Research Paper:
Prediction of Fetal Growth Restriction Using Placental Image Features in BOLD MRI
Kentaro Nishida*, Kento Morita*
, Naosuke Enomoto**
, Shoichi Magawa***
, Masafumi Nii***
, and Tetsushi Wakabayashi*
*Department of Information Engineering, Graduate School of Engineering, Mie University
1577 Kurimamachiya-cho, Tsu, Mie 514-8507, Japan
**Department of Obstetrics and Gynecology, Mie Chuo Medical Center
2158-5 Hisai-myojin-cho, Tsu, Mie 514-1101, Japan
***Department of Obstetrics and Gynecology, Faculty of Medicine, Mie University
2-174 Edobashi, Tsu, Mie 514-8507, Japan
Fetal growth restriction (FGR) is a disease during pregnancy that increases the risk of preterm birth and perinatal death. Currently, the diagnosis of FGR relies on ultrasonography-based estimated fetal body weight (EFBW). However, EFBW can only provide an indirect assessment of FGR because a low EFBW is only a result of growth restriction. Recent research has indicated that placental oxygenation function is a key indicator of fetal growth; however, its assessment through ultrasonography is impractical. Techniques other than ultrasonography for placental function have been investigated, and a significant difference in placental oxygenation function between FGR and non-FGR cases has been demonstrated using blood oxygen level-dependent magnetic resonance imaging (BOLD MRI). BOLD MRI can visualize oxygenation in vivo, and may be useful as a marker for the direct assessment of placental oxygenation function. However, visual assessment of placental oxygenation in BOLD MRI is challenging, even for experts, because of the complexity of analyzing the signal intensity on MRI. In this study, we proposed an automated method for predicting FGR by utilizing placental image features extracted from BOLD MRI during oxygen administration. In addition to the FGR/non-FGR classification method, we propose a placental region segmentation method to reduce the manual annotation burden. The proposed segmentation method achieved a Dice coefficient of 0.809, outperforming other deep learning methods. In the FGR/non-FGR classification experiments, conducted with four-fold cross-validation on 22 subjects, the highest performance was obtained using a pre-trained ResNet50 combined with a fully connected layer with transfer learning as a feature extractor (subject-wise accuracy of 0.908, ROC-AUC of 0.927, and F1 score of 0.922). These results demonstrate that placental image features extracted from BOLD MRI can effectively differentiate between FGR and non-FGR cases, suggesting the potential for a direct and automated approach to assess FGR through placental oxygenation function.
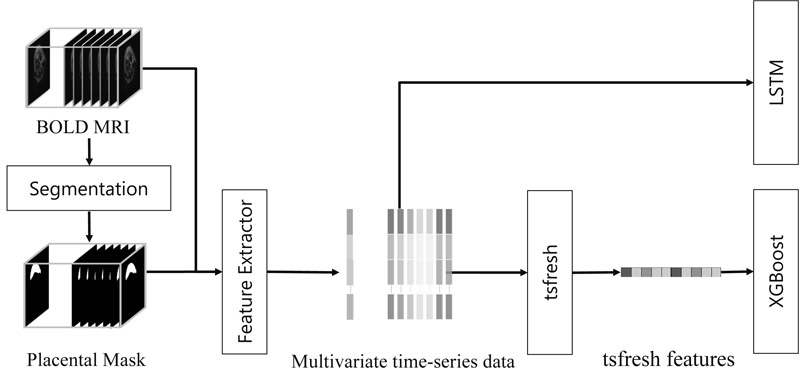
Flow of methods for FGR estimation
- [1] L. M. M. Nardozza et al., “Fetal growth restriction: Current knowledge,” Archives of Gynecology and Obstetrics, Vol.295, No.5, pp. 1061-1077, 2017. https://doi.org/10.1007/s00404-017-4341-9
- [2] V. Flenady et al., “Major risk factors for stillbirth in high-income countries: A systematic review and meta-analysis,” The Lancet, Vol.377, No.9774, pp. 1331-1340, 2011. https://doi.org/10.1016/S0140-6736(10)62233-7
- [3] G. J. Burton and E. Jauniaux, “Pathophysiology of placental-derived fetal growth restriction,” American J. of Obstetrics & Gynecology, Vol.218, No.2, Supplement, pp. S745-S761, 2018. https://doi.org/10.1016/j.ajog.2017.11.577
- [4] H. A. Wollmann, “Intrauterine growth restriction: Definition and etiology,” Hormone Research, Vol.49, No.Suppl. 2, pp. 1-6, 1998. https://doi.org/10.1159/000053079
- [5] M. Kovo et al., “The placental factor in early- and late-onset normotensive fetal growth restriction,” Placenta, Vol.34, No.4, pp. 320-324, 2013. https://doi.org/10.1016/j.placenta.2012.11.010
- [6] T. Y. Khong, F. De Wolf, W. B. Robertson, and I. Brosens, “Inadequate maternal vascular response to placentation in pregnancies complicated by pre-eclampsia and by small-for-gestational age infants,” BJOG: An Int. J. of Obstetrics & Gynaecology, Vol.93, No.10, pp. 1049-1059, 1986. https://doi.org/10.1111/j.1471-0528.1986.tb07830.x
- [7] R. Pijnenborg, L. Vercruysse, and M. Hanssens, “The uterine spiral arteries in human pregnancy: Facts and controversies,” Placenta, Vol.27, Nos.9-10, pp. 939-958, 2006. https://doi.org/10.1016/j.placenta.2005.12.006
- [8] S. Magawa et al., “Evaluation of placental oxygenation in fetal growth restriction using blood oxygen level-dependent magnetic resonance imaging,” Placenta, Vol.126, pp. 40-45, 2022. https://doi.org/10.1016/j.placenta.2022.06.005
- [9] F. Song et al., “Predicting the risk of fetal growth restriction by radiomics analysis of the placenta on T2WI: A retrospective case-control study,” Placenta, Vol.134, pp. 15-22, 2023. https://doi.org/10.1016/j.placenta.2023.02.007
- [10] R. Li et al., “A hybrid model for fetal growth restriction assessment by automatic placental radiomics on T2-weighted MRI and multifeature fusion,” J. of Magnetic Resonance Imaging, Vol.61, No.1, pp. 494-504, 2025. https://doi.org/10.1002/jmri.29399
- [11] S. M. Abulnaga et al., “Automatic segmentation of the placenta in BOLD MRI time series,” Proc. of the 7th Int. Workshop on Perinatal, Preterm and Paediatric Image Analysis, 2022. https://doi.org/10.1007/978-3-031-17117-8_1
- [12] H. Minakami et al., “Guidelines for obstetrical practice in Japan: Japan Society of Obstetrics and Gynecology (JSOG) and Japan Association of Obstetricians and Gynecologists (JAOG) 2011 edition,” The J. of Obstetrics and Gynaecology Research, Vol.37, No.9, pp. 1174-1197, 2011. https://doi.org/10.1111/j.1447-0756.2011.01653.x
- [13] D. G. Lowe, “Distinctive image features from scale-invariant keypoints,” Int. J. of Computer Vision, Vol.60, No.2, pp. 91-110, 2004. https://doi.org/10.1023/B:VISI.0000029664.99615.94
- [14] B. Koçak, E. Ş. Durmaz, E. Ateş, and Ö. Kılıçkesmez, “Radiomics with artificial intelligence: A practical guide for beginners,” Diagnostic and Interventional Radiology, Vol.25, No.6, pp. 485-495, 2019. https://doi.org/10.5152/dir.2019.19321
- [15] J. J. M. van Griethuysen, “Computational radiomics system to decode the radiographic phenotype,” Cancer Research, Vol.77, No.21, pp. e104-e107, 2017. https://doi.org/10.1158/0008-5472.CAN-17-0339
- [16] K. Simonyan and A. Zisserman, “Very deep convolutional networks for large-scale image recognition,” arXiv:1409.1556, 2014. https://doi.org/10.48550/arXiv.1409.1556
- [17] K. He, X. Zhang, S. Ren, and J. Sun, “Deep residual learning for image recognition,” 2016 IEEE Conf. on Computer Vision and Pattern Recognition, pp. 770-778, 2016. https://doi.org/10.1109/CVPR.2016.90
- [18] J. Deng et al., “ImageNet: A large-scale hierarchical image database,” 2009 IEEE Conf. on Computer Vision and Pattern Recognition, pp. 248-255, 2009. https://doi.org/10.1109/CVPR.2009.5206848
- [19] H.-C. Shin et al., “Deep convolutional neural networks for computer-aided detection: CNN architectures, dataset characteristics and transfer learning,” IEEE Trans. on Medical Imaging, Vol.35, No.5, pp. 1285-1298, 2016. https://doi.org/10.1109/TMI.2016.2528162
- [20] M. Christ, N. Braun, J. Neuffer, and A. W. Kempa-Liehr, “Time Series FeatuRe Extraction on basis of Scalable Hypothesis tests (tsfresh – A Python package),” Neurocomputing, Vol.307, pp. 72-77, 2018. https://doi.org/10.1016/j.neucom.2018.03.067
- [21] S. Hochreiter and J. Schmidhuber, “Long short-term memory,” Neural Computation, Vol.9, No.8, pp. 1735-1780, 1997. https://doi.org/10.1162/neco.1997.9.8.1735
- [22] T. Chen and C. Guestrin, “XGBoost: A scalable tree boosting system,” Proc. of the 22nd ACM SIGKDD Int. Conf. on Knowledge Discovery and Data Mining, pp. 785-794, 2016. https://doi.org/10.1145/2939672.2939785
- [23] O. Ronneberger, P. Fischer, and T. Brox, “U-Net: Convolutional networks for biomedical image segmentation,” Proc. of the 18th Int. Conf. on Medical Image Computing and Computer-Assisted Intervention, Part 3, pp. 234-241, 2015. https://doi.org/10.1007/978-3-319-24574-4_28
This article is published under a Creative Commons Attribution-NoDerivatives 4.0 Internationa License.