Review:
Current Status and Future Trends on Robot Vision Technology
Manabu Hashimoto*, Yukiyasu Domae**, and Shun’ichi Kaneko***
*Chukyo University
101-2 Yagoto-Honmachi, Showa-ku, Nagoya, Aichi 466-8666, Japan
**Mitsubishi Electric Corporation
8-1-1 Tsukaguchi, Hon-machi, Amagasaki, Hyogo 661-8661, Japan
***Hokkaido University
Kita-14, Nishi-9, Kita-ku, Sapporo 060-0814, Japan
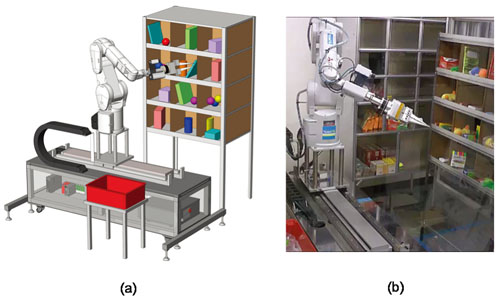
Intelligent robot classifying randomly stacked items in bin: (a) illustration of robot and setup and (b) actual robot system
- [1] P. J. Besl and N. D. McKay, “A Method for Registration of 3-D Shapes,” IEEE Trans. Pattern Analysis and Machine Intelligence (PAMI), Vol.14, No.2, pp. 239-256, 1992.
- [2] S. Granger and X. Pennec, “Multi-scale EM-ICP: A Fast and Robust Approach for Surface Registration,” European Conf. on Computer Vision, Vol.2353, pp. 418-432, 2002.
- [3] D. Chetverikov, D. Svirko, D. Stepanov, and P. Krsek, “The Trimmed Iterative Closest Point Algorithm,” Proc. Int. Conf. on Pattern Recognition, Vol.3, pp. 545-548, 2002.
- [4] T. ZinBer, J. Schmidt, and H. Niemann, “A Refind ICP Algorithm for Robust 3-D Correspondence Estimation,” Proc. Int. Conf. on Image Processing, Vol.3, pp. II-695-8, 2003.
- [5] S. Kaneko, T. Kondo, and A. Miyamoto, “Robust Matching of 3D Contours using Iterative Closest Point Algorithm Improved by M-estimation,” Pattern Recognition, Vol.36, Issue 9, pp. 2041-2047, 2003.
- [6] A. W. Fitzgibbon, “Robust Registration of 2D and 3D points sets,” Image and Vision Computing, Vol.21, pp. 1145-1153, 2003.
- [7] J. M. Phillps, R. Liu, and C. Tomasi, “Outlier Robust ICP for Minimizing Fractional RMSD,” Int. Conf. on 3-D Digital Imaging and Modeling, pp. 427-434, 2007.
- [8] A. Nuchter, K. Lingemann, and J. Hertzberg, “Cached K-d Tree Search for ICP Algorithms,” Int. Conf. on 3-D Digital Imaging and Modeling, pp. 419-426, 2007.
- [9] K. Tateno, D. Kotake, and S. Uchiyama, “A Model Fitting Method Using Intensity and Range Images for Bin-Picking Applications,” IEICE Trans. Inf.& Syst., Vol.J94-D, No.8, pp. 1410-1422, 2011 (in Japanese).
- [10] K. Ikeuchi and S. B. Kang, “Assembly Plan from Observation,” AAAI Technical Report FS-93-04, pp. 115-119, 1993.
- [11] H. Murase and S. K. Nayar, “3D Object Recognition from Appearance – Parametric Eigenspace Method –,” IEICE Trans. Inf.& Syst., Vol.J77-D-II, No.11, pp. 2179-2187, 1994 (in Japanese).
- [12] S. Ando, Y. Kusachi, A. Suzuki, and K. Arakawa, “Pose Estimation of 3D Object Using Support Vector Regression,” IEICE Trans. Inf.& Syst., Vol.J89-D, No.8, pp. 1840-1847, 2006 (in Japanese).
- [13] Y. Shibata and M. Hashimoto, “An Extended Method of the Parametric Eigenspace Method by Automatic Background Elimination,” Proc. Korea-Japan Joint Workshop on Frontiers of Computer Vision, pp. 246-249, 2013.
- [14] H. Yonezawa, H. Koichi et al., “Long-term operational experience with a robot cell production system controlled by low carbon-footprint Senju (thousand-handed) Kannon Model robots and an approach to improving operating efficiency,” Proc. of Automation Science and Engineering, pp. 291-298, 2011.
- [15] H. Do, T. Choi et al., “Automaton of cell production system for cellular phones usng dual-arm robots,” J. of Advanced Manufacturing Technology, Vol.83, No.5, pp. 1349-1360, 2016.
- [16] F. Tombari, S. Salti, and L. D. Stefano, “Unique Signatures of Histograms for Local Surface Description,” European Conf. on Computer Vision, pp. 356-369, 2010.
- [17] B. Drost, M. Ulrich, N. Navab, and S. Ilic, “Model Globally, Match Locally: Efficient and Robust 3D Object Recognition,” IEEE Computer Vision and Pattern Recognition, pp. 998-1005, 2010.
- [18] C. Choi, Y. Taguchi, O. Tuzel, M. Liu, and S. Ramalingam, “Voting-Based Pose Estimation for Robotic Assembly Using a 3D Sensor,” IEEE Int. Conf. on Robotics and Automation, pp. 1724-1731, 2012.
- [19] S. Akizuki and M. Hashimoto, “High-speed and Reliable Object Recognition Using Distinctive 3-D Vector-Pairs in a Range Image,” Int. Symposium on Optomechatronic Technologies (ISOT), pp. 1-6, 2012.
- [20] A. Mian, M. Bennamoun, and R. Owens, “On the Repeatability and Quality of Keypoints for Local Feature-based 3D Object Retrieval from Cluttered Scenes,” Int. J. of Computer Vision, Vol.89, Issue 2-3, pp. 348-361, 2010.
- [21] Y. Guo, F. Sohei, M. Bennamoun, M. Lu, and J. Wan, “Rotational Projection Statistics for 3D Local Surface Description and Object Recognition,” Int. J. of Computer Vision, Vol.105, Issue 1, pp. 63-86, 2013.
- [22] S. Takei, S. Akizuki, and M. Hashimoto, “SHORT: A Fast 3D Feature Description based on Estimating Occupancy in Spherical Shell Regions,” Int. Conf. on Image and Vision Computing New Zealand (IVCNZ), 2015.
- [23] S. Akizuki and M. Hashimoto, “DPN-LRF: A Local Reference Frame for Robustly Handling Density Differences and Partial Occlusions,” Int. Symposium on Visual Computing (ISVC), LNCS 9474, Part I, pp. 878-887, 2015.
- [24] H. Kayaba, S. Kaneko, H. Takauji, M. Toda, K. Kuno, and H. Suganuma, “Robust Matching of Dot Cloud Data Based on Model Shape Evaluation Oriented to 3D Defect Recognition,” IEICE Trans. D, Vol.J95-D, No.1, pp. 97-110, 2012.
- [25] S. Kaneko, T. Kondo, and A. Miyamoto, “Robust matching of 3D contours using iterative closest point algorithm improved by M-estimation,” Pattern Recognition, Vol.36, pp. 2041-2047, 2003.
- [26] Y. Domae, H. Okuda, Y. kitaaki, Y. Kimura, H. Takauji, K. Sumi, and S. Kaneko, “3-D Sensing for Flexible Linear Object Alignment in Robot Cell Production System,” J. of Robotics and Mechatronics, Vol.22, No.1, pp. 100-111, 2010.
- [27] Y. Domae, S. Kawato et al., “Self-calibration of Hand-eye Coordinate Systems by Five Observations of an Uncalibrated Mark,” CIEEJ Trans. On Electronics, Information and Systems, Vol.132, No.6, pp. 968-974, 2011 (in Japanese).
- [28] R. Haraguchi, Y. Domae et al., “Development of Production Robot System that can Assemble Products with Cable and Connector,” J. of Robotics and Mechatronics, Vol.23, No.6, pp. 939-950, 2011.
- [29] A. Noda, Y. Domae et al., “Bin-picking System for General Objects,” J. of the Robotics Society of Japan, Vol.33, No.5, pp. 387-394, 2015 (in Japanese).
- [30] Y. Domae, H. Okuda et al., “Fast graspability evaluation on single depth maps for bin picking with general grippers,” Proc. of ICRA, pp. 1997-2004, 2014.
- [31] N. Correll, K. E. Bekris et al., “Lessons from the Amazon Picking Chllenge,” CarXiv:1601.05484, 2016.
- [32] R. Jonschkowski, C. Eppner et al., “Probabilistic Multi-Class Segmentation for the Amazon Picking Challenge,” http://dx.doi.org/10.14279/depositonce-5051, 2016.
- [33] I. Lenz, H. Lee et al., “Deep Learning for Detecting Robotic Grasps,” Proc. of ICRA, pp.1957-1964, 2016.
- [34] L. Pinto and A. Gupta, “Supersizing Self-supervision: Learning to Grasp from 50K Tries and 700 Robot Hours,” arxiv:1509.06825, 2015.
- [35] S. Levine, P. Pastor et al., “Learning Hand-Eye Coordination for Robotic Grasping with Deep Learning and Large-Scale Data Collection,” arXiv:1603.02199, 2016.
- [36] C. Finn, X. Y. Tan et al., “Deep Spatial Autoencoders for Visuomotor Learning,” arXiv:1509.06113, 2016.
- [37] T. Komuro, Y. Senjo, K. Sogen, S. Kagami, and M. Ishikawa, “Real-Time Shape Recognition Using a Pixel-Parallel Processor,” J. of Robotics and Mechatronics, Vol.17, No.4, pp. 410-419, 2005.
- [38] T. Senoo, Y. Yamakawa, Y. Watanabe, H. Oku, and M. Ishikawa, “High-Speed Vision and its Application Systems,” J. of Robotics and Mechatronics, Vol.26, No.3, pp. 287-301, 2014.
This article is published under a Creative Commons Attribution-NoDerivatives 4.0 Internationa License.