Short Paper:
Rapid Feature Retrieval Method in Large-Scale Image Database
Fei Gao
School of Information Science and Technology, Tibet University
36 Jiangsu Road, Chengguan Qu, Lasa Shi, Xizang Zizhiqu, Lhasa, Tibet 850000, China
The retrieval of features in a large-scale image database can improve the degree of visualization of images. The conventional method of feature-retrieval is a time-consuming process because it retrieves by searching the keywords. In this paper, a rapid feature retrieval method based on granular computing is proposed for use in a large-scale image database. In this method, we first collect and process the images from the database. Next, we construct a binary tree to realize the multi-class classification of the image features and complete the feature retrieval using support vector machines. The experimental results demonstrate that the proposed method can effectively retrieve the features in the large-scale image database. The effectiveness of retrieval can reach more than 95%.
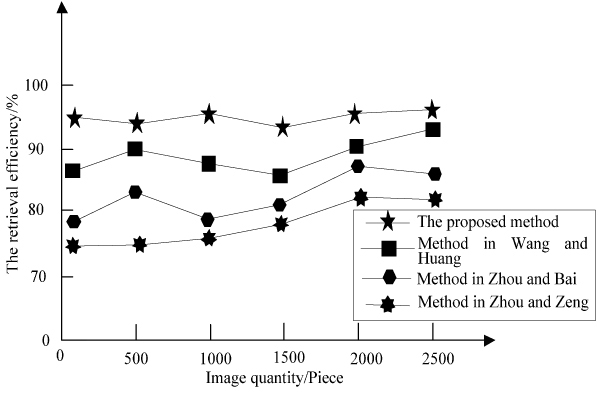
The advantages of the proposed method are verified by comparison
- [1] X. Yu, P. Xi, and J. Huang, “Design and Implementation of Distributed Retrieval in Video Monitoring System,” Computer Measurement & Control, Vol.23, No.7, pp. 2511-2514, 2015.
- [2] J. Chang, X. Guan, H. Wang et al., “Radar Emitter Recognition Based on Feature Vector with Hybrid Data Types,” Computer Simulation, Vol.33, No.9, pp. 1-4, 2016.
- [3] M. Liu, “Integrated Scheduling of Virtual Information Resources in Data Characteristics of Cloud Computing,” Bulletin of Science and Technology, Vol.31, No.10, pp. 199-201, 2015.
- [4] M. Zhou and Z. Bai, “Image retrieval and classification based on shape feature,” Foreign Electronic Measurement Technology, Vol.6, pp. 82-84, 2015.
- [5] L. Wang and S. Huang, “Image Retrieval algorithm based on Multi-scale convolution Neural Network,” Software Guide, Vol.15, No.2, pp. 38-40, 2016.
- [6] Y. Zhou and F. Zeng, “An Image Retrieval Algorithm Based on Two-Dimensional Compressive Sensing and Hierarchical Feature,” J. of Electronics, Vol.44, No.2, pp. 453-460, 2016.
- [7] T. Hashimoto, Y. Munakata, R. Yamanaka, and A. Kurosu, “Proposal of Episodic Memory Retrieval Method on Mood Congruence Effects,” J. Adv. Comput. Intell. Intell. Inform., Vol.21, No.4, pp. 722-729, 2017.
This article is published under a Creative Commons Attribution-NoDerivatives 4.0 Internationa License.