Research Paper:
3D Ship Hull Design Direct Optimization Using Generative Adversarial Network
Luan Thanh Trinh*
, Tomoki Hamagami*, and Naoya Okamoto**
*Yokohama National University
79-1 Tokiwadai, Hodogaya-ku, Yokohama, Kanagawa 240-0067, Japan
**Japan Marine United Corporation
Yokohama Blue Avenue Building, 4-4-2 Minatomirai, Nishi-ku, Yokohama, Kanagawa 220-0012, Japan
The direct optimization of ship hull designs using deep learning algorithms is increasingly expected, as it proposes optimization directions for designers almost instantaneously, without relying on complex, time-consuming, and expensive hydrodynamic simulations. In this study, we proposed a GAN-based 3D ship hull design optimization method. We eliminated the dependence on hydrodynamic simulations by training a separate model to predict ship performance indicators. Instead of a standard discriminator, we applied a relativistic average discriminator to obtain better feedback regarding the anomalous designs. We add two new loss functions for the generator: one restricts design variability, and the other sets improvement targets using feedback from the performance estimation model. In addition, we propose a new training strategy to improve learning effectiveness and avoid instability during training. The experimental results show that our model can optimize the form factor by 5.251% while limiting the deterioration of other indicators and the variability of the ship hull design.
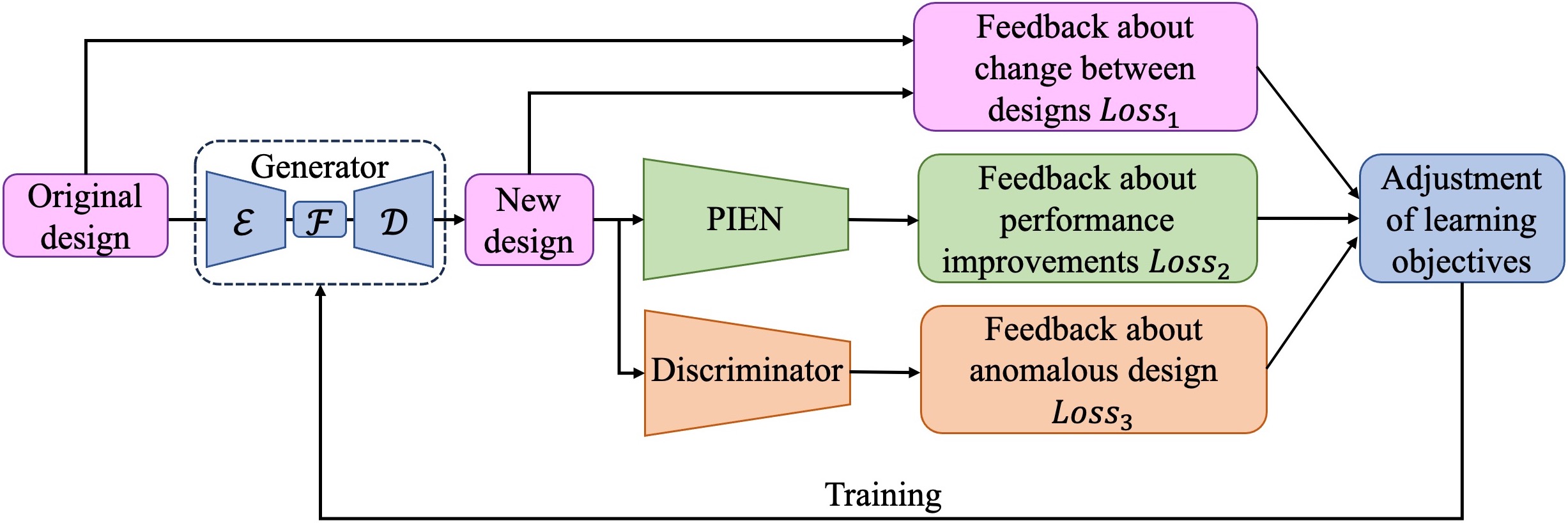
Overview of the proposed method
- [1] G. Guan, Q. Yang, Y. Wang, S. Zhou, and Z. Zhuang, “Parametric design and optimization of SWATH for reduced resistance based on evolutionary algorithm,” J. of Marine Science and Technology, Vol.26, pp. 54-70, 2021. https://doi.org/10.1007/s00773-020-00721-w
- [2] H.-J. Kim, J.-E. Choi, and H.-H. Chun, “Hull-form optimization using parametric modification functions and particle swarm optimization” J. of Marine Science and Technology, Vol.21, pp. 129-144, 2016. https://doi.org/10.1007/s00773-015-0337-y
- [3] X. Gua, W. Li, and F. Iorio, “Convolutional neural networks for steady flow approximation,” Proc. of the 22nd ACM SIGKDD Int. Conf. on Knowledge Discovery and Data Mining, pp. 481-490, 2020. https://doi.org/10.1145/2939672.2939738
- [4] O. Hennigh, “Lat-Net: Compressing lattice Boltzmann flow simulations using deep neural networks,” arXiv:1705.09036, 2017. https://doi.org/10.48550/arXiv.1705.09036
- [5] N. Umetani and B. Bickel, “Learning three-dimensional flow for interactive aerodynamic design,” ACM Trans. on Graphics, Vol.37, No.4, Article No.89, 2017. https://doi.org/10.1145/3197517.3201325
- [6] A. H. Nobari, W. Chen, and F. Ahmed, “Range-GAN: Range-constrained generative adversarial network for conditioned design synthesis,” Int. Design Engineering Technical Conf. and Computers and Information in Engineering Conf., 2021.
- [7] W. Chen and F. Ahmed, “Mo-PaDGAN: Reparameterizing engineering designs for augmented multi-objective optimization,” Applied Soft Computing, Vol.113, Part A, Article No.107909, 2021. https://doi.org/10.1016/j.asoc.2021.107909
- [8] J. Li and M. Zhang, “On deep-learning-based geometric filtering in aerodynamic shape optimization,” Aerospace Science and Technology, Vol.112, Article No.106603, 2021. https://doi.org/10.1016/j.ast.2021.106603
- [9] I. Goodfellow, J. Pouget-Abadie, M. Mirza, B. Xu, D. Warde-Farley, S. Ozair, A. Courville, and Y. Bengio, “Generative adversarial nets,” Advances in Neural Information Processing Systems, 2014.
- [10] A. Jolicoeur-Martineau, “The relativistic discriminator: A key element missing from standard GAN,” arXiv:1807.00734, 2018. https://doi.org/10.48550/arXiv.1807.00734
- [11] L. Bonfiglio, P. Perdikaris, and S. Brizzolara, “Multi-fidelity Bayesian optimization of SWATH hull forms,” J. of Ship Research, Vol.64, No.2, pp. 154-170, 2023. https://doi.org/10.5957/jsr.2020.64.2.154
- [12] H. Bagheri, H. Ghassemi, and A. Dehghanian, “Optimizing the seakeeping performance of ship hull forms using genetic algorithm,” The Int. J. on Marine Navigation and Safety of Sea Transportation, Vol.8, No.1, pp. 49-57, 2014. http://doi.org/10.12716/1001.08.01.06
- [13] S. Mahmood and D. Huang, “Computational fluid dynamics based bulbous bow optimization using a genetic algorithm,” J. of Marine Science and Engineering, Vol.11, pp. 286-294, 2012. https://doi.org/10.1007/s11804-012-1134-1
- [14] S. Zhang, B. Zhang, T. Tezdogan, L. Xu, and Y. Lai, “Computational fluid dynamics-based hull form optimization using approximation method,” Engineering Applications of Computational Fluid Mechanics, Vol.12, pp. 74-88, 2018. https://doi.org/10.1080/19942060.2017.1343751
- [15] A. Serani, C. Leotardi, U. Iemma, E. F. Campana, G. Fasano, and M. Diez, “Parameter selection in synchronous and asynchronous deterministic particle swarm optimization for ship hydrodynamics problems,” Applied Soft Computing, Vol.49, pp. 313-334, 2016. https://doi.org/10.1016/j.asoc.2016.08.028
- [16] Q. Zheng, B.-W. Feng, Z.-Y. Liu, and H.-C. Chang, “Application of improved particle swarm optimisation algorithm in hull form optimisation,” J. of Marine Science and Engineering, Vol.9, No.9, Article No.955, 2021. https://doi.org/10.3390/jmse9090955
- [17] A. Abbas, A. Rafiee, and M. Haase, “DeepMorpher: Deep learning-based design space dimensionality reduction for shape optimisation,” J. of Engineering Design, Vol.34, No.3, pp. 254-270, 2023. https://doi.org/10.1080/09544828.2023.2192606
- [18] W. Chen, K. Chiu, and M. Fuge, “Aerodynamic design optimization and shape exploration using generative adversarial networks,” AIAA Scitech 2019 Forum, 2020. https://doi.org/10.2514/6.2019-2351
- [19] S. Khan, K. Goucher-Lambert, K. Kostas, and P. Kaklis, “ShipHullGAN: A generic parametric modeller for ship hull design using deep convolutional generative model,” Computer Methods in Applied Mechanics and Engineering, Vol.411, Article No.116051, 2022. https://doi.org/10.1016/j.cma.2023.116051
- [20] G. Gkioxari, J. Malik, and J. Johnson, “Mesh RCNN,” Proc. of the IEEE/CVF Int. Conf. on Computer Vision, pp. 9784-9794, 2021. https://doi.org/10.1109/ICCV.2019.00988
- [21] A.G. Özbay, A. Hamzehloo, S. Laizet, P. Tzirakis, G. Rizos, and B. Schuller, “Poisson CNN: Convolutional neural networks for the solution of the Poisson equation on a Cartesian mesh,” Data-Centric Engineering, Vol.2, Article No.e6, 2021. https://doi.org/10.1017/dce.2021.7
- [22] R. Hanocka, A. Hertz, N. Fish, R. Giryes, S. Fleishman, and D. Cohen-Or, “MeshCNN: A network with an edge,” ACM Trans. on Graphics, Vol.38, No.4, Article No.90, 2019. https://doi.org/10.1145/3306346.3322959
- [23] R. Hanocka, G. Metzer, R. Giryes, and D. Cohen-Or, “Point2Mesh: A self-prior for deformable meshes,” arXiv:2005.11084, 2020. https://doi.org/10.48550/arXiv.2005.11084
- [24] N. Wang, Y. Zhang, Z. Li, Y. Fu, W. Liu, and Y.-G. Jiang, “Pixel2Mesh: Generating 3D mesh models from single RGB images,” Proc. of the IEEE/CVF Int. Conf. on Computer Vision, pp. 52-67 2018.
- [25] A. Dosovitskiy, L. Beyer, A. Kolesnikov, D. Weissenborn, X. Zhai, T. Unterthiner, M. Dehghani, M. Minderer, G. Heigold, S. Gelly, J. Uszkoreit, and N. Houlsby, “An image is worth 16x16 words: Transformers for image recognition at scale,” Proc. of the IEEE Conf. on Computer Vision and Pattern Recognition, pp. 1097-1105, 2021.
- [26] Z. Liu, Y. Lin, Y. Cao, H. Hu, Y. Wei, Z. Zhang, S. Lin, and B. Guo, “Swin transformer: Hierarchical vision transformer using shifted windows,” Proc. of the IEEE/CVF Int. Conf. on Computer Vision, pp. 10012-10022, 2021.
- [27] H. Touvron, M. Cord, M. Douze, F. Massa, A. Sablayrolles, and H. Jegou, “Training data-efficient image transformers & distillation through attention,” Proc. of the 38th Int. Conf. on Machine Learning, pp. 10347-10357, 2021.
- [28] W. Wang, E. Xie, X. Li, D.-P. Fan, K. Song, D. Liang, T. Lu, P. Luo, and L. Shao, “Pyramid vision transformer: A versatile backbone for dense prediction without convolutions,” arXiv:2102.12122, 2021. https://doi.org/10.48550/arXiv.2102.12122
- [29] M. Raghu, T. Unterthiner, S. Kornblith, C. Zhang, and A. Dosovitskiy, “Do vision transformers see like convolutional neural networks?,” arXiv:2108.08810, 2021. https://doi.org/10.48550/arXiv.2108.08810
- [30] A. Vaswani, N. Shazeer, N. Parmar, J. Uszkoreit, L. Jones, A. N. Gomez, Ł. Kaiser, and I. Polosukhin, “Attention is all you need,” Advances in Neural Information Processing Systems, pp. 5998-6008, 2017.
- [31] K. He, X. Zhang, S. Ren, and J. Sun, “Deep residual learning for image recognition,” Proc. of the IEEE Conf. on Computer Vision and Pattern Recognition, pp. 770-778, 2016.
- [32] A. Krizhevsky, I. Sutskever, and G. E. Hinton, “ImageNet classification with deep convolutional neural networks,” Proc. of the IEEE Conf. on Computer Vision and Pattern Recognition, pp. 1097-1105, 2012.
- [33] X. Ding, X. Zhang, Y. Zhou, J. Han, G. Ding, and J. Sun, “Scaling up your kernels to 31x31: Revisiting large kernel design in CNNs,” arXiv:2203.06717, 2022. https://doi.org/10.48550/arXiv.2203.06717
- [34] C. B. Vennerød, A. Kjærran, and E. S. Bugge, “Long Short-Term Memory RNN,” arXiv:2105.06756, 2021. https://doi.org/10.48550/arXiv.2105.06756
- [35] C. Ledig, L. Theis, F. Huszar, J. Caballero, A. Cunningham, A. Acosta, A. Aitken, A. Tejani, J. Totz, Z. Wang, and W. Shi, “Photo-realistic single image super-resolution using a generative adversarial network,” Computer Vision and Pattern Recognition, pp. 4681-4690, 2017.
- [36] A. Subramaniam, M. L. Wong, R. D. Borker, S. Nimmagadda, and S. K. Lele, “Turbulence enrichment using physics-informed generative adversarial networks,” arXiv:2003.01907, 2020. https://doi.org/10.48550/arXiv.2003.01907
- [37] S. Ioffe and C. Szegedy, “Batch normalization: Accelerating deep network training by reducing internal covariate shift,” arXiv:1502.03167, 2015. https://doi.org/10.48550/arXiv.1502.03167
- [38] X. Wang, K. Yu, S. Wu, J. Gu, Y. Liu, C. Dong, C. C. Loy, Y. Qiao, and X. Tang, “ESRGAN: Enhanced super-resolution generative adversarial networks,” Computer Vision - ECCV 2018 Workshops, 2018.
- [39] B. Xu, N. Wang, T. Chen, and M. Li, “Empirical evaluation of rectified activations in convolutional network,” Computer Vision and Pattern Recognition, 2015.
This article is published under a Creative Commons Attribution-NoDerivatives 4.0 Internationa License.