Paper:
Adaptive Fertigation System Using Hybrid Vision-Based Lettuce Phenotyping and Fuzzy Logic Valve Controller Towards Sustainable Aquaponics
Ronnie S. Concepcion II*,, Sandy C. Lauguico*, Jonnel D. Alejandrino*, Argel A. Bandala*, Edwin Sybingco*, Ryan Rhay P. Vicerra**, Elmer P. Dadios**, and Joel L. Cuello***
*Electronics and Communications Engineering Department, De La Salle University
2401 Taft Avenue, Ermita, Manila 1004, Philippines
**Manufacturing Engineering and Management Department, De La Salle University
2401 Taft Avenue, Ermita, Manila 1004, Philippines
***Agricultural and Biosystems Engineering Department, University of Arizona
Tucson, Arizona, USA
Corresponding author
Sustainability is a major challenge in any plant factory, particularly those involving precision agriculture. In this study, an adaptive fertigation system in a three-tier nutrient film technique aquaponic system was developed using a non-destructive vision-based lettuce phenotype (VIPHLET) model integrated with an 18-rule Mamdani fuzzy inference system for nutrient valve control. Four lettuce phenes, that is, fresh weight, chlorophylls a and b, and vitamin C concentrations as outputted by the genetic programming-based VIPHLET model were optimized for each growth stage by injecting NPK nutrients into the mixing tank, as determined based on leaf canopy signatures. This novel adaptive fertigation system resulted in higher nutrient use efficiency (99.678%) and lower chemical waste emission (14.108 mg L-1) than that by manual fertigation (92.468%, 178.88 mg L-1). Overall, it can improve agricultural malpractices in relation to sustainable agriculture.
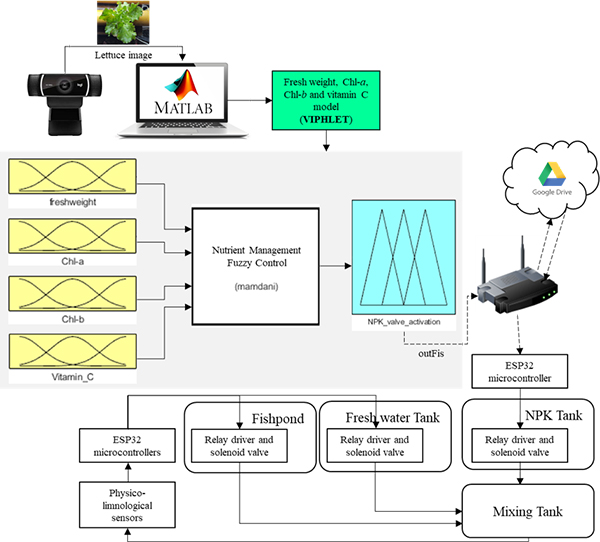
VIPHLET-fuzzy control for fertigation
- [1] E. P. Dadios and J. Ashraf, “Genetic algorithm with adaptive and dynamic penalty functions for the selection of cleaner production measures: A constrained optimization problem,” Clean Technol. Environ. Policy, Vol.8, No.2, pp. 85-95, 2006.
- [2] A. de Gelder, E. Heuvelink, and J. J. G. Opdam, “Tomato yield in a closed greenhouse and comparison with simulated yields in closed and conventional greenhouses,” Int. Conf. on Sustainable Greenhouse Systems (Greensys2004), Acta Horticulturae, No.691, pp. 549-552, 2005.
- [3] S. J. Seidel et al., “Towards improved calibration of crop models – Where are we now and where should we go?,” Eur. J. Agron., Vol.94, pp. 25-35, 2018.
- [4] M. I. Schiattone et al., “Impact of irrigation regime and nitrogen rate on yield, quality and water use efficiency of wild rocket under greenhouse conditions,” Sci. Hortic., Vol.229, pp. 182-192, 2018.
- [5] G. Conversa et al., “A decision support system (GesCoN) for managing fertigation in vegetable crops. Part II – model calibration and validation under different environmental growing conditions on field grown tomato,” Front. Plant Sci., Vol.6, Article No.495, 2015.
- [6] L. Cimó et al., “Doses de Fosforo na Fertirrigacao de Mudas em Substratos a base de fibra de coco e Influencia na Producao do Repolho,” Irrig. Botucatu, Vol.22, No.1, pp. 34-43, 2017 (in Portuguese with English abstract).
- [7] J. V. Prado-Hernández et al., “Lettuce (Lactuca sativa) crop production using drip irrigation installed at different depths,” Int. Horticultural Congress (IHC2018), Acta Horticulturae, No.1253, pp. 183-190, 2019.
- [8] O. L. Vargas et al., “Irrigation and fertigation with drip and alternative micro irrigation systems in northern highbush blueberry,” HortScience, Vol.50, No.6, pp. 897-903, 2015.
- [9] R. E. Abdelraouf et al., “Influence of N-fertigation stress and agro-organic wastes (biochar) to improve yield and water productivity of sweet pepper under sandy soils conditions,” Plant Arch., Vol.20, No.1, pp. 3208-3217, 2020.
- [10] M. K. I. A. Rahman et al., “Enhanced fertigation control system towards higher water saving irrigation,” Indones. J. Electr. Eng. Comput. Sci., Vol.10, No.3, pp. 859-866, 2018.
- [11] P. Garcia-Caparros et al., “Integral management of irrigation water in intensive horticultural systems of Almería,” Sustain., Vol.9, No.12, pp. 1-21, 2017.
- [12] A. J. Steidle Neto, S. Zolnier, and D. de Carvalho Lopes, “Development and evaluation of an automated system for fertigation control in soilless tomato production,” Comput. Electron. Agric., Vol.103, pp. 17-25, 2014.
- [13] P. Visconti et al., “Development of sensors-based agri-food traceability system remotely managed by a software platform for optimized farm management,” Sensors, Vol.20, No.13, pp. 1-43, 2020.
- [14] D. Savvas and N. Gruda, “Application of soilless culture technologies in the modern greenhouse industry – A review,” Eur. J. Hortic. Sci., Vol.83, No.5, pp. 280-293, 2018.
- [15] D. Allen Pattillo et al., “Performance of aquaculture effluent for tomato production in outdoor raised beds,” Horttechnology, Vol.30, No.5, pp. 624-631, 2020.
- [16] F. Kimera et al., “Efficient utilization of aquaculture effluents to maximize plant growth, yield, and essential oils composition of Origanum majorana cultivation,” Ann. Agric. Sci., Vol.66, No.1, pp. 1-7, 2021.
- [17] M. M. Maboko and C. P. Du Plooy, “Response of hydroponically grown cherry and fresh market tomatoes to reduced nutrient concentration and foliar fertilizer application under shadenet conditions,” HortScience, Vol.52, No.4, pp. 572-578, 2017.
- [18] M. Gallardo, A. Elia, and R. B. Thompson, “Decision support systems and models for aiding irrigation and nutrient management of vegetable crops,” Agric. Water Manag., Vol.240, Article No.106209, 2020.
- [19] Y. Othman et al., “Soilless culture: Management of growing substrate, water, nutrient, salinity, microorganism and product quality,” Fresenius Environ. Bull., Vol.28, No.4A, pp. 3249-3260, 2019.
- [20] C. L. McCarthy, N. H. Hancock, and S. R. Raine, “Applied machine vision of plants: A review with implications for field deployment in automated farming operations,” Intell. Serv. Robot., Vol.3, pp. 209-217, 2010.
- [21] S. C. Lauguico et al., “Implementation of Inverse Kinematics for Crop-Harvesting Robotic Arm in Vertical Farming,” Proc. of the IEEE 2019 9th Int. Conf. on Cybernetics and Intelligent Systems (CIS) and Robotics, Automation and Mechatronics (RAM), 2019.
- [22] R. S. Concepcion II et al., “Lettuce growth stage identification based on phytomorphological variations using coupled color superpixels and multifold watershed transformation,” Int. J. Adv. Intell. Informatics, Vol.6, No.3, pp. 261-277, 2020.
- [23] S. C. Lauguico et al., “Lettuce life stage classification from texture attributes using machine learning estimators and feature selection processes,” Int. J. Adv. Intell. Informatics, Vol.6, No.2, p. 173, 2020.
- [24] J. Alejandrino et al., “Visual classification of lettuce growth stage based on morphological attributes using unsupervised machine learning models,” 2020 IEEE Region 10 Conf. (TENCON), pp. 438-443, 2020.
- [25] C. J. T. Dinio et al., “Automated Water Source Scheduling System with Flow Control System,” 2018 IEEE 10th Int. Conf. on Humanoid, Nanotechnology, Information Technology,Communication and Control, Environment and Management (HNICEM), 2018.
- [26] R. Concepcion II, E. Dadios, and J. Cuello, “Non-destructive in Situ Measurement of Aquaponic Lactuca Sativa Leaf Photosynthetic Pigments and Nutrient Concentrations Using Hybrid Decision Tree and Multigene Symbolic Genetic Programming,” AGRIVITA, J. Agric. Sci. (accepted).
- [27] R. Concepcion et al., “Genetic algorithm-based visible band tetrahedron greenness index modeling for lettuce biophysical signature estimation,” 2020 IEEE Region 10 Conf. (TENCON), pp. 679-684, 2020.
This article is published under a Creative Commons Attribution-NoDerivatives 4.0 Internationa License.