Paper:
Analysis of Water Quality Conditions of Lake Hachiroko Using Fuzzy C-Means
Kai Matsui, Yoichi Kageyama, and Hiroshi Yokoyama
Akita University
1-1 Tegata Gakuen-machi, Akita-shi, Akita 010-8502, Japan
Corresponding author
Lake Hachiroko, Japan, has many water quality issues, evident from phenomena such as green algae blooms. Understanding the details of the surface water quality of the lake, and the effect of seasons on the quality, is important. In our previous studies, we conducted fuzzy regression analysis of remote sensing data and direct measurements of water quality. The results showed that estimation maps of water quality were well created, using only five data points of the water quality parameters. To obtain maps that are in good agreement with the experimental data, remote sensing data and water quality values should be acquired simultaneously. However, performing such simultaneous observations can affect the preparation of the water quality estimation maps. We overcame this obstacle by using fuzzy c-means clustering (FCM), and considered the effect of specific disturbances and uncertainties on the remote sensing data. Furthermore, FCM using only remote sensing data creates estimation maps in which relative water surface conditions are classified. Therefore, determining the relationship between FCM results and water quality facilitates the creation of low-cost, high-frequency water quality estimation maps. Our results indicated that FCM was particularly effective in determining the presence of suspended solids (SS) during water quality analysis. However, the relationship between FCM results and water quality has not been determined in detail. In this study, we analyzed the water quality conditions of Lake Hachiroko with FCM using the data collected by the Advanced Space-borne Thermal Emission and Reflection Radiometer on Terra and, the Operational Land Imager on Landsat-8. In addition, FCM results were compared with the maps created by fuzzy regression analysis and the actual conditions of water pollution. The results indicated that (i) the maps created using FCM are effective in determining the water surface conditions, (ii) the FCM maps using data obtained during August and September have a strong relationship with biochemical oxygen demand (BOD) and SS, and (iii) the FCM maps using data obtained during May and June have a strong relationship with chemical oxygen demand (COD), SS, and total nitrogen (T-N).
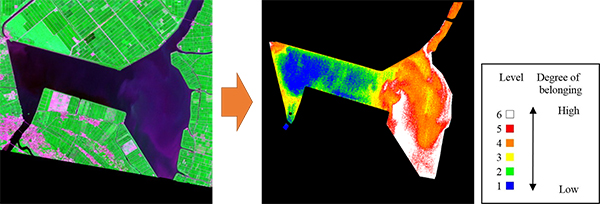
Estimated water quality map of Lake Hachiroko using fuzzy c-means
- [1] C. Giardino, V. E. Brando, A. G. Dekker, N. Strömbeck, and G. Candiani, “Assessment of Water Quality in Lake Garda (Italy) Using Hyperion,” Remote Sensing of Environment, Vol.109, pp. 183-195, 2007.
- [2] O. Youichi, M. Bunkei, F. Takehiko, M. Kazuo, and I. Akio, “Application of Spectral Decomposition Algorithm for Mapping Water Quality in a Turbid Lake (Lake Kasumigaura, Japan) from Landsat TM Data,” ISPRS J. of Photogrammetry and Remote Sensing, Vol.64, pp. 73-85, 2009.
- [3] M. Nishida and K. Otsuka, “Application of Fuzzy Regression Model on Water Quality Analysis with Satellite Image Data and Drawing of Estimation Map,” IEEJ Trans. on Electronics, Information and Systems, Vol.115-C, pp. 381-388, 1995.
- [4] Y. Kageyama and M. Nishida, “Water Quality Analysis Based on Remote Sensing Data and Numerical Model,” J. of Geography, Vol.109, No.1, pp. 27-36, 2000.
- [5] D. Wang, Y. Kageyama, M. Nishida, and H. Shirai, “Algorithm to Analyze Water Quality Conditions of Lake Hachiroko using Textures of JERS-1 SAR Data,” Int. J. of the Society of Materials Engineering for Resources, Vol.18, No.2, pp. 51-58, 2012.
- [6] D. Wang, Y. Kageyama, M. Nishida, H. Shirai, and C. Kasai, “Water Quality Analysis of Lake Hachiroko, Japan, Using ALOS PALSAR Data,” Int. J. of the Society of Materials Engineering for Resources, Vol.20, No.2, pp. 175-180, 2014.
- [7] D. Wang, Y. Kageyama, M. Nishida, H. Shirai, and A. Motozawa, “Water Quality Analysis in Lake Hachiroko, Japan, Using ALOS AVNIR-2 Data,” IEEJ Trans. on Electrical and Electronic Engineering, Vol.8, No.6, pp. 627-633, 2013.
- [8] D. Wang, Y. Kageyama, M. Nishida, and H. Shirai, “Analysis of Water Quality of Lake Hachiroko, Japan, Using Fuzzy Multiple Regression Model with ALOS AVNIR-2 Data,” J. Adv. Comput. Intell. Intell. Inform., Vol.20, No.6, pp. 992-1003, 2016.
- [9] Y. Kageyama, A. Izumi, M. Nishida, and H. Yokoyama, “Application of Fuzzy C-means for Understanding Water Quality in Lake Hachiroko, Japan,” IEEJ Trans. on Electrical and Electronic Engineering, Vol.11, No.6, pp. 835-837, 2016.
- [10] http://www.pref.akita.lg.jp/ [accessed November 19, 2017]
- [11] www.env.go.jp/ [accessed November 19, 2017]
- [12] http://landbrowser.geogrid.org/landbrowser/ [accessed November 19, 2017]
- [13] https://asterweb.jpl.nasa.gov/ [accessed November 19, 2017]
- [14] https://landsat.usgs.gov/ [accessed November 19, 2017]
- [15] http://www.jma.go.jp/ [accessed November 20, 2017]
- [16] M. Takagi and H. Shimoda, “Handbook of Image Analysis [Revised Edition],” University of Tokyo Press, 2004.
- [17] H. Ishibuchi, “Fuzzy regression analysis,” J. of Japan Society for Fuzzy Theory and Systems, Vol.4, pp. 52-60, 1992.
- [18] M. Mizumoto, “Fuzzy reasoning (1),” J. of Japan Society for Fuzzy Theory and Systems, Vol.4, pp. 256-264, 1992.
This article is published under a Creative Commons Attribution-NoDerivatives 4.0 Internationa License.