Paper:
MMMs-Induced Possibilistic Fuzzy Co-Clustering and its Characteristics
Seiki Ubukata, Katsuya Koike, Akira Notsu, and Katsuhiro Honda
Osaka Prefecture University
1-1 Gakuen-cho, Nakaku, Sakai, Osaka 599-8531, Japan
In the field of cluster analysis, fuzzy theory including the concept of fuzzy sets has been actively utilized to realize flexible and robust clustering methods. Fuzzy C-means (FCM), which is the most representative fuzzy clustering method, has been extended to achieve more robust clustering. For example, noise FCM (NFCM) performs noise rejection by introducing a noise cluster that absorbs noise objects and possibilistic C-means (PCM) performs the independent extraction of possibilistic clusters by introducing cluster-wise noise clusters. Similarly, in the field of co-clustering, fuzzy co-clustering induced by multinomial mixture models (FCCMM) was proposed and extended to noise FCCMM (NFCCMM) in an analogous fashion to the NFCM. Ubukata et al. have proposed noise clustering-based possibilistic co-clustering induced by multinomial mixture models (NPCCMM) in an analogous fashion to the PCM. In this study, we develop an NPCCMM scheme considering variable cluster volumes and the fuzziness degree of item memberships to investigate the specific aspects of fuzzy nature rather than probabilistic nature in co-clustering tasks. We investigated the characteristics of the proposed NPCCMM by applying it to an artificial data set and conducted document clustering experiments using real-life data sets. As a result, we found that the proposed method can derive more flexible possibilistic partitions than the probabilistic model by adjusting the fuzziness degrees of object and item memberships. The document clustering experiments also indicated the effectiveness of tuning the fuzziness degree of object and item memberships, and the optimization of cluster volumes to improve classification performance.
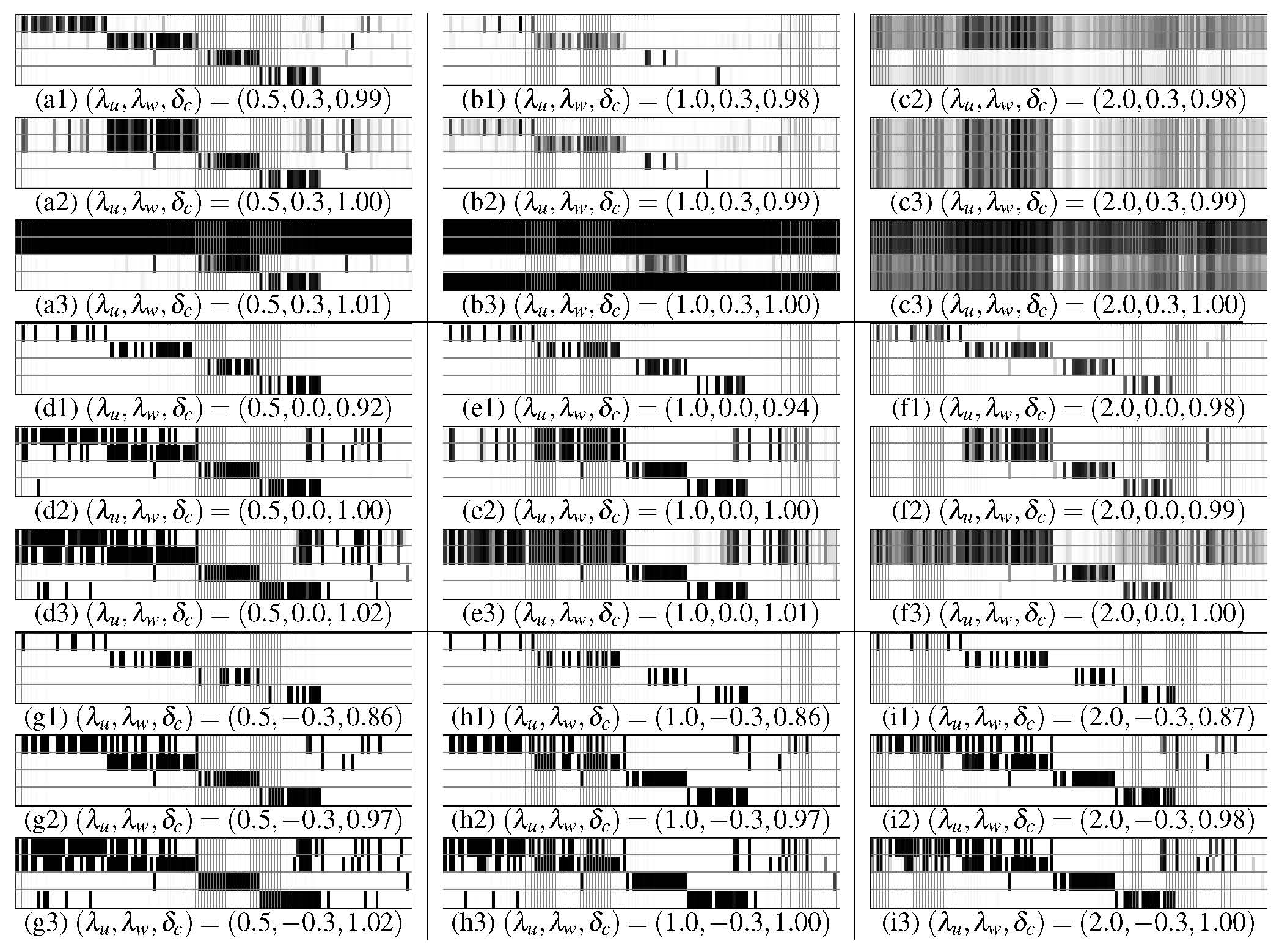
Possibilistic partitions of objects obtained by the proposed MMMs-induced possibilistic fuzzy co-clustering with different fuzziness degrees of objects and items"," and the parameter of noise rejection
- [1] L. Zadeh, “Fuzzy sets,” Information and Control, Vol.8, pp. 338-353, 1965.
- [2] J. B. MacQueen, “Some Methods of Classification and Analysis of Multivariate Observations,” Proc. 5th Berkeley Symp. Math. Stat. Prob., pp. 281-297, 1967.
- [3] J. C. Dunn, “A Fuzzy Relative of the ISODATA Process and Its Use in Detecting Compact Well-Separated Clusters,” J. of Cybernetics, Vol.3, pp. 32-57, 1974.
- [4] J. C. Bezdek, “Pattern Recognition with Fuzzy Objective Function Algorithms,” Plenum Press, 1981.
- [5] E. H. Ruspini, “A new approach to clustering,” Information and Control, Vol.15, No.1, pp. 22-32, 1969.
- [6] S. Miyamoto and M. Mukaidono, “Fuzzy c-means as a regularization and maximum entropy approach,” Proc. of the 7th Int. Fuzzy Syst. Assoc. World Cong., Vol.2, pp. 86-92, 1997.
- [7] S. Miyamoto, H. Ichihashi, and K. Honda, “Algorithms for Fuzzy Clustering,” Springer, 2008.
- [8] H. Ichihashi, K. Miyagishi, and K. Honda, “Fuzzy c-means clustering with regularization by K-L information,” Proc. of 10th IEEE Int. Conf. on Fuzzy Systems, Vol.2, pp. 924-927, 2001.
- [9] R. J. Hathaway, “Another interpretation of the EM algorithm for mixture distributions,” Statistics and Probability Letters, Vol.4, pp. 53-56, 1986.
- [10] R. O. Duda and P. E. Hart, “Pattern Classification and Scene Analysis,” John Wiley and Sons, 1973.
- [11] R. N. Davé, “Characterization and detection of noise in clustering,” Pattern Recognition Letters, Vol.12, No.11, pp. 657-664, 1991.
- [12] R. N. Davé and R. Krishnapuram, “Robust clustering methods: a unified view,” IEEE Trans. on Fuzzy Systems, Vol.5, pp. 270-293, 1997.
- [13] R. Krishnapuram and J. M. Keller, “A possibilistic approach to clustering,” IEEE Trans. on Fuzzy Systems, Vol.1, pp. 98-110, 1993.
- [14] R. Krishnapuram and J. M. Keller, “The possibilistic C-means algorithm: insights and recommendations,” IEEE Trans. on Fuzzy Systems, Vol.4, Issue 3, pp. 385-393, 1996.
- [15] N. R. Pal, K. Pal, and J. C. Bezdek, “A mixed C-means clustering model,” Proc. of the IEEE Int. Conf. on Fuzzy Systems, p. 1121, 1997.
- [16] Y. Kanzawa, “Sequential Cluster Extraction Using Power-Regularized Possibilistic c-Means,” J. Adv. Comput. Intell. Intell. Inform., Vol.19, No.1, pp. 67-73, 2015.
- [17] Y. Hamasuna and Y. Endo, “On Cluster Extraction from Relational Data Using L1-Regularized Possibilistic Assignment Prototype Algorithm,” J. Adv. Comput. Intell. Intell. Inform., Vol.19, No.1, pp. 23-28, 2015.
- [18] Y. Hamasuna and Y. Endo, “On Sequential Cluster Extraction Based on L1-Regularized Possibilistic c-Means,” J. Adv. Comput. Intell. Intell. Inform., Vol.19, No.5, pp. 655-661, 2015.
- [19] C.-H. Oh, K. Honda, and H. Ichihashi, “Fuzzy clustering for categorical multivariate data,” Proc. of Joint 9th IFSA World Congress and 20th NAFIPS Int. Conf., pp. 2154-2159, 2001.
- [20] Y. Kanzawa, “Fuzzy Co-Clustering Algorithms Based on Fuzzy Relational Clustering and TIBA Imputation,” J. Adv. Comput. Intell. Intell. Inform., Vol.18, No.2, pp. 182-189, 2014.
- [21] Y. Kanzawa, “Bezdek-Type Fuzzified Co-Clustering Algorithm,” J. Adv. Comput. Intell. Intell. Inform., Vol.19, No.6, pp. 852-860, 2015.
- [22] L. Rigouste, O. Cappé, and F. Yvon, “Inference and evaluation of the multinomial mixture model for text clustering,” Information Processing and Management, Vol.43, No.5, pp. 1260-1280, 2007.
- [23] I. Holmes, K. Harris, and C. Quince, “Dirichlet multinomial mixtures: generative models for microbial metagenomics,” PLoS ONE, Vol.7, Issue 2, e30126, 2012.
- [24] K. Honda, S. Oshio, and A. Notsu, “Fuzzy co-clustering induced by multinomial mixture models,” J. Adv. Comput. Intell. Intell. Inform., Vol.19, No.6, pp. 717-726, 2015.
- [25] K. Honda, N. Yamamoto, S. Ubukata, and A. Notsu, “Noise Rejection in MMMs-induced Fuzzy Co-clustering,” J. Adv. Comput. Intell. Intell. Inform., Vol.21, No.7, pp. 1144-1151, 2017.
- [26] S. Ubukata, K. Koike, A. Notsu, and K. Honda, “Possibilistic Co-clustering Based on Extension of Noise Rejection Scheme in FCCMM,” Proc. of Joint 17th World Congress of Int. Fuzzy Systems Association and 9th Int. Conf. on Soft Computing and Intelligent Systems, #93, pp. 1-6, 2017.
This article is published under a Creative Commons Attribution-NoDerivatives 4.0 Internationa License.