Paper:
Visualization of Potential Technical Solutions by SOM and Co-Clustering and its Extension to Multi-View Situation
Yasushi Nishida and Katsuhiro Honda
Osaka Prefecture University
1-1 Gakuen-cho, Naka-ku, Sakai, Osaka 599-8531, Japan
In order to support inspiration of potential technical solutions, this paper considers visualization of solving means varied in patent documents through SOM. Non-structured patent document data can be quantified through two different scheme: word level co-occurrence probability vectors and correlation coefficients of the generated co-occurrence probability vectors. Comparing the two SOMs derived with the above schemes is useful for supporting innovation acceleration through extraction of important pairs of related factors in new technology development. In this paper, co-cluster structures are utilized for emphasizing field-related solutions by constructing multiple SOMs after co-clustering. Document × keyword co-occurrence analysis achieves extraction of co-clusters consisting of mutually related pairs in particular fields. Additionally, this paper also considers an extension to a multi-view situation, where each patent is characterized by additional patent classification system of F-term by Japan Patent Office. Through multi-view co-clustering among documents × keywords × F-terms, theme field-related knowledge is demonstrated to be extracted.
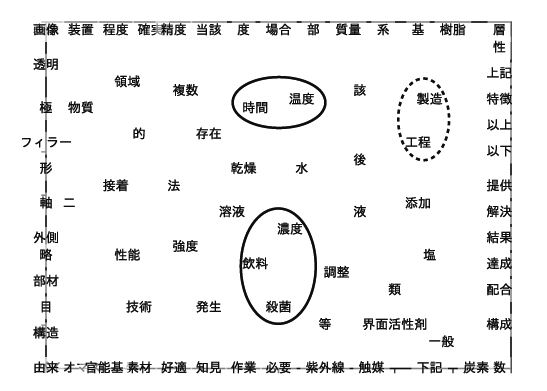
SOM visualization of technical term connections
- [1] T. Kohonen, “Self-Organizing Maps,” 3rd edition, Springer Series in Information Sciences, No.30, Springer, 2001.
- [2] Y. Nishida and K. Honda, “Visualization of technical solution by self-organizing maps for accelerating innovation,” J. of Japan Society for Fuzzy Theory and Intelligent Informatics, Vol.30, No.2, pp. 543-547, 2018 (in Japanese).
- [3] T. Hashimoto, K. Murakami, T. Inui, K. Utsumi, and M. Ishikawa, “Topic extraction and social problem detection based on document clustering,” Sociotechnica, Vol.5, pp. 216-226, 2008.
- [4] H. Yanaka and Y. Ohsawa, “Clustering documents on case vectors represented by predicate-argument structures – applied for eliciting technological problems from patents,” Proc. of 2016 Federated Conf. on Computer Science and Information Systems, Vol.ACSIS-8, pp. 175-180, 2016.
- [5] C.-H. Oh, K. Honda, and H. Ichihashi, “Fuzzy clustering for categorical multivariate data,” Proc. of Joint 9th IFSA World Congress and 20th NAFIPS Int. Conf., Vol.4, pp. 2154-2159, 2001.
- [6] K. Kummamuru, A. Dhawale, and R. Krishnapuram, “Fuzzy co-clustering of documents and keywords,” Proc. of the 12th IEEE Int. Conf. Fuzzy Systems, Vol.2, pp. 772-777, 2003.
- [7] Y. Nishida and K. Honda, “Visualization of potential technical solutions by self-organizing maps and co-cluster extraction,” Proc. of Joint 10th Int. Conf. on Soft Computing and Intelligent Systems and 19th Int. Symp. on Advanced Intelligent Systems (SCIS-ISIS 2018), pp. 820-825, 2018.
- [8] K. Honda, T. Sako, S. Ubukata, and A. Notsu, “Visual co-cluster assessment with intuitive cluster validation through cooccurrence-sensitive ordering,” J. Adv. Comput. Intell. Intell. Inform., Vol.22, No.5, pp. 585-592, 2018.
- [9] I. Schellner, “Japanese file index classification and F-terms,” World Patent Information, Vol.24, No.3, pp. 197-201, 2002.
- [10] S. Bickel and T. Scheffer, “Multi-view clustering,” Proc. of the 4th IEEE Int. Conf. on Data Mining, pp. 19-26, 2004.
- [11] Y.-M. Xu, C.-D. Wang, and J.-H. Lai, “Weighted multi-view clustering with feature selection,” Pattern Recognition, Vol.53, pp. 25-35, 2016.
- [12] Y. Yang and H. Wang, “Multi-view clustering: A survey,” Big Data Mining and Analytics, Vol.1, No.2, pp. 83-107, 2018.
- [13] T. Sato, “Neologism dictionary based on the language resources on the web for mecab-ipadic,” 2015, https://github.com/neologd/mecab-ipadic-neologd, [accessed April 16, 2019]
- [14] G. Salton and C. Buckley, “Term-weighting approaches in automatic text retrieval,” Information Processing & Management, Vol.24, No.5, pp. 513-523, 1988.
- [15] M. Abadi et al., “TensorFlow: Large-scale machine learning on heterogeneous systems,” https://www.tensorflow.org/ [accessed April 16, 2019]
- [16] D. Michler, N. Shahidzadeh, M. Westbroek, R. van Roij, and D. Bonn, “Are antagonistic salts surfactants?,” Langmuir, Vol.31, No.3, pp. 906-911, 2015.
- [17] T. Ramesh, B. Nayak, A. Amirbahman, C. P. Tripp, and S. Mukhopadhyay, “Application of ultraviolet light assisted titanium dioxide photocatalysis for food safety: A review,” Innovative Food Science & Emerging Technologies, Vol.38, Part A, pp. 105-115, 2016.
- [18] K. Honda, N. Yamamoto, S. Ubukata, and A. Notsu, “Noise rejection in MMMs-induced fuzzy co-clustering,” J. Adv. Comput. Intell. Intell. Inform., Vol.21, No.7, pp. 1144-1151, 2017.
This article is published under a Creative Commons Attribution-NoDerivatives 4.0 Internationa License.