Paper:
Robust and Automatic Skyline Detection Algorithm Based on MSSDN
Fan Guo*, Yuxiang Mai**, Jin Tang*,, Yu Huang*, and Lijun Zhu*
*School of Automation, Central South University
Changsha 410083, China
**School of Computer Science and Engineering, Central South University
Changsha 410083, China
Corresponding author
Automatic detection of the skyline plays an important role in several applications, such as visual geo-localization, flight control, port security, and mountain peak recognition. Existing skyline detection methods are mostly used under common weather conditions; however, they do not consider bad weather situations, such as rain, which limits their application in real scenes. In this paper, we propose a multi-stream-stage DenseNet to detect skyline automatically under different weather conditions. This model fully considers the adverse factors influencing the skyline and outputs a probability graph of the skyline. Finally, a dynamic programming algorithm is implemented to detect the skyline in images accurately. A comparison with the existing state-of-the-art methods proves that the proposed model shows a good performance under rainy or common weather conditions and exhibits the best detection precision for the public database.
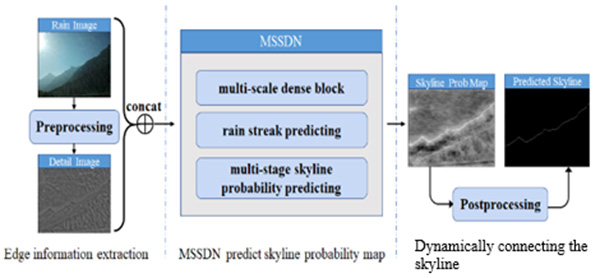
Algorithm framework for skyline detection
- [1] C.-C. Chiu, Y.-J. Liu, S.-Y. Chiu et al., “A skyline detection algorithm for use in different weather and environmental conditions,” Proc. of the IEEE Int. Conf. on Electro Information Technology (EIT), pp. 680-685, Grand Forks, USA, May 19-21, 2016.
- [2] Y.-J. Liu, C.-C. Chiu, and Y.-H. Yang, “A Robust Vision-Based Skyline Detection Algorithm Under Different Weather Conditions,” IEEE Access, Vol.5, pp. 22992-23009, 2017.
- [3] M. Ayadi, L. Suta, M. Scuturici, S. Miguet, and C. B. Amar, “A parametric algorithm for skyline extraction,” Proc. of the 17th Int. Conf. on Advanced Concepts for Intelligent Vision Systems, pp. 604-615, Lecce, Italy, October 24-27, 2016.
- [4] W.-N. Lie, T C.-I. Lin, T.-C. Lin et al., “A robust dynamic programming algorithm to extract skyline in images for navigation,” Pattern Recognition Letters, Vol.26, No.2, pp. 221-230, 2005.
- [5] S. Fefilatyev, V. Smarodzinava, L. O. Hall et al., “Horizon Detection Using Machine Learning Techniques,” Proc. of Int. Conf. on Machine Learning and Applications, pp. 17-21, Orlando, USA, December 14-16, 2006.
- [6] Y.-L. Hung, C.-W. Su, Y.-H. Chang et al., “Skyline localization for mountain images,” Proc. of the IEEE Int. Conf. on Multimedia and Expo, pp. 1-6, San Jose, USA, July 15-19, 2013.
- [7] T. Ahmad, G. Bebis, M. Nicolescu et al., “An Edge-Less Approach to Horizon Line Detection,” Proc. of the IEEE Int. Conf. on Machine Learning and Applications, pp. 1095-1102, Miami, USA, December 9-11, 2015.
- [8] T. Ahmad, G. Bebis, E. E. Regentova et al., “A Machine Learning Approach to Horizon Line Detection Using Local Features,” Proc. of the 9th Int. Symp. on Advances in Visual Computing, pp. 181-193, Rethymnon, Greece, July 29-31, 2013.
- [9] A. P. Yazdanpanah, E. E. Regentova, V. Muthukumar et al., “Real-Time Horizon Line Detection Based on Fusion of Classification and Clustering,” Int. J. of Computer Applications, Vol.121, No.10, pp. 5-11, No.10, 2015.
- [10] L. Porzi, S. R. Buló. Lanz, P. Valigi, and E. Ricci, “Learning Contours for Automatic Annotations of Mountains Pictures on a Smartphone,” Proc. of the ACM/IEEE Int. Conf. on Distributed Smart Cameras, Article No.13, pp. 1-6, November 4-7, 2014.
- [11] R. Verbickas and A. Whitehead, “Sky and Ground Detection Using Convolutional Neural Networks,” Proc. of Int. Conf. on Machine Vision and Machine Learning, Paper No.64, pp. 64-1-64-10, Prague, Czech Republic, August 14-15, 2014.
- [12] A. P. Yazdanpanah, E. E. Regentova, A. K. Mandava et al., “Sky Segmentation by Fusing Clustering with Neural Networks,” Proc. of the 9th Int. Symp. on Visual Computing, Vol.8034, pp. 663-672, Rethymnon, Greece, July 29-31, 2013.
- [13] L. Porzi, S. R. Bulò, and E. Ricci, “A Deeply-Supervised Deconvolutional Network for Horizon Line Detection,” Proc. of the 24th ACM Int. Conf. on Multimedia, pp. 137-141, Amsterdam, The Netherlands, October 15-19, 2016.
- [14] O. Ronneberger, P. Fischer, and T. Brox, “U-Net: Convolutional Networks for Biomedical Image Segmentation,” Proc. of Int. Conf. on Medical Image Computing and Computer-Assisted Intervention, pp. 234-241, Munich, Germany, October 5-9, 2015.
- [15] D. Frajberg, P. Fraternali, and R. N. Torres, “Convolutional Neural Network for Pixel-Wise Skyline Detection,” Proc. of the 26th Int. Conf. on Artificial Neural Networks, pp. 12-20, Alghero, Italy, September 11-14, 2017.
- [16] T. Ahmad, P. Campr, M. Čadik et al., “Comparison of Semantic Segmentation Approaches for Horizon/Sky Line Detection,” Proc. of Int. Joint Conf. on Neural Networks, pp. 4436-4443, Anchorage, USA, May 14-19, 2017.
- [17] O. Saurer, G. Baatz, K. Köser et al., “Image Based Geo-Localization in the Alps,” Int. J. of Computer Vision, Vol.116, No.3, pp. 213-225, 2016.
- [18] J. Dai, L. Yi, K. He et al., “R-FCN: Object Detection via Region-Based Fully Convolutional Networks,” Proc. of the 30th Conf. on Neural Information Processing Systems, pp. 379-387, Barcelona, Spain, December 5-10, 2016.
- [19] V. Badrinarayanan, A. Kendall, and R. Cipolla, “SegNet: A Deep Convolutional Encoder-Decoder Architecture for Scene Segmentation,” IEEE Trans. on Pattern Analysis and Machine Intelligence, Vol.39, No.12, pp. 2481-2495, 2017.
- [20] K. He, X. Zhang, S. Q. Ren, and J. Sun, “Identity mappings in deep residual networks,” Proc. of the 14th European Conf. on Computer Vision, pp. 630-645, Amsterdam, The Netherlands, October 11-14, 2016.
- [21] K. He, X. Zhang, S. Ren, and J. Sun, “Spatial pyramid pooling in deep convolutional networks for visual recognition,” Proc. of the European Conf. on Computer Vision, pp. 346-361, Zurich, Switzerland, September 6-12, 2014.
- [22] J. Long, E. Shelhamer, and T. Darrell, “Fully convolutional networks for semantic segmentation,” Proc. of the IEEE Conf. on Computer Vision and Pattern Recognition, pp. 3431-3440, Boston, USA, June 7-12, 2015.
- [23] Y. Liu, M.-M. Cheng, X. Hu et al., “Richer convolutional features for edge detection,” IEEE Trans. on Pattern Analysis and Machine Intelligence, Vol.41, No.8, pp. 1939-1946, 2019.
- [24] K. He, J. Sun, and X. O. Tang, “Guided image filtering,” IEEE Trans. on Pattern Analysis and Machine Intelligence, Vol.35, No.6, pp. 1397-1409, 2013.
- [25] D.-A. Huang, L.-W. Kang, Y.-C. F. Wang, and C.-W. Lin, “Self-learning based image decomposition with applications to single image denoising,” IEEE Trans. on Multimedia, Vol.16, No.1, pp. 83-93, 2014.
- [26] L.-W. Kang, C.-W. Lin, and Y.-H. Fu, “Automatic single-image-based rain streaks removal via image decomposition,” IEEE Trans. on Image Processing, Vol.21, No.4, pp. 1742-1755, 2012.
- [27] X. Fu, J. Huang, D. Zeng et al., “Removing rain from single images via a deep detail network,” Proc. of the IEEE Conf. on Computer Vision and Pattern Recognition, pp. 1715-1723, Honolulu, USA, July 21-26, 2017.
- [28] G. Huang, Z. Liu, L. van der Maaten et al., “Densely connected convolutional networks,” Proc. of the IEEE Conf. on Computer Vision and Pattern Recognition, pp. 2261-2269, Honolulu, USA, July 21-26, 2017.
- [29] S. Xie and Z. Tu, “Holistically-nested edge detection,” Proc. of the IEEE Int. Conf. on Computer Vision, pp. 1395-1403, Santiago, Chile, December 7-13, 2015.
- [30] J. Brejcha and M. Čadík, “GeoPose3K: mountain landscape database for camera pose estimation in outdoor environments,” Image and Vision Computing, Vol.66, pp. 1-14, 2017.
- [31] L.-C. Chen, G. Papandreou, F. Schroff et al., “Rethinking atrous convolution for semantic image segmentation,” arXiv preprint, arXiv:1706.05587, 2017.
- [32] H. Zhao, J. Shi, X. Qi et al., “Pyramid scene parsing network,” Proc. of the IEEE Conf. on computer vision and pattern recognition, pp. 6230-6239, Honolulu, USA, July 21-26, 2017.
- [33] K. He, X. Zhang, S. Ren et al., “Deep residual learning for image recognition,” Proc. of the IEEE Conf. on Computer Vision and Pattern Recognition, pp. 770-778, Las Vegas, USA, June 27-30, 2016.
This article is published under a Creative Commons Attribution-NoDerivatives 4.0 Internationa License.