Paper:
Selection of Required Controller for Position- and Force-Based Task in Motion Copying System
Toshiaki Okano*, Roberto Oboe**, Kouhei Ohnishi***, and Toshiyuki Murakami*
*Keio University
3-14-1 Hiyoshi, Kouhoku, Yokohama, Kanagawa 223-8522, Japan
**Department of Management Engineering, University of Padova
Stradella S. Nicola, 3, Vicenza 36100, Italy
***Haptics Research Center, Keio University
7-1 Shinkawasaki, Saiwai, Kawasaki, Kanagawa 212-0032, Japan
With the remarkable development of related technologies, the number of robots has been gradually increasing and their presence is becoming much more familiar in our daily lives. The motion copying system (MCS) is utilized as the method for conducting some tasks by robots. This system enables tasks to be reproduced when the environmental conditions are not changed. The task reproduction performance is degraded when environmental variations occur, and human-like adaptable motion is expected to be developed in the MCS. This study reveals the dominant element of motion, and the control strategy is varied at each time in each axis by considering the task realization. The flexibility of motion is learned from both the operator and the task implementation. The task reproduction experiments by MCS are conducted to verify the effectiveness of the proposal.
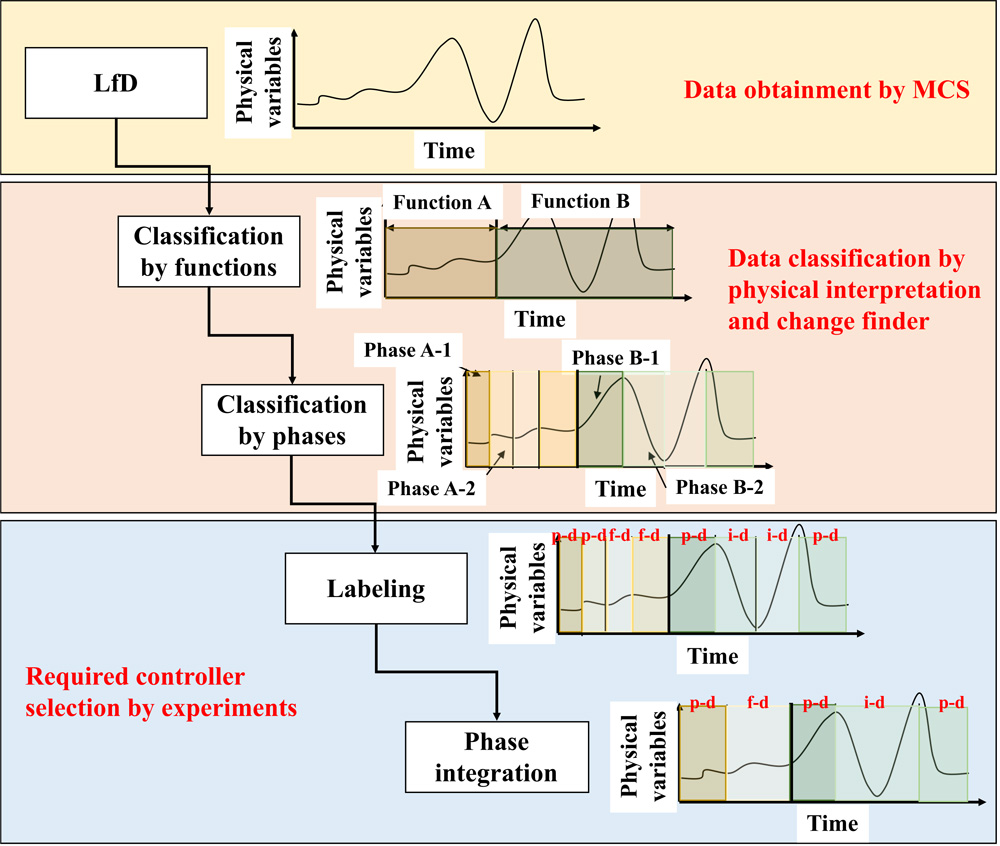
The illustration of task and motion analysis processes for the MCS
- [1] P. Kormushev, S. Calinon, and D. G. Caldwell, “Imitation learning of positional and force skills demonstrated via kinesthetic teaching and haptic input,” Advanced Robotics, Vol.25, No.5, pp. 581-603, 2011.
- [2] K. Kronander and A. Billard, “Learning compliant manipulation through kinesthetic and tactile human-robot interaction,” IEEE Trans. on Haptics, Vol.7, No.3, pp. 367-380, 2014.
- [3] J. D. F. Heredia, J. I. U. Rubrico, S. Shirafuji, and J. Ota, “Teaching tasks to multiple small robots by classifying and splitting a human example,” J. Robot. Mechatron., Vol.29, No.2, pp. 419-433, 2017.
- [4] Z. Zhu and H. Hu, “Robot Learning from Demonstration in Robotic Assembly: A Survey,” Robotics, Vol.7, No.2, 17, 2018.
- [5] Y. Yokokura, S. Katsura, and K. Ohishi, “Stability analysis and experimental validation of a motion-copying system,” IEEE Trans. on Industrial Electronics, Vol.56, No.10, pp. 3906-3913, 2009.
- [6] W. Iida and K. Ohnishi, “Reproducibility and operationality in bilateral teleoperation,” Proc. of the 8th IEEE Int. Workshop on Advanced Motion Control, pp. 217-222, 2004.
- [7] K. Miura, A. Matsui, and S. Katsura, “Synthesis of motion-reproduction systems based on motion-copying system considering control stiffness,” IEEJ J. of Industry Applications, Vol.21, No.2, pp. 1015-1023, 2016.
- [8] Y. Nagatsu and S. Katsura, “Decoupling and Performance Enhancement of Hybrid Control for Motion-Copying System,” IEEE Trans. on Industrial Electronics, Vol.64, No.1, pp. 420-431, 2017.
- [9] Y. Hirai, T. Mizukami, Y. Suzuki, T. Tsuji, and T. Watanabe, “Hierarchical Proximity Sensor for High-Speed and Intelligent Control of Robotic Hand,” J. Robot. Mechatron., Vol.31, No.3, pp. 453-463, 2019.
- [10] T. Morizono, K. Tahara, and H. Kino, “Choice of Muscular Forces for Motion Control of a Robot Arm with Biarticular Muscles,” J. Robot. Mechatron., Vol.31, No.1, pp. 143-155, 2019.
- [11] D. M. Wolpert, R. C. Miall, and M. Kawato, “Internal models in the cerebellum,” Trends in cognitive sciences, Vol.2, No.9, pp. 338-347, 1998.
- [12] L. Rozo, S. Calinon, D. G. Caldwell, P. Jimenez, and C. Torras, “Learning physical collaborative robot behaviors from human demonstrations,” IEEE Trans. on Robotics, Vol.32, No.3, pp. 513-527, 2016.
- [13] N. Saito and T. Satoh, “Posture control considering joint stiffness of a robotic arm driven by rubberless artificial muscle,” Int. J. Automation Technol., Vol.10, No.4, pp. 503-510, 2016.
- [14] F. J Abu-dakka, L. Rozo, amd D. G. Caldwell, “Force-based Learning of Variable Impedance Skills for Robotic Manipulation,” Robotics and Autonomous Systems, Vol.109, pp. 156-167, 2018.
- [15] C. Li, Z. Zhang, G. Xia, X. Xie, and Q. Zhu, “Efficient Force Control Learning System for Industrial Robots Based on Variable Impedance Control,” Sensors, Vol.18, No.8, 2539, 2018.
- [16] R. Wu, H. Zhang, T. Peng, L. Fu, and J. Zhao, “Human-Robot Interaction and Demonstration Learning Mode Based on Electromyogram Signal and Variable Impedance Control,” Mathematical Problems in Engineering, Vol.2018, 8658791, 2018.
- [17] K. Hongo, Y. Nakanishi, M. Yoshida, I. Mizuuchi, and M. Inaba, “Development of bilateral wearable device kento for control robots using muscle-actuator modules,” J. Robot. Mechatron., Vol.22, No.3, pp. 308-314, 2010.
- [18] M. T. Mason, “Compliance and force control for computer controlled manipulators,” IEEE Trans. on Systems, Man, and Cybernetics, Vol.11, No.6, pp. 418-432, 1981.
- [19] M. H. Raibert and J. J. Craig, “Hybrid position/force control of manipulators,” J. of Dynamic Systems, Measurement, and Control, Vol.103, No.2, pp. 126-133, 1981.
- [20] N. Hogan, “Impedance control: An approach to manipulation,” 1984 American Control Conf., pp. 304-313, 1984.
- [21] O. Khatib, “A unified approach for motion and force control of robot manipulators: The operational space formulation,” IEEE J. on Robotics and Automation, Vol.3, No.1, pp. 43-53, 1987.
- [22] B. Hannaford, “A design framework for teleoperators with kinesthetic feedback,” IEEE Trans. on Robotics and Automation, Vol.5, No.4, pp. 426-434, 1989.
- [23] D. A. Lawrence, “Stability and transparency in bilateral teleoperation,” IEEE Trans. on Robotics and Automation, Vol.9, No.5, pp. 624-637, 1993.
- [24] P. F. Hokayem and M. W. Spong, “Bilateral teleoperation: An historical survey,” Automatica, Vol.42, No.12, pp. 2035-2057, 2006.
- [25] K. Ohnishi, M. Shibata, and T. Murakami, “Motion control for advanced mechatronics,” IEEE/ASME Trans. on Mechatronics, Vol.1, No.1, pp. 56-67, 1996.
- [26] T. Murakami, F. Yu, and K. Ohnishi, “Torque sensorless control in multidegree-of-freedom manipulator,” IEEE Trans. on Industrial Electronics, Vol.40, No.2, pp. 259-265, 1993.
- [27] Y. Kawahara and M. Sugiyama, “Sequential change-point detection based on direct density-ratio estimation,” Statistical Analysis and Data Mining: The ASA Data Science J., Vol.5, No.2, pp. 114-127, 2012.
- [28] S. Liu, M. Yamada, N. Collier, and M. Sugiyama, “Change-point detection in time-series data by relative density-ratio estimation,” Neural Networks, Vol.43, pp. 72-83, 2013.
- [29] S. J. Lederman and R. L. Klatzky, “Haptic perception: A tutorial,” Attention, Perception, & Psychophysics, Vol.71, No.7, pp. 1439-1459, 2009.
This article is published under a Creative Commons Attribution-NoDerivatives 4.0 Internationa License.