Letter:
Evaluation Method for Complex Electromagnetic Environment
Yan Li*,, Yigang He**, and Baiqiang Yin**
*School of Information and Electrical Engineering, Hunan University of Science and Technology
Taoyuan Road, Xiangtan, Hunan 411201, China
**School of Electrical Engineering and Automation, Hefei University of Technology
No.193 Tunxi Road, Hefei, Anhui 230009, China
Corresponding author
To perform a complexity evaluation for an electromagnetic environment (EME), a new method based on the S-transform is proposed, which can simultaneously count the time occupancy, frequency occupancy, and energy occupancy in the time–frequency domain. The frequency coincidence, modulation similarity, and background noise intensity are selected as important evaluation indices, and their physical interpretations are analyzed and calculated. The Extreme Learning Machine (ELM) method is adopted to evaluate the environmental complexity. The proposed method (S-ELM) requires less training time and has a fast convergence rate. The simulation and experimental results confirm that the proposed method is accurate and efficient.
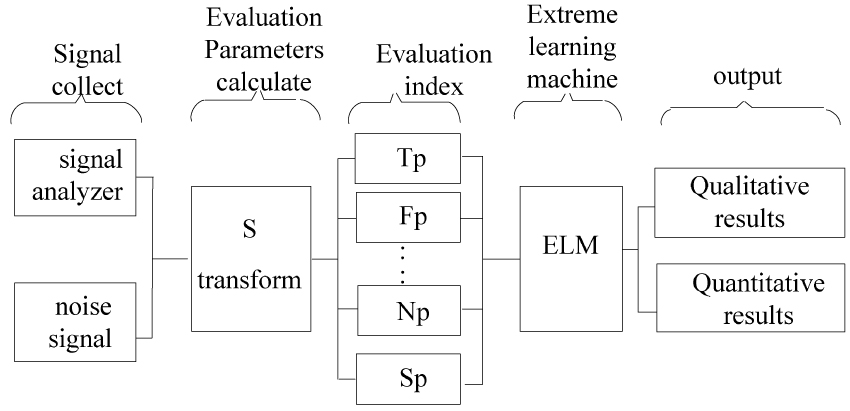
Flow chart of extreme learning machine to realize electromagnetic environment complexity evaluation
- [1] W. H. Tetley, “Analytical prediction of electromagnetic environments,” IEEE Trans. on Communications Systems, Vol.9, No.2, pp. 175-185, 1961.
- [2] Z. Wang, F. Wang, and Z. Zhao, “The battlefield electromagnetic environment complexity of objective analysis and evaluation,” Modern Defence Technology, Vol.3, pp. 19-25, 2010.
- [3] Z. Dong and Q. Li, “Complexity evaluation of artificial electromagnetism environment based on AHP,” Command Control & Simulation, Vol.30, No.5, pp. 106-110, 2008.
- [4] H. Dai and D. Su, “Study of the complexity evaluationon electromagnetism environment,” J. of Microwaves, Vol.25, No.3, pp. 25-27, 2009.
- [5] Z. Wang and J. He, “The research of battlefield electromagnetic environment complexity quantitative evaluation method,” Electronic Information Warfare Technology, Vol.2, pp. 50-53, 2008.
- [6] S. Lian, X. Li, Y. Shen et al., “Study on complexity prediction of electromagnetic environment based on GM(1,1),” Fire Control & Command Control, Vol.37, No.11, pp. 33-36, 2012.
- [7] Y. Gu, Z. Zhang, W. Wang et al., “Research and simulation implementation of electromagnetic environment complex evaluation algorithm,” J. of System Simulation, Vol.24, No.2, pp. 394-397, 2012.
- [8] Q. H. Vu, M. Li, and K. H. Ryu, “Assessment of Electromagnetic Frequency Sounding Problem in a Near Surface Geological Environment,” J. Adv. Comput. Intell. Intell. Inform., Vol.17, No.4, pp. 604-610, 2013.
- [9] S. Ventosa, C. Simon, M. Schimmel et al., “The S-transform from a wavelet point of view,” IEEE Trans. Signal Process., Vol.56, No.7, pp. 2771-2780, 2008.
- [10] G.-B. Huang, Q.-Y. Zhu, and C.-K. Siew, “Extreme learning machine: a new learning scheme of feedforward neural networks,” IEEE Int. Joint Conf. on Neural Networks, Vol.2, pp. 985-990, 2004.
- [11] C. Chen, K. Li, M. Duan, and K. Li, “Chapter 6 – Extreme Learning Machine and Its Applications in Big Data Processing,” H.-H. Hsu et al. (Eds.), “Big Data Analytics for Sensor-Network Collected Intelligence: Intelligent Data-Centric Systems,” pp. 117-150, Academic Press, 2016.
- [12] Y. Peng and B. L. Lu, “Discriminative extreme learning machine with supervised sparsity preserving for image classification,” Neurocomputing, Vol.261, pp. 242-252, 2017.
- [13] W. Yu et al., “The Faults Diagnostic Analysis for Analog Circuit Based on FA-TM-ELM,” J. of Electronic Testing, Vol.32, No.4, pp. 1-7, 2016.
- [14] L. Wang, W. Sun, and G. Pan, “An evaluating quickly method for electromagnetic environment complexity,” J. of Electronics & Information Technology, Vol.32, No.12, pp. 2942-2947, 2010.
- [15] H.-T. Lin, T.-J. Liang, and S.-M. Chen, “Estimation of battery state of health using probabilistic neural network,” IEEE Trans. on Industrial Informatics, Vol.9, No.2, pp. 679-684, 2013.
This article is published under a Creative Commons Attribution-NoDerivatives 4.0 Internationa License.