Paper:
Improving Grasping Performance of Suction Gripper with Curling Prevention Structure
Ryuichi Miura and Tsuyoshi Tasaki

Meijo University
1-501 Shiogamaguchi, Tenpaku-ku, Nagoya, Aichi 468-8502, Japan
To address labor shortages in retail stores, the automation of tasks, such as product display and disposal, using industrial robots is actively being promoted. These robots use suction grippers to grasp the products. However, conventional suction grippers often experience pad curling due to grasping pose errors, leading to air leaks and failed grasps. In this study, we proposed a curling prevention structure for suction pads to improve the grasping performance of suction grippers. Our solution focuses on a spring structure that reacts only to the parts where the force is applied. The proposed curling prevention structure consists of a ring that connects the cylinders with an elastic string. The cylinders passively applied force to the areas where curling occurred, thereby preventing curling. The design is highly versatile, as it is independent of the suction pad type. We performed experiments to evaluate the success or failure of grasping by varying the grasping angles of the robot arm for five types of products. The results showed that the range of grasping was expanded by up to 15° compared with that of conventional suction grippers. In addition, we performed experiments in which the robot grasped and conveyed products placed on store shelves using our gripper. The results showed improvements in both grasping and conveyance success rates.
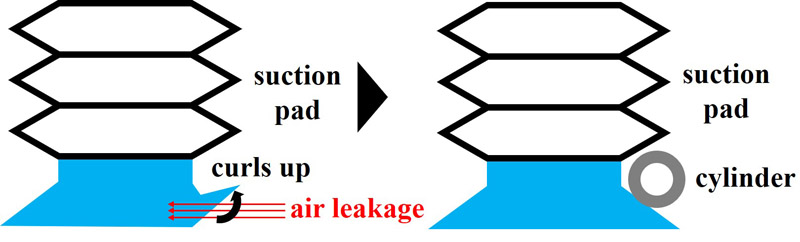
Adding cylinders prevents air leakage
- [1] G. A. Garcia Ricardez et al., “Restock and straightening system for retail automation using compliant and mobile manipulation,” Advanced Robotics, Vol.34, Nos.3-4, pp. 235-249, 2020. https://doi.org/10.1080/01691864.2019.1698460
- [2] J. Tanaka et al., “Portable compact suction pad unit for parallel grippers,” Advanced Robotics, Vol.34, Nos.3-4, pp. 202-218, 2020. https://doi.org/10.1080/01691864.2019.1686421
- [3] K. Matsumoto et al., “Selective instance segmentation for pose estimation,” Advanced Robotics, Vol.36, Nos.17-18, pp. 890-899, 2022. https://doi.org/10.1080/01691864.2022.2104621
- [4] J. Tanaka et al., “Verification experiment of stocking and disposal tasks by automatic shelf and mobile single-arm manipulation robot,” Advanced Robotics, Vol.36, Nos.17-18, pp. 900-919, 2022. https://doi.org/10.1080/01691864.2022.2104622
- [5] G. A. Garcia Ricardez et al., “Autonomous service robot for human-aware restock, straightening and disposal tasks in retail automation,” Advanced Robotics, Vol.36, Nos.17-18, pp. 936-950, 2022. https://doi.org/10.1080/01691864.2022.2109429
- [6] R. Tomikawa et al., “Development of display and disposal work system for convenience stores using dual-arm robot,” Advanced Robotics, Vol.36, No.23, pp. 1273-1290, 2022. https://doi.org/10.1080/01691864.2022.2136503
- [7] R. Sakai et al., “A mobile dual-arm manipulation robot system for stocking and disposing of items in a convenience store by using universal vacuum grippers for grasping items,” Advanced Robotics, Vol.34, Nos.3-4, pp. 219-234, 2020. https://doi.org/10.1080/01691864.2019.1705909
- [8] J. Mahler et al., “Dex-Net 3.0: Computing robust vacuum suction grasp targets in point clouds using a new analytic model and deep learning,” 2018 IEEE Int. Conf. on Robotics and Automation, pp. 5620-5627, 2018. https://doi.org/10.1109/ICRA.2018.8460887
- [9] H. Cao, H.-S. Fang, W. Liu, and C. Lu, “SuctionNet-1Billion: A large-scale benchmark for suction grasping,” IEEE Robotics and Automation Letters, Vol.6, No.4, pp. 8718-8725, 2021. https://doi.org/10.1109/LRA.2021.3115406
- [10] B. Yang, S. Atar, M. Grotz, B. Boots, and J. Smith, “DYNAMO-GRASP: DYNAMics-aware Optimization for GRASP point detection in suction grippers,” Proc. of the 7th Conf. on Robot Learning, pp. 2096-2112, 2023.
- [11] H. Fang, H-S. Fang, S. Xu, and C. Lu, “TransCG: A large-scale real-world dataset for transparent object depth completion and a grasping baseline,” IEEE Robotics and Automation Letters, Vol.7, No.3, pp. 7383-7390, 2022. https://doi.org/10.1109/LRA.2022.3183256
- [12] S. Sajjan et al., “Clear Grasp: 3D shape estimation of transparent objects for manipulation,” 2000 IEEE Int. Conf. on Robotics and Automation, pp. 3634-3642, 2020. https://doi.org/10.1109/ICRA40945.2020.9197518
- [13] E.-T. Baek, H.-J. Yang, S.-H. Kim, G. Lee, and H. Jeong, “Distance error correction in time-of-flight cameras using asynchronous integration time,” Sensors, Vol.20, No.4, Article No.1156, 2020. https://doi.org/10.3390/s20041156
- [14] C.-Y. Chai, Y.-P. Wu, and S.-L. Tsao, “Deep depth fusion for black, transparent, reflective and texture-less objects,” 2020 IEEE Int. Conf. on Robotics and Automation, pp. 6766-6772, 2020. https://doi.org/10.1109/ICRA40945.2020.9196894
- [15] Y. Cui, S. Schuon, S. Thrun, D. Stricker, and C. Theobalt, “Algorithms for 3D shape scanning with a depth camera,” IEEE Trans. on Pattern Analysis and Machine Intelligence, Vol.35, No.5, pp. 1039-1050, 2013. https://doi.org/10.1109/TPAMI.2012.190
- [16] C.-C. Chang, K.-C. Shih, H.-C. Ting, and Y.-S. Su, “Utilizing machine learning to improve the distance information from depth camera,” 2021 IEEE 3rd Eurasia Conf. on IOT, Communication and Engineering, pp. 405-408, 2021. https://doi.org/10.1109/ECICE52819.2021.9645639
- [17] R. A. Newcombe et al., “KinectFusion: Real-time dense surface mapping and tracking,” 2011 10th IEEE Int. Symp. on Mixed and Augmented Reality, pp. 127-136, 2011. https://doi.org/10.1109/ISMAR.2011.6092378
- [18] S. Izadi et al., “KinectFusion: Real-time 3D reconstruction and interaction using a moving depth camera,” Proc. of the 24th Annual ACM Symp. on User Interface Software and Technology, pp. 559-568, 2011. https://doi.org/10.1145/2047196.2047270
- [19] S. Doi, H. Koga, T. Seki, and Y. Okuno, “Novel proximity sensor for realizing tactile sense in suction cups,” 2020 IEEE Int. Conf. on Robotics and Automation, pp. 638-643, 2020. https://doi.org/10.1109/ICRA40945.2020.9196726
- [20] S. Aoyagi, M. Suzuki, T. Morita, T. Takahashi, and H. Takise, “Bellows suction cup equipped with force sensing ability by direct coating thin-film resistor for vacuum type robotic hand,” IEEE/ASME Trans. on Mechatronics, Vol.25, No.5, pp. 2501-2512, 2020. https://doi.org/10.1109/TMECH.2020.2982240
- [21] J. Lee, S. D. Lee, T. M. Huh, and H. S. Stuart, “Haptic search with the smart suction cup on adversarial objects,” IEEE Trans. on Robotics, Vol.40, pp. 226-239, 2024. https://doi.org/10.1109/TRO.2023.3331063
- [22] H. J. Lee et al., “An electronically perceptive bioinspired soft wet-adhesion actuator with carbon nanotube-based strain sensors,” ACS Nano, Vol.15, No.9, pp. 14137-14148, 2021. https://doi.org/10.1021/acsnano.1c05130
- [23] R. Miura, K. Fujita, and T. Tasaki, “Increasing the graspable objects by controlling the errors in the grasping points of a suction pad unit and selecting an optimal hand,” 2024 IEEE/SICE Int. Symp. on System Integration, pp. 196-201, 2024. https://doi.org/10.1109/SII58957.2024.10417393
- [24] K.-T. Yu et al., “A summary of Team MIT’s approach to the Amazon Picking Challenge 2015,” arXiv:1604.03639, 2016. https://doi.org/10.48550/arXiv.1604.03639
- [25] C. Hernandez et al., “Team Delft’s robot winner of the Amazon Picking Challenge 2016,” RoboCup 2016: Robot World Cup XX, pp. 613-624, 2016. https://doi.org/10.1007/978-3-319-68792-6_51
- [26] H. Okada, T. Inamura, and K. Wada, “What competitions were conducted in the service categories of the World Robot Summit?,” Advanced Robotics, Vol.33, No.17, pp. 900-910, 2019. https://doi.org/10.1080/01691864.2019.1663608
- [27] K. Wada, “New robot technology challenge for convenience store,” 2017 IEEE/SICE Int. Symp. on System Integration, pp. 1086-1091, 2017. https://doi.org/10.1109/SII.2017.8279367
- [28] J. J. Kuffner and S. M. LaValle, “RRT-connect: An efficient approach to single-query path planning,” Proc. of the 2000 IEEE Int. Conf. on Robotics and Automation, Vol.2, pp. 995-1001, 2000. https://doi.org/10.1109/ROBOT.2000.844730
- [29] A. Kirillov et al., “Segment Anything,” 2023 IEEE/CVF Int. Conf. on Computer Vision, pp. 3992-4003, 2023. https://doi.org/10.1109/ICCV51070.2023.00371
- [30] M. A. Fischler and R. C. Bolles, “Random sample consensus: A paradigm for model fitting with applications to image analysis and automated cartography,” Communications of the ACM, Vol.24, No.6, pp. 381-395, 1981. https://doi.org/10.1145/358669.358692
- [31] S. Liu, W. Dong, Z. Ma, and X. Sheng, “Adaptive aerial grasping and perching with dual elasticity combined suction cup,” IEEE Robotics and Automation Letters, Vol.5, No.3, pp. 4766-4773, 2020. https://doi.org/10.1109/LRA.2020.3003879
- [32] T. McCulloch and D. Herath, “Towards a universal gripper – On the use of suction in the context of the Amazon Robotic Challenge,” Australasian Conf. on Robotics and Automation 2016, pp. 153-160, 2016.
This article is published under a Creative Commons Attribution-NoDerivatives 4.0 Internationa License.