Paper:
Intelligent Edutab Box: Supporting Real-Time Face-to-Face Collaborative Learning
Yuto Omae*1, Kazutaka Mizukoshi*2, Tatsuro Furuya*3, Takayuki Oshima*4, Norihisa Sakakibara*4, Yoshiaki Mizuochi*4, Kazuhiro Yatsushiro*5, Masaya Matsushita*6, and Hirotaka Takahashi*7
*1Department of Industrial Engineering and Management, College of Industrial Technology, Nihon University
1-2-1 Izumi, Narashino, Chiba 275-8575, Japan
*2Digital Alliance Co., Ltd.
2-12-1 Kitaguchi, Kofu, Yamanashi 400-0024, Japan
*3Elementary School Attached to the University of Yamanashi
1-4-1 Kitashin, Kofu, Yamanashi 400-0005, Japan
*4Joetsu University of Education
1 Yamayashiki-machi, Joetsu, Niigata 943-8512, Japan
*5Yamanashi Prefectural University
5-11-1 Iida, Kofu, Yamanashi 400-0035, Japan
*6Nagaoka University of Technology
1603-1 Kamitomioka, Nagaoka, Niigata 940-2188, Japan
*7Research Center for Space Science, Advanced Research Laboratories, Tokyo City University
8-15-1 Todoroki, Setagaya, Tokyo 158-0082, Japan
Educational benefits of collaborative learning have been demonstrated in several studies and various systems have been developed to date. Numerous efforts have been made to enhance these benefits by supporting collaborative learning with information and communications technology. These efforts have primarily involved support for constructing collaborative learning groups, for collaborative learning in e-learning environments, and for collaborative learning analysis. This study aims to develop a computer-supported collaborative learning system that supports instructors in real time to facilitate collaborative learning in a face-to-face environment with multiple learners at the same time to provide enhanced support. Both the learner and instructor have one tablet terminal and conduct collaborative learning in a single classroom. Herein, the learner can use the tablet to save an educational log and freely browse the educational log of another learner. By referencing the educational logs, learners can learn through face-to-face communication. Additionally, the instructor can determine (1) who is viewing whose educational log and to what extent and (2) which learner is struggling to achieve targets. Herein, an overview of the proposed system is provided and the results obtained using the proposed system are reported to evaluate its effectiveness.
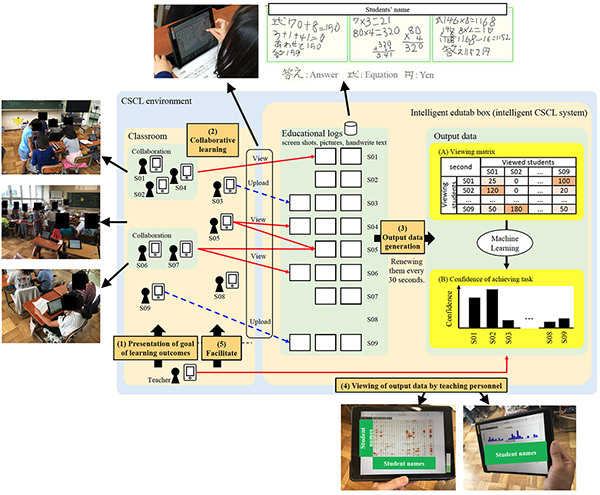
The Intelligent Edutab Box and CSCL environment
- [1] P. Dillenbourg, “What do you mean by collaborative learning?,” P. Dillenbourg (Ed.), “Collaborative learning: Cognitive and Computational Approaches,” pp. 1-19, Elsevier Science, 1999.
- [2] C. Y. Cheng, S. R. Liou, H. M. Tsai, and C. H. Chang, “The effects of team-based learning on learning behaviors in the maternal-child nursing course,” Nurse Educ. Today, Vol.34, No.1, pp. 25-30, 2014.
- [3] A. Aydin and F. Biyikli, “The effect of jigsaw technique on the students’ laboratory material recognition and usage skills in general physics laboratory-I course,” Universal J. of Educational Res., Vol.5, No.7, pp. 1073-1082, 2017.
- [4] M. Kazemi, “The effect of jigsaw technique on the learners’ reading achievement: the case of English as L2,” The Modern J. of Appl. Linguistics, Vol.5, No.3, pp. 170-184, 2012.
- [5] F. J. Prins, D. M. Sluijsmans, P. A. Kirschner, and J. W. Strijbos, “Formative peer assessment in a CSCL environment: a case study,” Assessment & Evaluation in Higher Educ., Vol.30, No.4, pp. 417-444, 2005.
- [6] P. Nikro and M. Barkhordari, “Investigation of the effect of collaborative learning on the self-confidence in solving mathematical problems,” Academic J. of Psychological Stud., Vol.4, No.3, pp. 122-128, 2015.
- [7] G. Zurita and M. Nussbaum, “A conceptual framework based on activity theory for mobile CSCL,” British J. of Educational Technol., Vol.38, No.2, pp. 211-235, 2007.
- [8] R. Valdivia and M. Nussbaum, “Face-to-face collaborative learning in computer science classes,” Int. J. of Eng. Educ., Vol.23, No.3, pp. 434-440, 2007.
- [9] K. Mizukoshi, T. Oshima, Y. Mizuochi, and K. Yatsushiro, “A portable CSCL system “edutab box” with an autonomous wireless network,” Proc. 2016 IEEE/SICE Int. Symp. on Syst. Integration, pp. 640-645, 2016.
- [10] E. Szewkis, M. Nussbaum, T. Rosen, J. Abalos, F. Denardin, D. Caballero, A. Tagle, and C. Alcoholado, “Collaboration within large groups in the classroom,” Int. J. of Computer-Supported Collaborative Learn., Vol.6, No.4, pp. 561-575, 2011.
- [11] L. K. Soh, N. Khandaker, and H. Jiang, “I-MINDS: A multiagent system for intelligent computer-supported collaborative learning and classroom management,” Int. J. of Artif. Intell. in Educ., Vol.18, No.2, pp. 119-151, 2008.
- [12] A. Casamayor, A. Amandi, and M. Campo, “Intelligent assistance for teachers in collaborative e-learning environments,” Comput. & Educ., Vol.53, No.4, pp. 1147-1154, 2009.
- [13] T. Monahan, G. McArdle, and M. Bertolotto, “Virtual reality for collaborative e-learning,” Comput. & Educ., Vol.50, No.4, pp. 1339-1353, 2008.
- [14] K. Andreas, T. Tsiatsos, T. Terzidou, and A. Pomportsis, “Fostering collaborative learning in Second Life: Metaphors and affordances,” Comput. & Educ., Vol.55, No.2, pp. 603-615, 2010.
- [15] N. Avouris, V. Komis, G. Fiotakis, M. Margaritis, and N. Tselios, “A tool to support interaction and collaboration analysis of learning activities,” CBLIS Conf. Proc. 2003 Volume I: New Technol. and Their Appl. in Educ., pp. 215-225, 2003.
- [16] N. Avouris, G. Fiotakis, G. Kahrimanis, M. Margaritis, and V. Komis, “Beyond logging of fingertip actions: analysis of collaborative learning using multiple sources of data,” J. of Interactive Learn. Res., Vol.18, No.2, pp. 231-250, 2007.
- [17] K. Mizukoshi, T. Oshima, N. Sakakibara, Y. Mizuochi, Y. Omae, H. Takahashi, and K. Yatsushiro, “Performance analysis of “edulog” system,” Proc. 2017 IEEE/SICE Int. Symp. on Syst. Integration, pp. 583-588, 2017.
- [18] J. Moreno, D. A. Ovalle, and R. M. Vicari, “A genetic algorithm approach for group formation in collaborative learning considering multiple student characteristics,” Comput.& Educ., Vol.58, No.1, pp. 560-569, 2012.
- [19] M. Wessner and H. R. Pfister, “Group formation in computer-supported collaborative learning,” Proc. 2001 Int. ACM SIGGROUP Conf. on Supporting Group Work, pp. 24-31, 2001.
- [20] Y. Omae, T. Furuya, K. Mizukoshi, T. Oshima, N. Sakakibara, Y. Mizuochi, K. Yatsushiro, and H. Takahashi, “Machine learning-based collaborative learning optimizer toward intelligent CSCL system,” Proc. 2017 IEEE/SICE Int. Symp. on Syst. Integration, pp. 577-582, 2017.
- [21] R. C. D. Reis, S. Isotani, C. L. Rodriguez, K. T. Lyra, P. A. Jaques, and I. I. Bittencourt, “Affective states in computer-supported collaborative learning: Studying the past to drive the future,” Comput. & Educ., Vol.120, pp. 29-50, 2018.
- [22] I. Magnisalis, S. Demetriadis, and A. Karakostas, “Adaptive and intelligent systems for collaborative learning support: A review of the field,” IEEE Trans. on Learn. Technol., Vol.4, No.1, pp. 5-20, 2011.
- [23] A. S. Palincsar and L. R. Herrenkohl, “Designing collaborative learning contexts,” Theory into Practice, Vol.41, No.1, pp. 26-32, 2002.
- [24] G. Tan, P. B. Gallo, G. M. Jacobs, and C. Lee, “Using cooperative learning to integrate thinking and information technology in a content-based writing lesson,” The Internet TESL J., Vol.5, No.8, pp. 13-21, 1999.
- [25] C. A. Collazos, L. A. Guerrero, J. A. Pino, S. Renzi, J. Klobas, M. Ortega, M. A. Redondo, and C. Bravo, “Evaluating collaborative learning processes using system-based measurement,” Educational Technol. & Soc., Vol.10, No.3, pp. 257-274, 2007.
- [26] Y. Omae, T. Furuya, K. Mizukoshi, T. Oshima, N. Sakakibara, Y. Mizuochi, K. Yatsushiro, and H. Takahashi, “Data mining for discovering effective time-series transition of learning strategies on mutual viewing-based learning,” J. Adv. Comput. Intell. Intell. Inform., Vol.22, No.7, pp. 1046-1055, 2018.
- [27] S. Chayanukro, “A generalized e-learning usage behaviour model by data mining technique,” J. of Inf. and Commun. Technol., Vol.13, pp. 37-53, 2014.
- [28] A. F. Gkontzis, S. Kotsiantis, R. Tsoni, and V. S. Verykios, “An effective LA approach to predict student achievement,” Proc. of the 22nd Pan-Hellenic Conf. on Inform., pp. 76-81, 2018.
- [29] N. Kondo and T. Hatanaka, “Estimation of students’ learning states using bayesian networks and log data of learning management system,” Int. J. of Institutional Res. and Manage., Vol.3, No.2, pp. 35-49, 2019.
- [30] M. Ueno, “Intelligent LMS with an agent that learns from log data,” E-Learn: World Conf. on E-Learning in Corporate, Government, Healthcare, and Higher Educ., pp. 3169-3176, 2005.
- [31] Y. Park, “Analysis of online behavior and prediction of learning performance in blended learning environments,” Educational Technol. Int., Vol.15, No.2, pp. 71-88, 2014.
- [32] H. F. Golino, C. M. A. Gomes, and D. Andrade, “Predicting academic achievement of high-school students using machine learning,” Psychology, Vol.5, No.18, pp. 2046-2057, 2014.
- [33] H. Goker, H. I. Bulbul, and E. Irmak, “The estimation of students’ academic success by data mining methods,” 12th Int. Conf. on Mach. Learn. and Appl., Vol.2, pp. 535-539, 2013.
- [34] T. Oshima, Y. Mizuochi, K. Yatushiro, and K. Mizukoshi, “Development of CSCL system with usability for lower elementary school grade students,” Proc. 2016 IEEE/SICE Int. Symp. on Syst. Integration, pp. 646-651, 2016.
- [35] T. Hastie, R. Tibshirani, and J. Friedman, “The elements of statistical learning: data mining, inference, and prediction,” Springer, 2009.
- [36] C. Schuldt, I. Laptev, and B. Caputo, “Recognizing human actions: a local SVM approach,” Proc. 17th Int. Conf. on Pattern Recognit., Vol.3, pp. 32-36, 2004.
- [37] F. Xu, X. Liu, and K. Fujimura, “Pedestrian detection and tracking with night vision,” IEEE Trans. on Intell. Transp. Syst., Vol.6, No.1, pp. 63-71, 2005.
- [38] S. Huang and N. Fang, “Predicting student academic performance in an engineering dynamics course: A comparison of four types of predictive mathematical models,” Comput. & Educ., Vol.61, pp. 133-145, 2013.
- [39] A. S. Ahamed, N. T. Mahmood, and R. M. Rahman, “An intelligent system to predict academic performance based on different factors during adolescence,” J. of Inform. and Telecommunication, Vol.1, No.2, pp. 155-175, 2017.
- [40] H. Lu and J. Yuan, “Student performance prediction model based on discriminative feature selection,” Int. J. of Emerging Technol. in Learn., Vol.13, No.10, pp. 55-68, 2018.
- [41] L. Breiman, “Random Forests,” Mach. Learn., Vol.45, No.1, pp. 5-32, 2001.
- [42] M. Robnik-Sikonja and I. Kononenko, “Theoretical and empirical analysis of ReliefF and RReliefF,” Mach. Learn., Vol.53, No.1-2, pp. 23-69, 2003.
This article is published under a Creative Commons Attribution-NoDerivatives 4.0 Internationa License.