Paper:
Enhancement of Scan Matching Using an Environmental Magnetic Field
Sam Ann Rahok*, Hirohisa Oneda**, Taichi Nakayama*, Kazumichi Inoue*, Shigeji Osawa**, Akio Tanaka*, and Koichi Ozaki***
*National Institute of Technology, Oyama College
771 Nakakuki, Oyama, Tochigi 323-0806, Japan
**National Institute of Technology, Yuge College
1000 Yuge, Kamijima, Ehime 794-2593, Japan
***Utsunomiya University
712 Yoto, Utsunomiya, Tochigi 321-8585, Japan
Scan matching is one of the most reliable localization methods for mobile robots in known environments. However, an unexpected shift in posture remains its major issue. A method that uses an environmental magnetic field, a magnetic field that occurs in the environment, is presented to address this issue. The environmental magnetic field, which mostly refers to the geomagnetic field, is rarely changed by time. This unique property provides a means to enhance scan matching to provide a more robust localization method by using it to compensate the mobile robot’s pose. In this study, we describe how to compensate the mobile robot’s pose with the environmental magnetic field. Through experiments, we show that a mobile robot with the proposed method can recover, even if irregular changes in posture occur during the navigation.
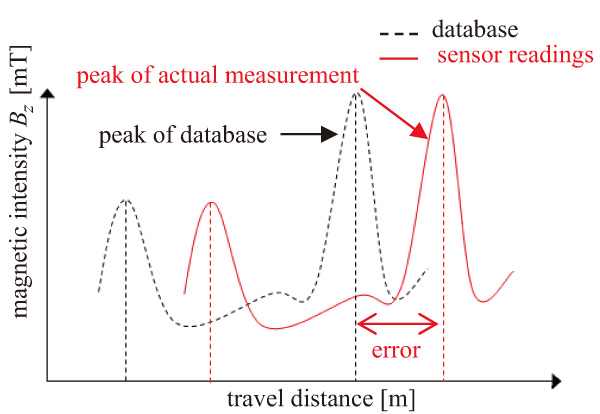
Localization using Bz of magnetic field
- [1] F. Gustafsson, F. Gunnarsson, N. Bergman, U. Forssell, J. Jansson, R. Karlsson, and P.-J. Nordlund, “Particle Filter for Positioning, Navigation, and Tracking,” IEEE Trans. Signal Process, Vol.50, No.2, pp. 425-437, 2002.
- [2] S. Thrun, “Particle Filters in Robotics,” UAI’02 Proc. of the 18th Conf. on Uncertainty in Artificial Intelligence, pp. 511-518, 2002.
- [3] M. D. Breitenstein, F. Reichlin, B. Leibe, E. Koller-Meier, and L. Van Gool, “Robust Tracking-by-detection using a detector confidence particle filter,” Proc. of IEEE Int. Conf. on Computer Vision, pp. 1515-1522, 2009.
- [4] S. Thrun, D. Fox, W. Burgard, and F. Dellaert, “Robust Monte Carlo Localization for Mobile Robots,” Artificial Intelligence J., Vol.128, pp. 99-141, 2011.
- [5] S. Kristensen and P. Jensfelt, “An Experimental Comparison of Localisation Methods, the MHL Sessions,” Proc. of IEEE/RSJ Int. Conf. on Intelligent Robots and Systems, pp. 992-997, 2003.
- [6] S. Engelson and D. McDermott, “Error Correction in Mobile Robot Map Learning,” Proc. of IEEE Int. Conf. on Robotics and Automation, pp. 2555-2560, 1992.
- [7] S. Toshifumi, “An Investigation on a Magnetic Field Environment Measuring Technics (part 3),” Summaries of Technical Papers of Annual Meeting Architechural of Japan, pp. 82-182, 2000 (in Japanese).
- [8] S. A. Rahok, Y. Shikanai, and K. Ozaki, “Navigation Using an Environmental Magnetic Field for Outdoor Autonomous Mobile Robots,” Advanced Robotics, Vol.25, Nos.13-14, pp. 1751-1771, 2011.
- [9] S. A. Rahok, K. Inoue, and K. Ozaki, “Development of a Mobile Robot to Run in Tsukuba Challenge 2010,” Advanced Robitics, Vol.26, No.14, pp. 1555-1575, 2012.
- [10] S. A. Rahok, H. Oneda, A. Tanaka, and K. Ozaki, “A Robust Navigation Method For Mobile Robots in Real-world Environment,” J. Robot. Mechatron., Vol.26, No.2, pp. 177-184, 2014.
- [11] S. Thrun, W. Bergard, and D. Fox, “Probabilistic Robotics (Intelligent Robotics and Autonomous Agents series),” MIT Press, 2006.
This article is published under a Creative Commons Attribution-NoDerivatives 4.0 Internationa License.