single-award.php
JACIII Best Paper and Young Researcher Awards 2021
![]() |
JACIII BEST PAPER AWARD 2021 Self-Structured Cortical Learning Algorithm by Dynamically Adjusting Columns and Cells Sotetsu Suzugamine, Takeru Aoki, Keiki Takadama, and Hiroyuki Sato |
![]() | ![]() | ![]() | ![]() | |
Sotetsu Suzugamine Graduate School of Information and Engineering Sciences, The University of Electro-Communications | Takeru Aoki Graduate School of Information and Engineering Sciences, The University of Electro-Communications | Keiki Takadama Graduate School of Information and Engineering Sciences, The University of Electro-Communications | Hiroyuki Sato Graduate School of Information and Engineering Sciences, The University of Electro-Communications |
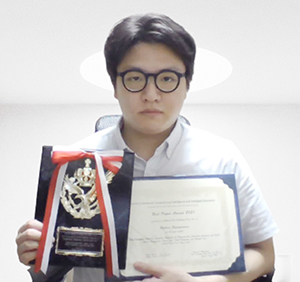
Fig. Mr. Sotetsu Suzugamine received a plaque and a certificate of the JACIII Best Paper Award 2021 on behalf of the authors.
Message from the Winner
I am very honored to receive the JACIII Best Paper Award 2021. I would like to express my sincere gratitude to the editorial board, reviewers, and everyone else who supported this study.
This paper, “Self-Structured Cortical Learning Algorithm by Dynamically Adjusting Columns and Cells,” is a study of the cortical learning algorithm (CLA). CLA is a time-series data prediction algorithm based on the human neocortex, the most important part of human intelligence. In this paper, we propose a method of dynamically increasing or decreasing self-structures, which are called columns and cells and which correspond to human neurons, to adjust them depending on the input data. The proposed method eliminates the need for column and cell parameters, which were previously determined statically. Furthermore, the experimental results on a multistep prediction of real-world power consumption show that the proposed self-structured CLA achieves high prediction accuracy.
Once again, I would like to express my gratitude to everyone involved in publishing this paper and making it possible for me to receive this award. I wish for the future development of scientific research in the field of computational intelligence and intelligent informatics.
![]() |
JACIII YOUNG RESEARCHER AWARD 2021 Emotion Recognition Based on Multi-Composition Deep Forest and Transferred Convolutional Neural Network Xiaobo Liu, Xu Yin, Min Wang, Yaoming Cai, and Guang Qi |
![]() | ![]() | ![]() | ![]() | ![]() | |
Xiaobo Liu School of Automation, China University of Geosciences | Xu Yin School of Automation, China University of Geosciences | Min Wang School of Automation, China University of Geosciences | Yaoming Cai School of Computer Science, China University of Geosciences | Guang Qi School of Automation, China University of Geosciences |
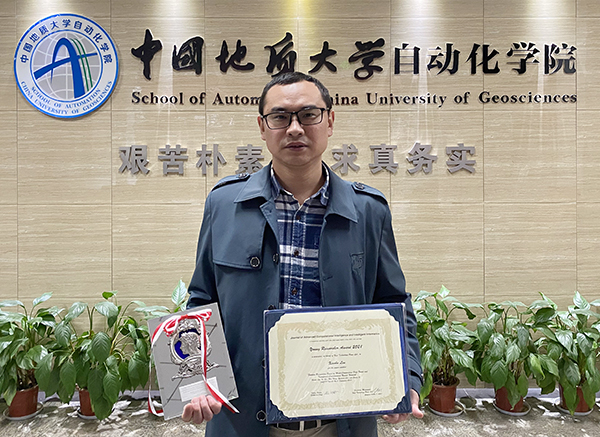
Fig. Dr. Xialbo Liu holding a plaque and a certificate of the JACIII Young Researcher Award 2021.
Message from the Winner
It is a great honor for me to receive the JACIII Young Researcher Award 2021. This award is the best form of praise for our work and will provide strong stimulation for our passion for research. We are deeply grateful to the JACIII editorial board/office, which made our paper easy to understand and gave it a beautiful layout. We are also grateful to the reviewers, who provided insightful comments that gave our paper more meaning and research value. Next, I wish to give my deepest thanks to my co-authors for their considerable contributions to emotion recognition based on multi-composition deep forest and transferred convolutional neural network. Our many discussions resulted in numerous rewrites, not to mention memories I will cherish forever.
We propose a novel emotion-recognition framework that uses a knowledge-transfer approach to capturing features, employing an improved, deep-forest model to determine the final emotions. The structure of a very deep, convolutional network is learned from ImageNet and is utilized to extract face and emotion features from other data sets, thereby solving the problem of insufficiently labeled samples. These features are then input into a classifier, “multi-composition deep forest,” which consists of 16 forests for facial emotion recognition, to enhance the diversity of the framework. The proposed method does not require that a network be trained with a complex structure, and the decision-tree-based classifier can achieve accurate results with very few parameters, making it easier to implement, train, and apply. Moreover, the classifier can adaptively decide the complexity of its model without iteratively updating parameters.
This truly interesting work is an indication of a new direction in facial emotion recognition. I hope that this paper promotes the development of both transfer learning and deep forest as well as related research, so that facial emotion recognition may find additional applications. The JACIII, the most popular journal of computational intelligence and intelligent informatics, is a powerful platform for sharing ideas and work, and I look forward to its continued improvement and success going forward.
![]() |
JACIII YOUNG RESEARCHER AWARD 2021 Object-Oriented 3D Semantic Mapping Based on Instance Segmentation Jinxin Chi, Hao Wu, and Guohui Tian |
![]() | ![]() | ![]() | |
Jinxin Chi School of Control Science and Engineering, Shandong University | Hao Wu School of Control Science and Engineering, Shandong University | Guohui Tian School of Control Science and Engineering, Shandong University |
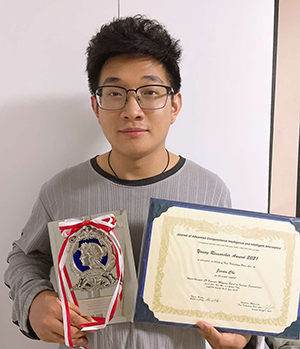
Fig. Mr. Jinxin Chi holding a plaque of the JACIII Young Researcher Award 2021.
Message from the Winner
I am very honored to receive the JACIII Young Researcher Award 2021. I am deeply grateful to the JACIII editorial office, reviewers, co-authors, and all supporters of this paper, “Object-Oriented 3D Semantic Mapping Based on Instance Segmentation.” This award is highly encouraging to my further study. My research interests include Visual SLAM and Robot Semantic Understanding. This paper proposes an object-oriented, 3D semantic mapping method that combines state-of-the-art, deep-learning-based Instance Segmentation and a Visual SLAM algorithm. The method helps robots not only gain navigation-oriented geometric information about the surrounding environment but also obtain individually-oriented attribute and location information about the objects. An object recognition and target association algorithm applied to continuous image frames is also proposed. The algorithm combines visual SLAM, which uses visual consistency between image frames to promote the results of object matching and recognition over continuous image frames, to improve the accuracy of object recognition. I hope that this study will contribute to the development of both Semantic Robot and Visual SLAM, and that Semantic Visual SLAM will be promoted and widely used in the future. I also wish for the development of the JACIII and the field of computational intelligence and intelligent informatics. Thank you.
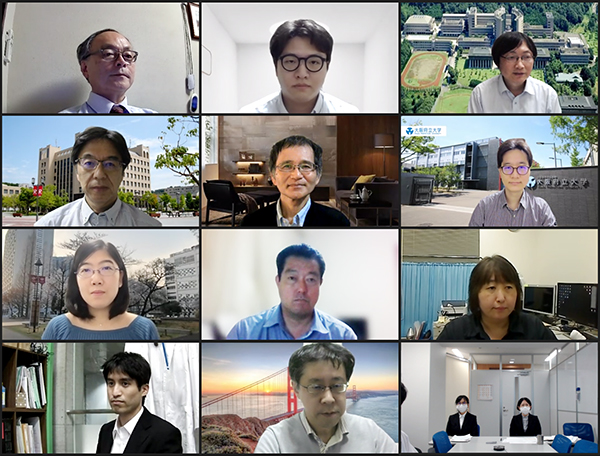
Fig. The winner of the Best Paper Award, editorial boards, and editorial staffs on the online ceremony. First row from left to right: Prof. Kaoru Hirota (Editor-in-Chief), Mr. Sotetsu Suzugamine (the BPA winner), and Prof. Jinhua She. Second: Prof. Yoichiro Maeda, Prof. Kiyohiko Uehara, and Dr. Seiki Ubukata. Third: Dr. Zhenni Pan, Prof. Tomohiro Yoshikawa, and Prof. Miho Ohsaki. Fourth: Dr. Hiroki Shibata, Prof. Kazuteru Miyazaki, and editorial staffs.