Short Paper:
Research on Multiband Packet Fusion Algorithm for Hyperspectral Remote Sensing Images
Cai Zhao
Xi’an Jiaotong University, City College
Xi’an 710018, China
The data recorded by current algorithms contains more errors, which reduces the quality of hyperspectral remote sensing images and affects the fusion results. A fusion algorithm based on improved IHS transform is proposed. In order to avoid the noise and diffusion spread and the uniform distribution of gray level, the detail information is preserved and the image is geometric corrected, denoised and histogram equalized. Then the feature extraction, edge detection and feature matching are performed to the images. The weighted average fusion criterion is used to improve the fusion algorithm of IHS transform to improve the spectral distortion of fusion images. Through statistical and visual interpretation of evaluation results, the proposed fusion algorithm preserves the original spectral information and has good visual effects, which is more in line with human subjective evaluation criteria.
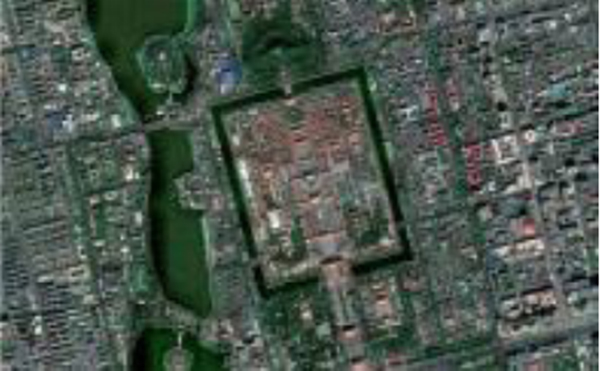
Fusion results of the algorithm after optimization
- [1] J. Xu, X. Yu, W. Pei, D. Hu, and L. Zhang, “A remote sensing image fusion method based on feedback sparse component analysis,” Computers & Geosciences, Vol.85, Part B, pp. 115-123, 2015.
- [2] D. Guo, J. Yan, and X. Qu, “High quality multi-focus image fusion using self-similarity and depth information,” Optics Communications, Vol.338, pp. 138-144, 2015.
- [3] D. D. Alexakis, A. Sarris, C. Kalaitzidis, et al., “Integrated use of satellite remote sensing, gis, and ground spectroscopy techniques for monitoring olive oil mill waste disposal areas on the island of crete, greece,” Int. J. of Remote Sensing, Vol.37, No.3, pp. 669-693, 2016.
- [4] A. A. Bloom, J. Worden, Z. Jiang, et al., “Remote-sensing constraints on south america fire traits by bayesian fusion of atmospheric and surface data,” Geophysical Research Letters, Vol.42, No.4, pp. 1268-1274, 2015.
- [5] Q. Wang, M. Wei, and Y. Zhu, “Compressive Sensing of Noisy 3-D Images Based on Threshold Selection,” J. Adv. Comput. Intell. Intell. Inform., Vol.22, No.1, pp. 70-75, 2018.
- [6] G. Yang, Y. Zhang, J. Yang, et al., “Automated classification of brain images using wavelet-energy and biogeography-based optimization,” Multimedia Tools & Applications, Vol.75, No.23, pp. 15601-15617, 2016.
This article is published under a Creative Commons Attribution-NoDerivatives 4.0 Internationa License.