T. Kuong, E. Uchino, and N. Suetake, “IVUS Tissue Characterization of Coronary Plaque by Classification Restricted Boltzmann Machine,” J. Adv. Comput. Intell. Intell. Inform., Vol.21, No.1, pp. 67-73, 2017.
- [6] G. Yang, Y. Zhang, J. Yang, G. Ji, Z. Dong, S. Wang, C. Feng, and Q. Wang, “Automated classification of brain images using wavelet-energy and biogeography-based optimization,” Multimedia Tools and Applications, Vol.75, No.23, pp. 15601-15617, 2016.
- [7] Y. Li, J. Peng, F. Jiang, K. Liu, and X. Zhang, “Joint Spectrum Sensing and Data Transmission Optimization for Energy Efficiency in Cognitive Radio Sensor Networks: A Dynamic Cooperative Method,” J. Adv. Comput. Intell. Intell. Inform., Vol.19, No.2, pp. 197-204, 2015.
This article is published under a Creative Commons Attribution-NoDerivatives 4.0 Internationa License.