Research Paper:
Construction of Handwritten Indus Signs Dataset Employing Social Approach
Sujata Saini
, Hiroki Shibata
, and Yasufumi Takama

School of System Design, Tokyo Metropolitan University
6-6 Ashigaoka, Hino, Tokyo 191-0065, Japan
This paper constructs a dataset of handwritten Indus signs employing a social approach. A writing system called the Indus script was created in the Indus civilization. It has been decoded numerous times throughout the years, but it has not yet been fully deciphered. Due to a lack of information and the scarcity of evidence, the mystery of the Indus signs has not yet been fully solved. Recently, there has been an increase in demand for huge datasets in order to use cutting-edge machine learning techniques. Considering the restricted availability of images of authentic Indus signs, this paper proposes creating an Indus signs dataset by asking participants to draw the Indus signs while referring to the image of the original Indus signs. A web application was developed and used to collect the 44 participants’ handwritten images of ten Indus signs. To show the availability of the constructed dataset, it is used to train convolutional neural networks. The experimental result demonstrates that the model can classify the images of original Indus script with 70% accuracy.
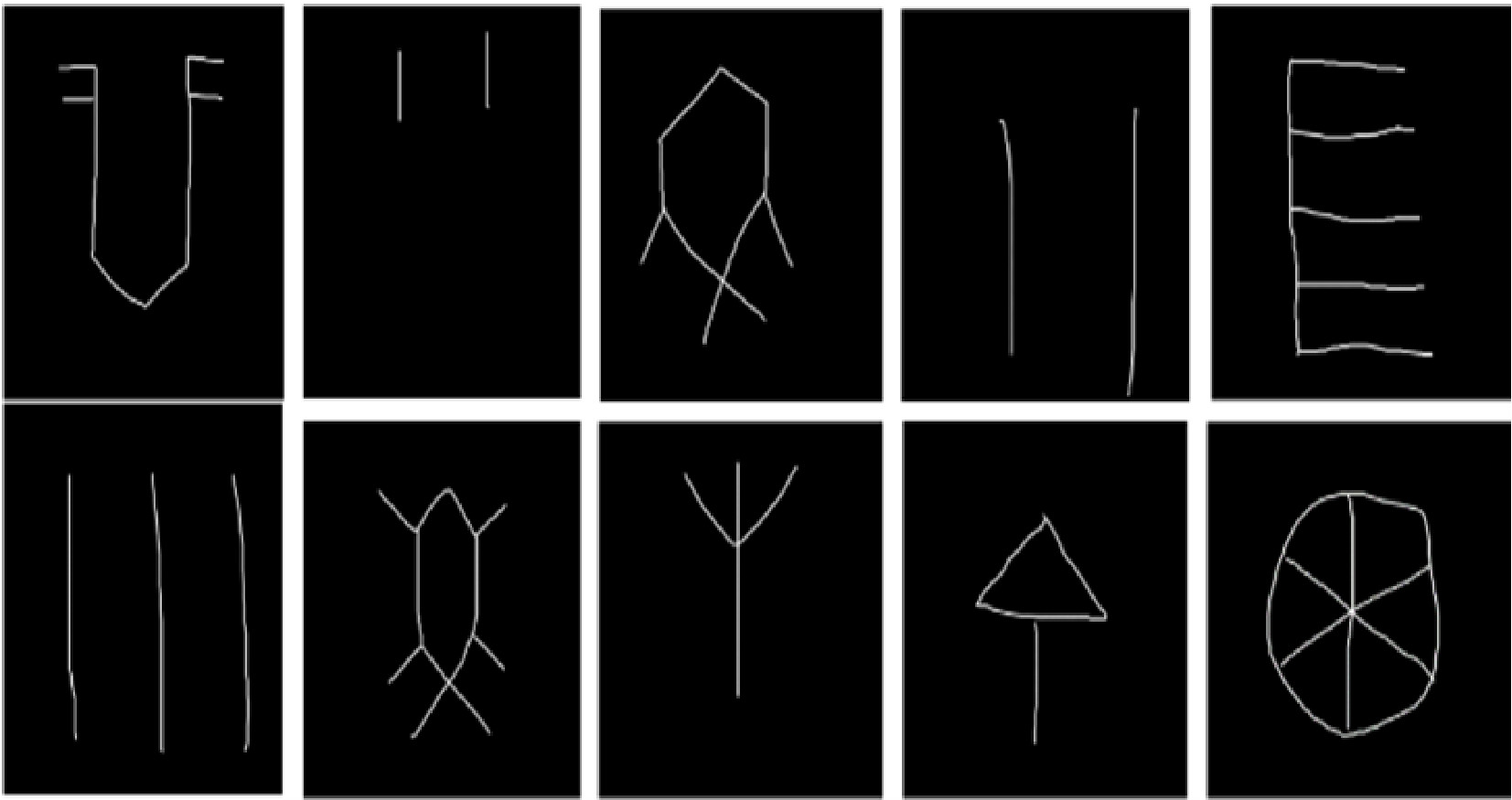
Handwritten Indus sign dataset
- [1] A. Fuls, “Ancient Writing and Modern Technologies – Structural Analysis of Numerical Indus Inscriptions,” Studies on Indus Script: Conf. on Indus Script Mohenjodaro 2020, pp. 57-90, 2020.
- [2] R. P. N. Rao et al., “A Markov Model of the Indus Script,” Proc. Natl. Acad. Sci., Vol.106, No.33, pp. 13685-13690, 2009. https://doi.org/10.1073/pnas.0906237106
- [3] R. P. N. Rao et al., “Entropy, the Indus Script, and Language: A reply to R. Sproat,” Comput. Linguist., Vol.36, No.4, pp. 795-805, 2010. https://doi.org/10.1162/coli_c_00030
- [4] N. Yadav et al., “Statistical Analysis of the Indus Script Using n-Grams,” PLOS ONE, Vol.5, No.3, Article No.e9506, 2010. https://doi.org/10.1371/journal.pone.0009506
- [5] B. K. Wells and A. Fuls, “Interactive Concordance of Indus Texts (ICIT): An Online Database of Indus Inscriptions and Iconography.” https://www.indus.epigraphica.de/ [Accessed March 29, 2022]
- [6] F. Gao, “Rapid Feature Retrieval Method in Large-Scale Image Database,” J. Adv. Comput. Intell. Intell. Inform., Vol.22, No.7, pp. 1088-1092, 2018. https://doi.org/10.20965/jaciii.2018.p1088
- [7] S. Saini, H. Shibata, and Y. Takama, “Toward Construction of Handwritten Indus Signs Dataset,” The 10th Int. Symp. on Computational Intelligence and Industrial Applications (ISCIIA2022), Session No.C2-2, 2022.
- [8] V. Vani and S. R. Ananthalakshmi, “Soft Computing Approaches for Character Credential and Word Prophecy Analysis with Stone Encryptions,” Soft Comput., Vol.24, No.16, pp. 12013-12026, 2020. https://doi.org/10.1007/s00500-019-04643-7
- [9] S. Palaniappan and R. Adhikari, “Deep Learning the Indus Script,” arXiv: 1702.00523, 2017. https://doi.org/10.48550/arXiv.1702.00523
- [10] V. U. Prabhu, “Kannada-MNIST: A New Handwritten Digits Dataset for the Kannada Language,” arXiv: 1908.01242, 2019. https://doi.org/10.48550/arXiv.1908.01242
- [11] T. Clanuwat et al., “Deep Learning for Classical Japanese Literature,” arXiv: 1812.01718, 2018. https://doi.org/10.48550/arXiv.1812.01718
- [12] D. Gengler et al., “Personalized Federated Image Classification Using Weight Erosion,” École Polytechnique Fédérale de Lausanne, 2021. https://www.epfl.ch/labs/mlo/wp-content/uploads/2021/05/crpmlcourse-paper854.pdf [Accessed March 18, 2023]
- [13] A. Paszke et al., “PyTorch: An Imperative Style, High-Performance Deep Learning Library,” Proc. of the 33rd Int. Conf. on Neural Information Processing System (NIPS’19), pp. 8026-8037, 2019.
This article is published under a Creative Commons Attribution-NoDerivatives 4.0 Internationa License.