Paper:
Navigation Model for a Robot as a Human Group Member to Adapt to Changing Conditions of Personal Space
Yotaro Fuse* and Masataka Tokumaru**
*Graduate School of Science and Engineering, Kansai University
3-3-35 Yamate-cho, Suita, Osaka 564-8680, Japan
**Kansai University
3-3-35 Yamate-cho, Suita, Osaka 564-8680, Japan
In the present paper, we propose a robotic model to help determine a robot’s position under the changing conditions of human personal space in a human-robot group. Recently, several attempts have been made to develop personal robots suitable for human communities. Determining a robot’s position is important not only to avoid collisions with humans but also to maintain a socially acceptable distance from them. Interpersonal space maintained by persons in a community depends on the particular context and situations. Therefore, robots need to determine their own positions while considering the positions of other persons and evaluating the changes made in their personal space. To address this problem, we proposed a robot navigation model and examined whether the experiment participants could distinguish the robot’s trajectory from the human’s trajectory in the experimental scenario. We prepared a scenario in which robots in a group needed to keep an appropriate distance in a three-dimensional space. The experiment participants provided their impressions on robot movements while watching the records representing the scenario. The results indicate that (1) a robot using the proposed model is able to follow the other group members and (2) the experiment participants were not sure whether the trajectories of the robots were controlled by humans and by the proposed model. Therefore, we conclude that the proposed model generates suitable trajectories in robot groups.
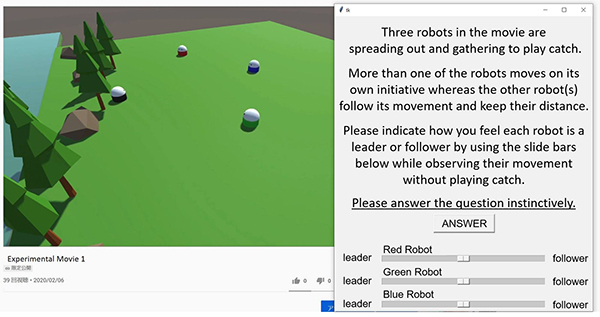
Environment in which a participant evaluated robots
- [1] F. Hegel et al., “Understanding Social Robots,” Proc. of the 2009 2nd Int. Conf. on Advances in Computer-Human Interactions, pp. 169-174, 2009.
- [2] T. Fong, I. Nourbakhsh, and K. Dautenhahn, “A survey of socially interactive robots,” Robotics and Autonomous Systems, Vol.42, Issues 3-4, pp. 143-166, 2003.
- [3] C. Breazeal, “Designing Sociable Robots,” MIT Press, 2004.
- [4] T. Kruse et al., “Human-aware robot navigation: A survey,” Robotics and Autonomous Systems, Vol.61, Issue 12, pp. 1726-1743, 2013.
- [5] J. Rios-Martinez, A. Spalanzani, and C. Laugier, “From Proxemics Theory to Socially-Aware Navigation: A Survey,” Int. J. of Social Robotics, Vol.7, Issue 2, pp. 137-153, 2015.
- [6] D. C. Feldman, “The Development and Enforcement of Group Norms,” The Academy of Management Review, Vol.9, No.1, pp. 47-53, 1984.
- [7] Y. Fuse, H. Takenouchi, and M. Tokumaru, “A Robot in a Human-Robot Group Learns Group Norms and Makes Decisions Through Indirect Mutual Interaction with Humans,” J. Adv. Comput. Intell. Intell. Inform., Vol.24, No.1, pp. 169-178, 2020.
- [8] E. Sundstrom and I. Altman, “Interpersonal relationships and personal space: Research review and theoretical model,” Human Ecology, Vol.4, Issue 1, pp. 47-67, 1976.
- [9] M. Cristani et al., “Towards Computational Proxemics: Inferring Social Relations from Interpersonal Distances,” Proc. of the 2011 IEEE 3rd Int. Conf. on Privacy, Security, Risk and Trust and 2011 IEEE 3rd Int. Conf. on Social Computing, pp. 290-297, 2011.
- [10] L. A. Hayduk, “Personal space: Understanding the simplex model,” J. of Nonverbal Behavior, Vol.18, Issue 3, pp. 245-260, 1994.
- [11] I. Chatterjee and A. Steinfeld, “Performance of a low-cost, human-inspired perception approach for dense moving crowd navigation,” Proc. of the 25th IEEE Int. Symp. on Robot and Human Interactive Communication (RO-MAN), pp. 578-585, 2016.
- [12] W. Chi et al., “A human-friendly robot navigation algorithm using the risk-RRT approach,” Proc. of the 2016 IEEE Int. Conf. on Real-time Computing and Robotics (RCAR), pp. 227-232, 2016.
- [13] X.-T. Truong, V. N. Yoong, and D. N. Trung, “Approaching humans in crowded and dynamic environments,” Proc. of the 2016 IEEE Int. Conf. on Advanced Intelligent Mechatronics (AIM), pp. 476-481, 2016.
- [14] F. Lindner, “A conceptual model of personal space for human-aware robot activity placement,” Proc. of the 2015 IEEE/RSJ Int. Conf. on Intelligent Robots and Systems (IROS), pp. 5770-5775, 2015.
- [15] Y. F. Chen et al., “Socially aware motion planning with deep reinforcement learning,” Proc. of the 2017 IEEE/RSJ Int. Conf. on Intelligent Robots and Systems (IROS), pp. 1343-1350, 2017.
- [16] J. Vroon et al., “Dynamics of social positioning patterns in group-robot interactions,” Proc. of the 2015 24th IEEE Int. Symp. on Robot and Human Interactive Communication (RO-MAN), pp. 394-399, 2015.
- [17] Y. Fuse, H. Takenouchi, and M. Tokumaru, “Evaluation of Robotic Navigation Model Considering Group Norms of Personal Space in Human-Robot Communities,” The Int. Conf. on Artificial Intelligence and Computational Intelligence (AICI2020), 2020.
- [18] E. Brunner and U. Munzel, “The Nonparametric Behrens-Fisher Problem: Asymptotic Theory and a Small-Sample Approximation,” Biometrical J., Vol.42, Issue 1, pp. 17-25, 2000.
- [19] S. Nakagawa and I. C. Cuthill, “Effect size, confidence interval and statistical significance: a practical guide for biologists,” Biological Reviews, Vol.82, Issue 4, pp. 591-605, 2007.
- [20] J. Cohen, “A power primer,” Psychological Bulletin, Vol.112, No.1, pp. 155-159, 1992.
This article is published under a Creative Commons Attribution-NoDerivatives 4.0 Internationa License.