Paper:
Simultaneous Execution of Dereverberation, Denoising, and Speaker Separation Using a Neural Beamformer for Adapting Robots to Real Environments
Daichi Nagano and Kazuo Nakazawa
Faculty of Science and Technology, Keio University
3-14-1 Hiyoshi, Kohoku-ku, Yokohama, Kanagawa 223-8522, Japan
It remains challenging for robots to accurately perform sound source localization and speech recognition in a real environment with reverberation, noise, and the voices of multiple speakers. Accordingly, we propose “U-TasNet-Beam,” a speech extraction method for extracting only the target speaker’s voice from all ambient sounds in a real environment. U-TasNet-Beam is a neural beamformer comprising three elements: a neural network for removing reverberation and noise, a second neural network for separating the voices of multiple speakers, and a minimum variance distortionless response (MVDR) beamformer. Experiments with simulated data and recorded data show that the proposed U-TasNet-Beam can improve the accuracy of sound source localization and speech recognition in robots compared to the conventional methods in a noisy, reverberant, and multi-speaker environment. In addition, we propose the spatial correlation matrix loss (SCM loss) as a loss function for the neural network learning the spatial information of the sound. By using the SCM loss, we can improve the speech extraction performance of the neural beamformer.
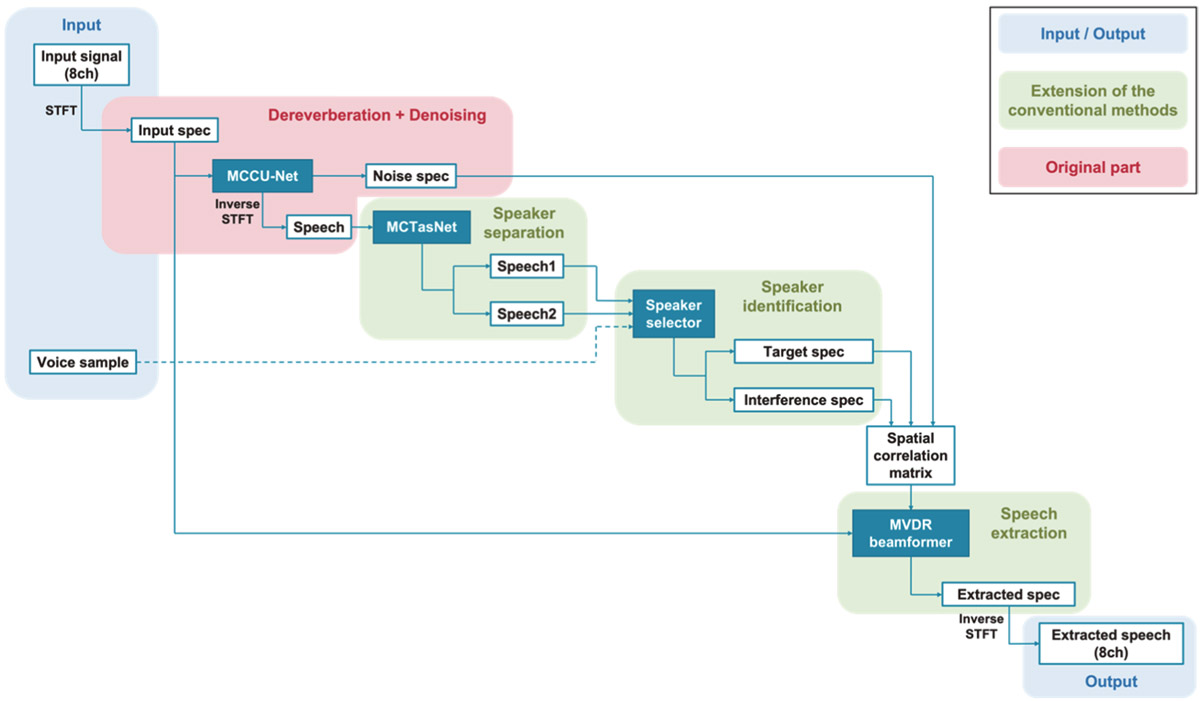
Schematic view of U-TasNet-Beam
- [1] O. Sugiyama, S. Uemura, A. Nagamine, R. Kojima, K. Nakamura, and K. Nakadai, “Outdoor acoustic event identification with DNN using a quadrotor-embedded microphone array,” J. Robot. Mechatron., Vol.29, No.1, pp. 188-197, 2017.
- [2] K. Sekiguchi, Y. Bando, K. Itoyama, and K. Yoshii, “Layout optimization of cooperative distributed microphone arrays based on estimation of sound source performance,” J. Robot. Mechatron., Vol.29, No.1, pp. 83-93, 2017.
- [3] D. D. Lee and H. S. Seung, “Learning the parts of objects by non-negative matrix factorization,” Nature, Vol.401, pp. 788-791, 1999.
- [4] A. Jansson, E. J. Humphrey, N. Montecchio, R. Bittner, A. Kumar, and T. Weyde, “Singing Voice Separation with Deep U-Net Convolutional Networks,” The 18th Int. Society for Music Information Retrieval Conf., 2017.
- [5] Y. Luo and N. Mesgarani, “Conv-TasNet: Surpassing Ideal Time-Frequency Magnitude Masking for Speech Separation,” IEEE/ACM Trans. on Audio, Speech, and Language processing, Vol.27, No.8, pp. 1256-1266, 2019.
- [6] C. Subakan, M. Ravanelli, S. Cornell, M. Bronzi, and J. Zhong, “Attention is all you need in speech separation,” IEEE Int. Conf. on Acoustics, Speech and Signal Processing, 2021.
- [7] M. Delcroix, K. Zmolikova, K. Kinoshita, A. Ogawa, and T. Nakatani, “Single channel target speaker extraction and recognition with speaker beam,” IEEE Int. Conf. on Acoustics, Speech and Signal Processing, pp. 5554-5558, 2018.
- [8] Q. Wang, H. Muckenhirn, K. Wilson, P. Sridhar, Z. Wu, J. Hershey, R. A. Saurous, R. J. Weiss, Y. Jia, and I. L. Moreno, “Voice-Filter: Targeted voice separation by speaker-conditioned spectrogram masking,” Proc. of Interspeech, pp. 2728-2732, 2019.
- [9] M. Souden, J. Benesty, and S. Affes, “On optimal frequency-domain multichannel linear filtering for noise reduction,” IEEE Trans. on Audio, Speech, Language Process., Vol.18, No.2, pp. 260-276, 2010.
- [10] E. Warsitz and R. Haeb-Umbach, “Blind acoustic beamforming based on generalized eigenvalue decomposition,” IEEE Trans. on Audio, Speech, Language Process, Vol.15, No.5, pp. 1529-1539, 2007.
- [11] P. Comon, “Independent component analysis, a new concept?,” Signal Processing, Vol.36, No.3, pp. 287-314, 1994.
- [12] D. Kitamura, N. Ono, H. Sawada, H. Kameoka, and H. Saruwatari, “Determined blind source separation unifying independent vector analysis and nonnegative matrix factorization,” IEEE/ACM Trans. on Audio, Speech and Language Processing, Vol.24, No.9, pp. 1626-1641, 2016.
- [13] J. Heymann, L. Drude, and R. Haeb-Umbach, “Neural network based spectral mask estimation for acoustic beamforming,” IEEE Int. Conf. on Acoustics, Speech, and Signal Processing, pp. 196-200, 2016.
- [14] W. Jiang, F. Wen, and P. Liu, “Robust beamforming for speech recognition using DNN-based time-frequency masks estimation,” IEEE Access, Vol.6, pp. 52385-52392, 2018.
- [15] T. Ochiai, M. Delcroix, R. Ikeshita, K. Kinoshita, T. Nakatani, and S. Araki, “Beam-Tasnet: Time-domain audio separation network meets frequency-domain beamformer,” IEEE Int. Conf. on Acoustics, Speech and Signal Processing, pp. 6384-6388, 2020.
- [16] R. Schmidt, “Multiple emitter location and signal parameter estimation,” IEEE Trans. on Antennas and Propagation, Vol.34, No.3, pp. 276-280, 1986.
- [17] H. S. Choi, J. H. Kim, J. Huh, A. Kim, J. W. Ha, and K. Lee, “Phase-aware speech enhancement with deep complex u-net,” Int. Conf. on Learning, Representations, 2018.
- [18] D. Yu, M. Kolbæk, Z.-H. Tan, and J. Jensen, “Permutation Invariant Training of Deep Models for Speaker-Independent Multi-talker Speech Separation,” IEEE Int. Conf. on Acoustics, Speech and Signal Processing, pp. 241-245, 2017.
- [19] L. Wan, Q. Wang, A. Papir, and I. L. Moreno, “Generalized end-to-end loss for speaker verification,” IEEE Int. Conf. on Acoustics, Speech and Signal Processing, pp. 4879-4888, 2018.
- [20] K. Wilson, M. Chinen, J. Thorpe, B. Patton, J. Hershey, A. R. Saurous, J. Skoglund, and F. R. Lyon, “Exploring tradeoffs in models for low-latency speech enhancement,” The 16th Int. Workshop on Acoustic Signal Enhancement, 2018.
- [21] D. Rethage, J. Pons, and X. Serra, “A wavenet for speech denoising,” IEEE Int. Conf. on Acoustics, Speech and Signal Processing, 2018.
- [22] J. Zhang, C. Zorila, R. Doddipatla, and J. Barker, “On end-to-end multi-channel time domain speech separation in reverberant environments,” IEEE Int. Conf. on Acoustics, Speech and Signal Processing, 2020.
- [23] C. Valentini-Botinhao, X. Wang, S. Takaki, and J. Yamagishi, “Speech enhancement for a noise-robust text-to-speech synthesis system using deep recurrent neural networks,” Proc. of Interspeech, pp. 352-356, 2016.
- [24] R. Scheibler, E. Bezzam, and I. Dokmanić, “Pyroomacoustics: A Python package for audio room simulations and array processing algorithms,” IEEE Int. Conf. on Acoustics, Speech, and Signal Processing, 2018.
This article is published under a Creative Commons Attribution-NoDerivatives 4.0 Internationa License.